基于无监督学习的单分子电导数据挖掘
项目介绍
AI项目解读
基本信息
- 批准号:61901402
- 项目类别:青年科学基金项目
- 资助金额:19.5万
- 负责人:
- 依托单位:
- 学科分类:F0122.物理电子学
- 结题年份:2022
- 批准年份:2019
- 项目状态:已结题
- 起止时间:2020-01-01 至2022-12-31
- 项目参与者:--
- 关键词:
项目摘要
At present, the commonly used single-molecule conductance measurement techniques are the scanning tunneling microscope break junction technique and the mechanically controllable break junction (MCBJ) technique, which can generate a large of conductance traces in a single experiment. Data analysis approaches are required to extract the most probable and reliable information from these traces. However, to extract reliable information from conductance traces is still a major challenge in single-molecule conductance measurements. Based on our previous studies on the electronic characterization of single molecular junction, we propose an efficient and reliable data processing and characterization for a large number of conductance data based on unsupervised learning. In this project, we will get a lot of conductance traces of many molecules by MCBJ. According to the data characteristics, we constructs about 1000 sets of conductance traces by simulating for the first time. Each set contains a large number of randomly generated conductance traces of different clusters, which are validation data set to validate the accuracy of the adopted unsupervised learning algorithms. Finally, based on the accuracy, the chosen specific algorithm is applied to the actual experimental conductance measurement data, and an effective single-molecule conductance data processing flow is designed to mine more effective and reliable single-molecule conductance information for molecule electronics research.
目前常用的单分子电导测量技术是扫描隧道显微镜裂结技术和机械可控裂结技术,这类技术可以在单次实验中重复获取大量电导曲线数据,对这些数据实现准确分类,充分挖掘出此数据中与单分子电导有关的信息,为制备分子器件提供了依据。但是,针对越来越庞大的数据,如何进行准确、有效、可信的处理,仍是个难题。本项目基于申请人此前的研究基础,提出了基于无监督学习算法,针对大量电导数据,实现高效、可信的数据处理与表征。本项目首先通过机械可控裂结技术获取大量分子的分子结电导的测量数据,依据数据特征,首次构建模拟出大约1000组的电导数据,每组中都含有大量的随机生成的不同类别的电导数据,使用这些数据作为算法的验证集,用于评判无监督学习算法的准确性。最后以此为依据,将特定算法具体应用于实际的实验测量数据,发展出一套行之有效的单分子电导数据的处理流程,挖掘出更多有效、可信的单分子电导信息,为分子电子学的研究提供可靠的数据基础
结项摘要
单分子电子学实验是为了揭示分子的物理和化学性质,为制备分子材料和设备做准备。断裂结技术产生高通量、高灵敏度的电荷传输数据,提供了一个独特的机会,用于研究单分子尺度的物理化学性质,包括分子与电极耦合的变化、分子电极的不同的连接位点,以及电荷传输量子效应,如量子干涉和近藤效应等。从这些大量的数据中提取有意义的且重要的信息,准确的对数据进行聚类和特征提取已成为单分子电学表征发展的一个关键步骤。然而,由于分子结的随机性,从单分子电导率测量中进一步提取信息仍然具有挑战性,并且使用传统的统计方法容易忽略一些的特征,例如,断裂结过程中不同分子结的构象对应的一些电导变化很容易被忽略。.本项目提出了基于无监督学习算法——聚类算法,针对大量的电导-距离曲线数据,实现高效、可信的数据处理与表征。本项目首先依据裂结数据特征,构建模拟出大约1000组的电导-聚类曲线数据,每组中都含有大量的随机生成的不同类别的电导数据,使用这些数据作为算法的验证集,用于评判聚类算法的准确性。最后以模拟数据为依据,发现谱聚类算法最优,Calinski-Harabasz指标判定聚类数最佳,并将此特定的聚类算法应用于实际的实验测量数据,如两步化学反应过程中的产品分析以及碳烷分子结测量中发现了一个容易被忽略但不可忽视的分子结构象。这实验数据也验证了以Calinski-Harabasz指标为准则的谱聚类算法为大量的断裂结数据分析提供了一种很有潜力的算法。本项目也因此发展出了一套行之有效的单分子电导数据的处理流程,挖掘出了更多有效、可信的单分子电导信息,为分子电子学的研究提供可靠的数据基础。在本项目的资助下,项目负责人发表了论文4篇,包括J. Phys. Chem. C(1篇),CCS Chemistry(1篇),Small(1篇),Angew. Chem. Int. Ed.(1篇)并获批一项专利。
项目成果
期刊论文数量(4)
专著数量(0)
科研奖励数量(0)
会议论文数量(0)
专利数量(1)
Structure Identification for Force-Induced Reaction Using Single-Molecule Conductance Measurement
使用单分子电导测量进行力诱导反应的结构识别
- DOI:10.31635/ccschem.022.202202297
- 发表时间:2022
- 期刊:CCS Chemistry
- 影响因子:11.2
- 作者:Jueting Zheng;Wenli Gao;Taige Lu;Lijue Chen;Luchun Lin;Ruiyun Huang;Yongxiang Tang;Gang Dong;Junyang Liu;Yifei Pan;Wengui Weng;Wenjing Hong
- 通讯作者:Wenjing Hong
Spectral Clustering to Analyze the Hidden Events in Single-Molecule Break Junctions
谱聚类分析单分子断裂连接中的隐藏事件
- DOI:10.1021/acs.jpcc.0c11473
- 发表时间:2021-02-08
- 期刊:JOURNAL OF PHYSICAL CHEMISTRY C
- 影响因子:3.7
- 作者:Lin, Luchun;Tang, Chun;Hong, Wenjing
- 通讯作者:Hong, Wenjing
Strain of Supramolecular Interactions in Single‐Stacking Junctions
单堆叠连接中的超分子相互作用应变
- DOI:10.1002/ange.202200191
- 发表时间:2022
- 期刊:Angewandte Chemie
- 影响因子:--
- 作者:Ruihao Li;Yu Zhou;Wenhui Ge;Jueting Zheng;Yixuan Zhu;Jie Bai;Xiaohui Li;Luchun Lin;Huicong Duan;Jia Shi;Yang Yang;Junyang Liu;Zitong Liu;Wenjing Hong
- 通讯作者:Wenjing Hong
数据更新时间:{{ journalArticles.updateTime }}
{{
item.title }}
{{ item.translation_title }}
- DOI:{{ item.doi || "--"}}
- 发表时间:{{ item.publish_year || "--" }}
- 期刊:{{ item.journal_name }}
- 影响因子:{{ item.factor || "--"}}
- 作者:{{ item.authors }}
- 通讯作者:{{ item.author }}
数据更新时间:{{ journalArticles.updateTime }}
{{ item.title }}
- 作者:{{ item.authors }}
数据更新时间:{{ monograph.updateTime }}
{{ item.title }}
- 作者:{{ item.authors }}
数据更新时间:{{ sciAawards.updateTime }}
{{ item.title }}
- 作者:{{ item.authors }}
数据更新时间:{{ conferencePapers.updateTime }}
{{ item.title }}
- 作者:{{ item.authors }}
数据更新时间:{{ patent.updateTime }}
其他文献
其他文献
{{
item.title }}
{{ item.translation_title }}
- DOI:{{ item.doi || "--" }}
- 发表时间:{{ item.publish_year || "--"}}
- 期刊:{{ item.journal_name }}
- 影响因子:{{ item.factor || "--" }}
- 作者:{{ item.authors }}
- 通讯作者:{{ item.author }}
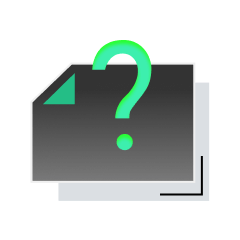
内容获取失败,请点击重试
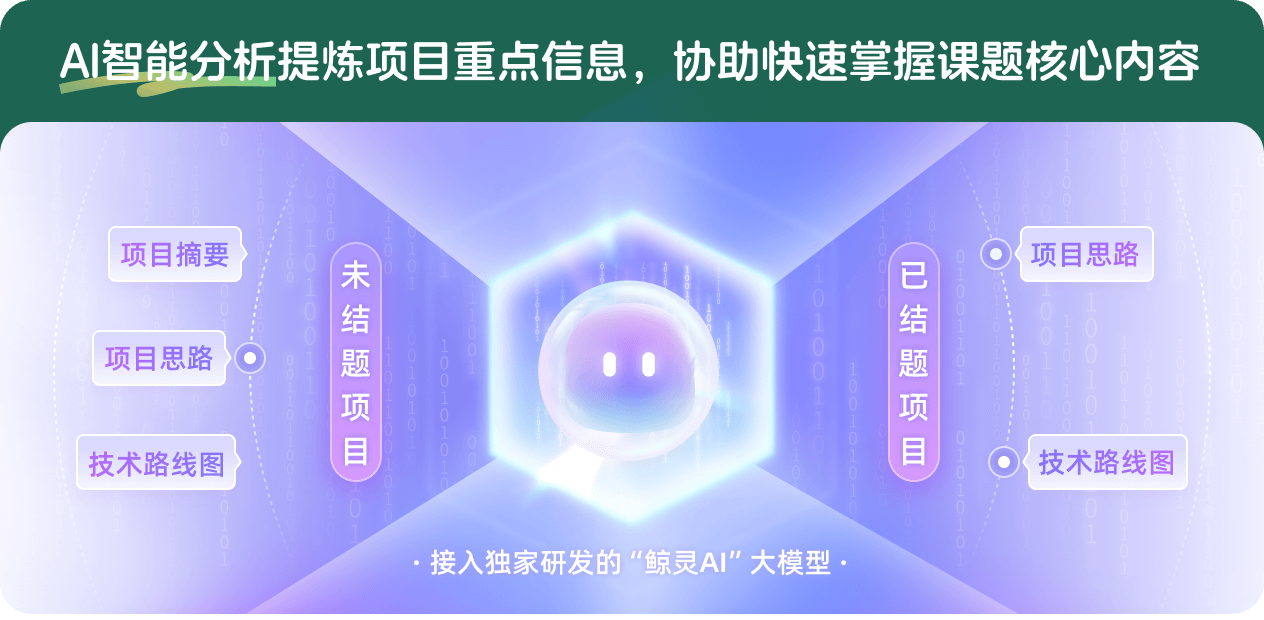
查看分析示例
此项目为已结题,我已根据课题信息分析并撰写以下内容,帮您拓宽课题思路:
AI项目摘要
AI项目思路
AI技术路线图
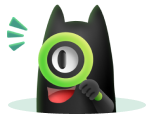
请为本次AI项目解读的内容对您的实用性打分
非常不实用
非常实用
1
2
3
4
5
6
7
8
9
10
您认为此功能如何分析更能满足您的需求,请填写您的反馈:
相似国自然基金
{{ item.name }}
- 批准号:{{ item.ratify_no }}
- 批准年份:{{ item.approval_year }}
- 资助金额:{{ item.support_num }}
- 项目类别:{{ item.project_type }}
相似海外基金
{{
item.name }}
{{ item.translate_name }}
- 批准号:{{ item.ratify_no }}
- 财政年份:{{ item.approval_year }}
- 资助金额:{{ item.support_num }}
- 项目类别:{{ item.project_type }}