连续动作空间深度Actor-Critic算法研究
项目介绍
AI项目解读
基本信息
- 批准号:61762032
- 项目类别:地区科学基金项目
- 资助金额:36.0万
- 负责人:
- 依托单位:
- 学科分类:F06.人工智能
- 结题年份:2021
- 批准年份:2017
- 项目状态:已结题
- 起止时间:2018-01-01 至2021-12-31
- 项目参与者:欧宜贵; 周辉; 王冬梅; 林志阳; 陈亚君; 王中正;
- 关键词:
项目摘要
By integrating the advantages of the perception of deep learning and the decision making of reinforcement learning, deep reinforcement learning provides a new way for high-dimensional sequential decision-making problems. However, because the research time is so short, deep reinforcement learning is facing many difficulties, such as the sample decorrelation, the convergence guarantee, the computational cost, the learning efficiency, etc. Moreover, most of the proposed algorithms are designed for the sequential decision-making problems in discrete action spaces, and they are difficult to meet the practical engineering application. To overcome these difficulties, this project will investigate deep actor-critic algorithms in depth. Firstly, we will propose some novel random sampling mechanisms to remove the correlation among sequential samples, and rebuild the computational models of value function, advantage function and policy gradient. Secondly, we will analyze the asymptotic convergence factors of deep neural networks, and present the convergent conditions and the proof method about deep actor-critic algorithms. Thirdly, we will optimize and reconstruct the network architecture of deep actor-critic algorithms for reducing the computation cost. Lastly, we will design some adaptive vector-valued step-sizes for improving the learning efficiency of deep actor-critic algorithms. The research results of this project will not only enrich and deepen the theory of deep reinforcement learning, but also improve its engineering practicability.
通过将深度学习的感知能力和强化学习的决策能力有机结合,深度强化学习为求解高维序贯决策问题提供了新的思路。然而由于研究时间不长,当前深度强化学习在样本去相关性、收敛保证、计算开销和学习效率等方面还存在许多问题,且所提算法大多面向离散动作空间序贯决策问题,难以满足实际工程需要。为此,本项目主要围绕连续动作空间深度Actor-Critic算法展开研究。首先,建立可自动消解序贯采样相关性的随机取样新机制,重构值函数、优势函数和策略梯度计算模型。其次,对深度神经网络的渐近收敛影响因素进行分析,给出深度Actor-Critic算法的收敛条件和证明方法。再次,对深度Actor-Critic算法的网络模型结构进行优化改造,减小计算开销。最后,设计适于深度Actor-Critic算法的自适应向量值步长,提高学习效率。所获成果不但可以丰富和深化现有深度强化学习理论,而且可以有效提高其工程实用性。
结项摘要
与大多数深度强化学习算法相似,连续动作空间深度Actor-Critic(DAC)算法也容易遭受样本相关性、收敛性难保证、学习效率低等问题。为此,本项目从三个层面展开研究:首先,对DAC的底层算法进行研究,分别提出了适于五种常用神经网络的迷你批RLS优化算法集、面向大规模非受限优化的非单调缩放共轭梯度算法、0-1二次规划的神经动力方法、优化加权核范数模型方法、基于RLS优化的CNN裁剪算法。其中,我们的迷你批RLS优化算法可视作一类特殊的SGD算法,其综合运用了我们平均近似、等效梯度和经典的误差反向传播技术,其时间和空间复杂度和SGD同阶,但是却具有更好的收敛性能。其次,采用经验回放、迷你批学习方式或门限机制等消减样本相关性,提出了一系列基于RLS优化ESN、FNN、CNN和RNN的深度Q学习和Sarsa算法。最后,基于RLS-ESN和RLS-RNN, 我们提出了采用标准策略梯度的优势Actor-Critic(A2C)算法,其迷你批样本从经验回放集抽取。基于RLS-CNN,我们进一步提出了采用标准和自然策略的梯度A2C算法(即RLSSA2C和RLSNA2C),其迷你批样本由多个并行Workers与环境交互产生。实验结果表明,RLSSA2C和RLSNA2C不仅具有较标准深度A2C算法更好的学习效率,而且具有较当前主流深度A2C算法更高的计算效率。
项目成果
期刊论文数量(6)
专著数量(0)
科研奖励数量(0)
会议论文数量(2)
专利数量(0)
A neurodynamic approach to zero-one quadratic programming
零一二次规划的神经动力学方法
- DOI:10.1007/s11075-021-01075-z
- 发表时间:2021-02
- 期刊:Numerical Algorithms
- 影响因子:2.1
- 作者:Ou Yigui;Lin Haichan
- 通讯作者:Lin Haichan
Exponential stability of the continuous method model for solving general variational inequality problems
求解一般变分不等式问题的连续法模型的指数稳定性
- DOI:10.2306/scienceasia1513-1874.2020.085
- 发表时间:2020
- 期刊:ScienceAsia
- 影响因子:1.2
- 作者:Wenjie Xu;Yigui Ou
- 通讯作者:Yigui Ou
递归最小二乘循环神经网络
- DOI:10.16383/j.aas.c190847
- 发表时间:--
- 期刊:自动化学报
- 影响因子:--
- 作者:赵杰;张春元;刘超;周辉;欧宜贵;宋淇
- 通讯作者:宋淇
A nonmonotone scaled conjugate gradient algorithm for large-scale unconstrained optimization
用于大规模无约束优化的非单调共轭梯度算法
- DOI:10.1080/00207160.2017.1368498
- 发表时间:2018-11
- 期刊:International Journal of Computer Mathematics
- 影响因子:1.8
- 作者:Ou Yigui;Zhou Xin
- 通讯作者:Zhou Xin
Estimation of the parameters of a weighted nuclear norm model and its application in image denoising
加权核范数模型参数估计及其在图像去噪中的应用
- DOI:10.1016/j.ins.2020.04.028
- 发表时间:2020-08-01
- 期刊:INFORMATION SCIENCES
- 影响因子:8.1
- 作者:Deng, Hongyao;Tao, Jinsong;Zhang, Chunyuan
- 通讯作者:Zhang, Chunyuan
数据更新时间:{{ journalArticles.updateTime }}
{{
item.title }}
{{ item.translation_title }}
- DOI:{{ item.doi || "--"}}
- 发表时间:{{ item.publish_year || "--" }}
- 期刊:{{ item.journal_name }}
- 影响因子:{{ item.factor || "--"}}
- 作者:{{ item.authors }}
- 通讯作者:{{ item.author }}
数据更新时间:{{ journalArticles.updateTime }}
{{ item.title }}
- 作者:{{ item.authors }}
数据更新时间:{{ monograph.updateTime }}
{{ item.title }}
- 作者:{{ item.authors }}
数据更新时间:{{ sciAawards.updateTime }}
{{ item.title }}
- 作者:{{ item.authors }}
数据更新时间:{{ conferencePapers.updateTime }}
{{ item.title }}
- 作者:{{ item.authors }}
数据更新时间:{{ patent.updateTime }}
其他文献
一种流体系结构中软硬结合的异构核协同方法
- DOI:--
- 发表时间:--
- 期刊:计算机学报
- 影响因子:--
- 作者:任巨;何义;张春元;伍楠;文梅;荀长庆;杨乾明
- 通讯作者:杨乾明
新工科背景下的软件工程课程实践教学建设:思考与探索
- DOI:10.16512/j.cnki.jsjjy.2018.07.002
- 发表时间:2018
- 期刊:计算机教育
- 影响因子:--
- 作者:毛新军;尹刚;尹良泽;张春元;毛晓光
- 通讯作者:毛晓光
一种减小OFDM 系统PAPR 的算法分析
- DOI:--
- 发表时间:2014
- 期刊:广西大学学报(自然科学版)
- 影响因子:--
- 作者:林志阳;王磊;王兆晖;张春元;周又玲;丁洁;张莉
- 通讯作者:张莉
动态故障树的不交化定量分析方法
- DOI:--
- 发表时间:--
- 期刊:计算机研究与发展
- 影响因子:--
- 作者:张红林;张春元;刘东;付剑;Zhang Honglin1,Zhang Chunyuan1,Liu Dong2,;Fu Ji
- 通讯作者:Fu Ji
适用于SIMD体系结构的FPGA分页仿真模型研究
- DOI:--
- 发表时间:--
- 期刊:计算机研究与发展
- 影响因子:--
- 作者:文梅;杨乾明;何义;伍楠;张春元;任巨
- 通讯作者:任巨
其他文献
{{
item.title }}
{{ item.translation_title }}
- DOI:{{ item.doi || "--" }}
- 发表时间:{{ item.publish_year || "--"}}
- 期刊:{{ item.journal_name }}
- 影响因子:{{ item.factor || "--" }}
- 作者:{{ item.authors }}
- 通讯作者:{{ item.author }}
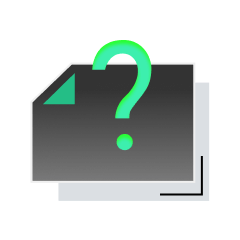
内容获取失败,请点击重试
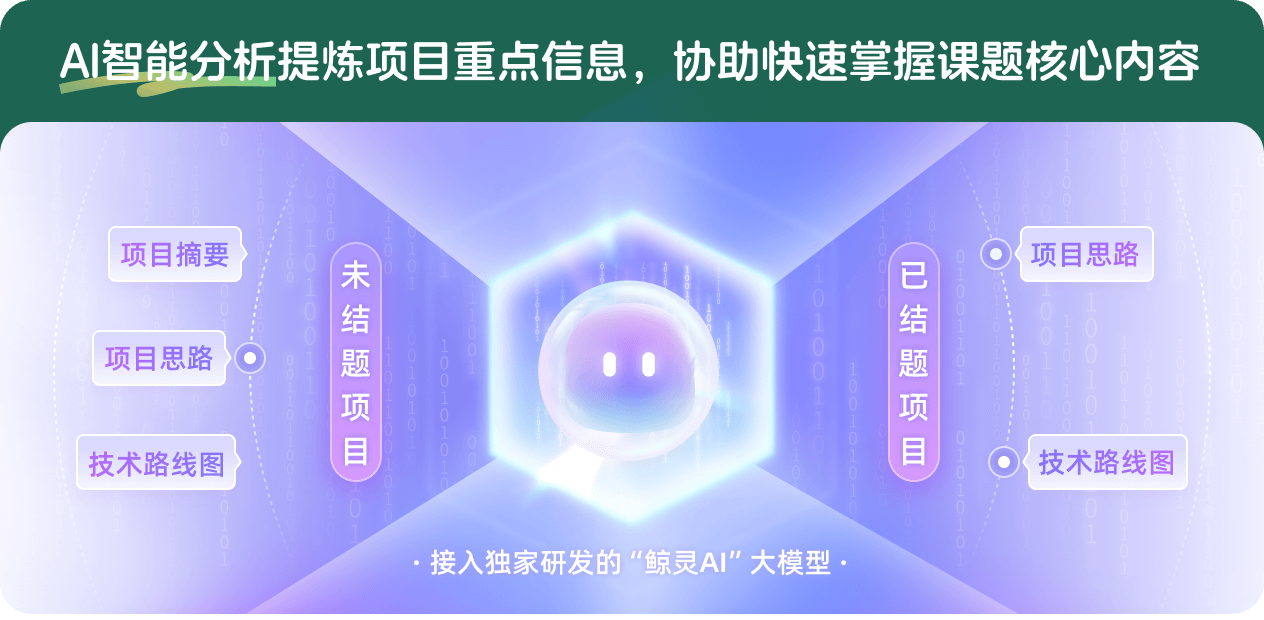
查看分析示例
此项目为已结题,我已根据课题信息分析并撰写以下内容,帮您拓宽课题思路:
AI项目摘要
AI项目思路
AI技术路线图
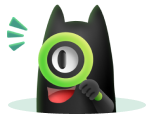
请为本次AI项目解读的内容对您的实用性打分
非常不实用
非常实用
1
2
3
4
5
6
7
8
9
10
您认为此功能如何分析更能满足您的需求,请填写您的反馈:
相似国自然基金
{{ item.name }}
- 批准号:{{ item.ratify_no }}
- 批准年份:{{ item.approval_year }}
- 资助金额:{{ item.support_num }}
- 项目类别:{{ item.project_type }}
相似海外基金
{{
item.name }}
{{ item.translate_name }}
- 批准号:{{ item.ratify_no }}
- 财政年份:{{ item.approval_year }}
- 资助金额:{{ item.support_num }}
- 项目类别:{{ item.project_type }}