基于非独立同分布样本的统计学习理论研究与应用
项目介绍
AI项目解读
基本信息
- 批准号:61473328
- 项目类别:面上项目
- 资助金额:58.0万
- 负责人:
- 依托单位:
- 学科分类:F0605.模式识别与数据挖掘
- 结题年份:2018
- 批准年份:2014
- 项目状态:已结题
- 起止时间:2015-01-01 至2018-12-31
- 项目参与者:刘燕; 范钦伟; 李锋; 范业田; 贾杰; 李文钰;
- 关键词:
项目摘要
The classical results of statistical learning theory are almost built under the assumption that samples are independently drawn from an identical distribution. However, this assumption is not always valid in practice, for example, estimation of information channel,prediction of time serials and functional data analysis.There have been many learning models developed for the non-i.i.d. learning problems, but the relevant theoretical analysis is still in the beginning stages. In this project, we will extend the classical results of the statistical learning theory to the scenario of non-i.i.d. samples. Since the non-i.i.d. scenario contains a wide variety of cases, it is impossible to find a unified form to cover all the cases. Instead,one feasible scheme is to find some representative processes,e.g., near-epoch dependence, L1-mixingale, Markov processes and Gaussian processes, which cover several useful cases in the scenario of non-i.i.d. samples, and then we study the theoretical properties of the learning process for each representative stochastic process individually. For each learning process, we will obtain the corresponding deviation inequalities, symmetrization inequalities and generalization bounds. We then analyze the consistence and the rate of convergence of the learning process. Next, we will study the complexity measures of the function classes evaluated at the real domain and the time interval and analyze the learnability of the existing learning models for the non-i.i.d. scenario. Based on the theoretical findings, we will induce the general characteristics of the non-i.i.d. learning processes and then improve the existing non-i.i.d. models.
经典的统计学习理论结果大多是基于样本独立同分布假设的,然而此假设在很多实际问题中无法满足,例如:信道估计、时间序列预测和泛函数据分析等。尽管已有诸多非独立同分布学习模型被应用到实际问题中,但是对这类学习模型的理论分析还相对薄弱。本项目拟将经典的统计学理论结果推广到非独立同分布学习问题中去。考虑到非独立同分布学习过程的复杂性,我们将研究几种基于代表性随机过程的学习过程,其中包括:near-epoch dependence、L1-mixingale、Markov过程和高斯过程等。对于每一种学习过程,我们将得到适用于该学习过程的偏差不等式和对称不等式并求得泛化界,进而分析此学习过程的一致性和收敛率。我们还将研究由时间泛函构成的函数类复杂度的性质以及非独立同分布学习模型的可学习性等。并以得到的理论结果为基础,我们将归纳出非独立同分布学习问题的共性并对已有的算法模型进行改进。
结项摘要
经典的统计学习理论结果主要是建立在样本独立同分布假设之上的,然而独立同分布假设在很多实际问题中是无法被满足的,例如:信道估计、时间序列预测和泛函数据分析等。尽管许多已经有很多模型被用于解决非独立同分布问题,但是其相应的理论分析还处于亟待解决的阶段。在本项目的资助之下,我们对基于非独立同分布样本的机器学习算法的泛化性进行了研究,给出了具有广泛意义的非独立同分布样本学习问题的泛化界。此外,我们还对多任务学习和临近风险最小化原则的泛化性进行了研究。在应用方面,我们结合RNN模型对时间序列进行分析,对盾构机的关键运行数据进行了准确的实时预测。随机矩阵理论是目前新的研究热点,其已经被广泛的应用于机器学习、量子计算等领域。但是现有的随机矩阵结果大多是与矩阵的维数相关的,因此这些结果并不适用于高维或是无穷维矩阵情形。在本项目的资助之下,我们还给出了与矩阵维数无关的的随机矩阵集中不等式,这是对先有随机矩阵结果的重要补充。此外我们还对随机矩阵理论在优化问题上的应用进行了研究,并给出了新的Bennett不等式的近似形式。
项目成果
期刊论文数量(5)
专著数量(0)
科研奖励数量(0)
会议论文数量(0)
专利数量(0)
LSV-Based Tail Inequalities for Sums of Random Matrices
基于 LSV 的随机矩阵和的尾部不等式
- DOI:10.1162/neco_a_00901
- 发表时间:2017
- 期刊:Neural Computation
- 影响因子:2.9
- 作者:Chao Zhang;杜磊;Dacheng Tao
- 通讯作者:Dacheng Tao
Drug-symptom networking: Linking drug-likeness screening to drug discovery.
药物症状网络:将药物相似性筛选与药物发现联系起来
- DOI:10.1016/j.phrs.2015.11.015
- 发表时间:2015
- 期刊:Pharmacological Research
- 影响因子:9.3
- 作者:Xu Xue;Zhang Chao;Li PiDong;Zhang FeiLong;Gao Kuo;Chen JianXin;Shang HongCai
- 通讯作者:Shang HongCai
Dynamic load prediction of tunnel boring machine (TBM) based on heterogeneous in-situ data
基于异构现场数据的隧道掘进机(TBM)动载荷预测
- DOI:10.1016/j.autcon.2018.03.030
- 发表时间:2018-08-01
- 期刊:AUTOMATION IN CONSTRUCTION
- 影响因子:10.3
- 作者:Sun, Wei;Shi, Maolin;Song, Xueguan
- 通讯作者:Song, Xueguan
Relaxed conditions for convergence analysis of online back-propagation algorithm with L2 regularizer for Sigma-Pi-Sigma neural network
Sigma-Pi-Sigma 神经网络 L-2 正则化在线反向传播算法收敛分析的宽松条件
- DOI:10.1016/j.neucom.2017.06.057
- 发表时间:2018-01-10
- 期刊:NEUROCOMPUTING
- 影响因子:6
- 作者:Liu, Yan;Yang, Dakun;Zhang, Chao
- 通讯作者:Zhang, Chao
数据更新时间:{{ journalArticles.updateTime }}
{{
item.title }}
{{ item.translation_title }}
- DOI:{{ item.doi || "--"}}
- 发表时间:{{ item.publish_year || "--" }}
- 期刊:{{ item.journal_name }}
- 影响因子:{{ item.factor || "--"}}
- 作者:{{ item.authors }}
- 通讯作者:{{ item.author }}
数据更新时间:{{ journalArticles.updateTime }}
{{ item.title }}
- 作者:{{ item.authors }}
数据更新时间:{{ monograph.updateTime }}
{{ item.title }}
- 作者:{{ item.authors }}
数据更新时间:{{ sciAawards.updateTime }}
{{ item.title }}
- 作者:{{ item.authors }}
数据更新时间:{{ conferencePapers.updateTime }}
{{ item.title }}
- 作者:{{ item.authors }}
数据更新时间:{{ patent.updateTime }}
其他文献
低透气性煤层钻射一体化瓦斯抽采技术研究与应用
- DOI:10.13637/j.issn.1009-6094.2016.04.033
- 发表时间:2016
- 期刊:安全与环境学报
- 影响因子:--
- 作者:张超;李树刚;林海飞;张静非;杨会军
- 通讯作者:杨会军
A denoising framework for microseismic and reflection seismic data based on block matching
基于块匹配的微震和反射地震数据去噪框架
- DOI:10.1190/geo2017-0782.1
- 发表时间:2018-09
- 期刊:GEOPHYSICS
- 影响因子:3.3
- 作者:张超;Mirko van der Baan
- 通讯作者:Mirko van der Baan
国产高强钢高温动态弹性模量试验研究
- DOI:10.13206/j.gjg201704023
- 发表时间:2017
- 期刊:钢结构
- 影响因子:--
- 作者:李国强;黄雷;张超
- 通讯作者:张超
青稞HD-ZipⅣ 5基因克隆及表达探究
- DOI:10.13271/j.mpb.017.002769
- 发表时间:2019
- 期刊:分子植物育种
- 影响因子:--
- 作者:李媛;多杰措;张超;熊辉岩;段瑞君
- 通讯作者:段瑞君
水稻OsUF的基序分析及其亚细胞定位
- DOI:10.3969/j.issn.1674-7968.2019.02.004
- 发表时间:2019
- 期刊:农业生物技术学报
- 影响因子:--
- 作者:丁作美;王媛媛;石超南;王红;张成龙;郑晓媛;张超;吴祖建;吴建国
- 通讯作者:吴建国
其他文献
{{
item.title }}
{{ item.translation_title }}
- DOI:{{ item.doi || "--" }}
- 发表时间:{{ item.publish_year || "--"}}
- 期刊:{{ item.journal_name }}
- 影响因子:{{ item.factor || "--" }}
- 作者:{{ item.authors }}
- 通讯作者:{{ item.author }}
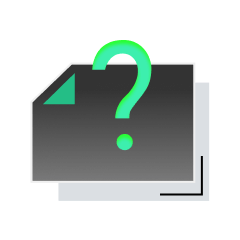
内容获取失败,请点击重试
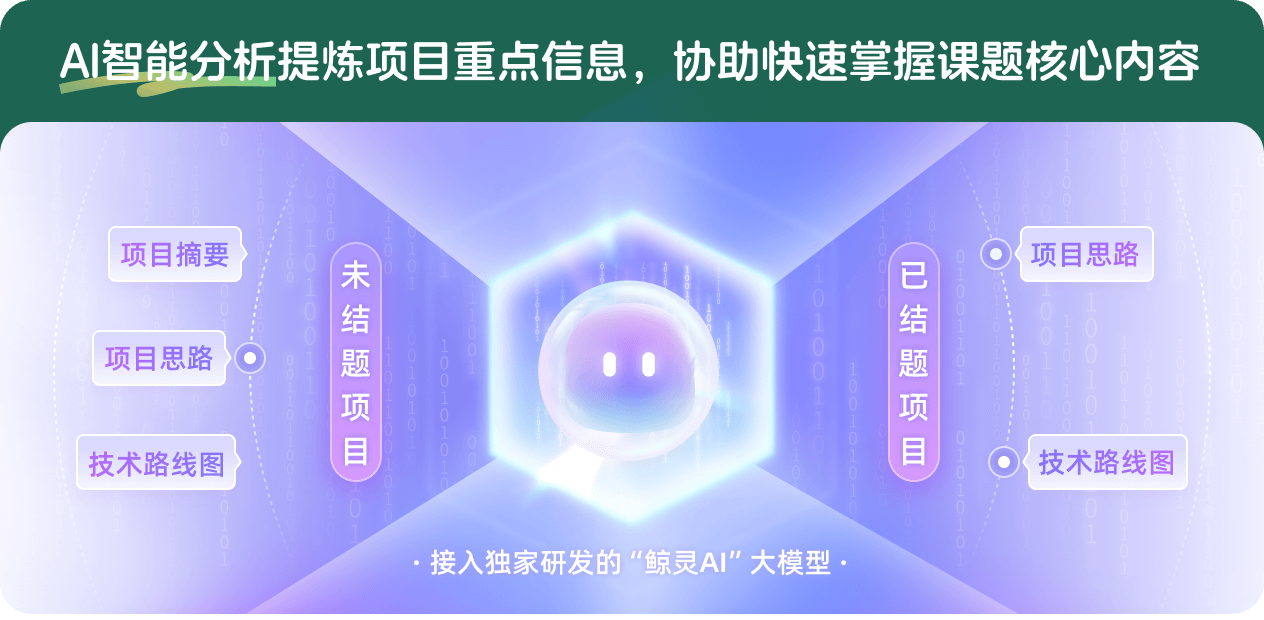
查看分析示例
此项目为已结题,我已根据课题信息分析并撰写以下内容,帮您拓宽课题思路:
AI项目摘要
AI项目思路
AI技术路线图
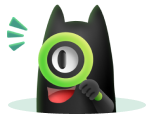
请为本次AI项目解读的内容对您的实用性打分
非常不实用
非常实用
1
2
3
4
5
6
7
8
9
10
您认为此功能如何分析更能满足您的需求,请填写您的反馈:
张超的其他基金
面向深度神经网络的统计学习理论研究
- 批准号:
- 批准年份:2021
- 资助金额:57 万元
- 项目类别:面上项目
多任务学习的理论分析与应用
- 批准号:11401076
- 批准年份:2014
- 资助金额:22.0 万元
- 项目类别:青年科学基金项目
相似国自然基金
{{ item.name }}
- 批准号:{{ item.ratify_no }}
- 批准年份:{{ item.approval_year }}
- 资助金额:{{ item.support_num }}
- 项目类别:{{ item.project_type }}
相似海外基金
{{
item.name }}
{{ item.translate_name }}
- 批准号:{{ item.ratify_no }}
- 财政年份:{{ item.approval_year }}
- 资助金额:{{ item.support_num }}
- 项目类别:{{ item.project_type }}