混合复发事件数据的统计分析及其他
项目介绍
AI项目解读
基本信息
- 批准号:11371062
- 项目类别:面上项目
- 资助金额:50.0万
- 负责人:
- 依托单位:
- 学科分类:A0402.统计推断与统计计算
- 结题年份:2017
- 批准年份:2013
- 项目状态:已结题
- 起止时间:2014-01-01 至2017-12-31
- 项目参与者:胡涛; 梁宝生; 曲荣华; 谢传龙; 陈文; 杨幸美; 李界;
- 关键词:
项目摘要
Recurrent event data and panel count data often occur in the medical,economic and social study.There are extensive literatures on the statistical inference on these two types of data, respectively. This project focuses on the mixed data that consist of the both types of data, which are defined by three types (called type I,II and III).In the current literature, there is only one our literature that studied the type I mixed data. In this project, we aim to study the three types of mixed data and propose the estimation method for the regression parameter and unknown baseline mean function, simultaneously, under the informative and noninformative censoring scheme. We propose the estimation equation method and maximum likelihood method for the proportional and additive mean model. The theoretical properties based on martingale and empirical process are applied to prove the asymptotical behaviors, including the consistency,convergence rate,normality and efficiency. Also we study the variable selection technique and high dimension reduction method, model checking technique and the hypothesis test on the significance of the treatment w.r.t control group. Finally, we apply our methodology to the CCSS data that study the long-term effect of cancer and PTSD study that study the covariate effect in Wenchuan earthquake, which improves the significance of our study results. In addition, we also explore the cure rate model under interval censored data, which aims to give out the good estimation procedure and effective algorithm.This project will greatly enrich the theory and application of recurrent event data, panel count data and their mixed data.
复发事件数据与面板计数数据在医学、经济学、社会学等领域非常普遍,有大量的文献在分别研究这两类数据的统计推断。本项目是研究这两类数据的混合数据,总共分为3类。在目前的国际文献中,还只有我们1篇文章来研究I型混合数据的估计方法。本项目重点研究3类不同的混合数据,在带有信息和不带有信息删失情况下,回归参数和未知基准均值函数的估计方法。重点研究在比例均值模型和可加均值模型下,基于估计方程和极大似然的估计方法。应用鞅过程和经验过程的理论性质,来证明估计的渐近性质,包括收敛速度和有效性。同时研究高维降维方法和变量选择的方法,以及模型检验、处理组与对照组的均值函数的假设检验问题。我们还将我们的方法用于CCSS数据和汶川大地震的PTSD发病率的研究,这样我们的研究成果具有重大的现实意义。对区间删失之下的治愈率模型,提出我们的估计方法和有效的算法。本项目将大大丰富复发事件和面板计数数据方面的理论和应用。
结项摘要
本项目在复发事件数据的混合型数据, 区间删失数据, 基因多效性等多个领域取得很多重要的成果, 有的还是突破性的成果. 一共发表了35篇文章, 其中SCI文章31篇, 发表在统计界”四大金刚”杂志上文章3篇: JASA 1篇, Biometrika 2篇. 在其他国际顶尖杂志CSDA, Statistica Sinica,Genetics,Genetic Epidemiology, Biostatistics, Scandinavian J. of Statistic 1篇, Statistics 共发表了13篇文章. 其中发表在2016年Genetic杂志的文章,标注为“Highlighted Article”. 同时培养了2名博士毕业,还有2名在读博士。. 本项目的主要成果:1. 提出了混合型的复发事件数据类型,I混合,II型混合数据和“Repeated Current Status Data”。利用零均值过程的性质构造估计方程的方法和极大似然估计的方法,得到回归参数和基准累积函数的大样本性质,丰富了半参数有效性的理论。并将方法应用在CSSC数据和帕金森数据,得到了很有意义的结论。同时也采用了变系数的方法来研究面板计数数据,得到参数的参数的极大偏似然函数估计和极大似然函数估计,得到他们的渐近性质与有效估计。2. 考虑了集群区间删失,二元区间删失,相依区间删失等在Cox模型,AFT模型和可加风险模型下参数的估计方法, 大大地拓宽了该类数据的分析方法. 我们采用了sieve和Copula方法,并证明了这种Sieve极大似然估计的有效性。 模拟数据和实际数据分析证明了该方法的有效性和可行性; 3. 提出了多元基因性状的基因多效性的检验问题。我们以线性模型和广义线性模型来解决这类问题,提出了两步检验方法和K-步序贯检验方法。该方法有效地控制了I型错误率,而且具有很高的功效。模拟结果和实际数据分析都表明了检验方法的优越性。同时提出了多元Microbiome回归核相关检验方法,用于检验多元连续变量与Microbiome结构之间的关系。. 本项目极大地丰富了复发数据与面板计数数据,删失数据在医学中的理论研究与应用。
项目成果
期刊论文数量(35)
专著数量(0)
科研奖励数量(0)
会议论文数量(0)
专利数量(0)
Learning dynamic causal relationships among sugar prices
学习糖价之间的动态因果关系
- DOI:10.1007/s10255-017-0699-5
- 发表时间:2017-08
- 期刊:Acta Mathematicae Applicatae Sinica-English Series
- 影响因子:0.8
- 作者:Xu Jing;Tong Xing-wei;Wang Fang;Chen Jian-ping
- 通讯作者:Chen Jian-ping
Efficient estimation for the additive hazards model in the presence of left-truncation and interval censoring
存在左截断和区间审查的加性危险模型的有效估计
- DOI:10.4310/sii.2015.v8.n3.a12
- 发表时间:2015-01-01
- 期刊:STATISTICS AND ITS INTERFACE
- 影响因子:0.8
- 作者:Wang, Peijie;Tong, Xingwei;Sun, Jianguo
- 通讯作者:Sun, Jianguo
A small-sample multivariate kernel machine test for microbiome association studies
用于微生物组关联研究的小样本多变量核机测试
- DOI:10.1002/gepi.22030
- 发表时间:2017-04-01
- 期刊:GENETIC EPIDEMIOLOGY
- 影响因子:2.1
- 作者:Zhan, Xiang;Tong, Xingwei;Chen, Jun
- 通讯作者:Chen, Jun
Tests for Coefficients in High-dimensional Additive Hazard Models
高维可加危险模型中的系数检验
- DOI:10.1111/sjos.12127
- 发表时间:2015
- 期刊:Scandinavian Journal of Statistics
- 影响因子:1
- 作者:Zhong Ping-Shou;Hu Tao;Li Jun
- 通讯作者:Li Jun
Adjusted empirical likelihood inference for additive hazards regression
加性风险回归的调整经验似然推断
- DOI:10.1080/03610926.2014.978948
- 发表时间:2016-02
- 期刊:Communications in Statistics - Theory and Methods
- 影响因子:--
- 作者:Wang Shanshan;Hu Tao;Cui Hengjian
- 通讯作者:Cui Hengjian
数据更新时间:{{ journalArticles.updateTime }}
{{
item.title }}
{{ item.translation_title }}
- DOI:{{ item.doi || "--"}}
- 发表时间:{{ item.publish_year || "--" }}
- 期刊:{{ item.journal_name }}
- 影响因子:{{ item.factor || "--"}}
- 作者:{{ item.authors }}
- 通讯作者:{{ item.author }}
数据更新时间:{{ journalArticles.updateTime }}
{{ item.title }}
- 作者:{{ item.authors }}
数据更新时间:{{ monograph.updateTime }}
{{ item.title }}
- 作者:{{ item.authors }}
数据更新时间:{{ sciAawards.updateTime }}
{{ item.title }}
- 作者:{{ item.authors }}
数据更新时间:{{ conferencePapers.updateTime }}
{{ item.title }}
- 作者:{{ item.authors }}
数据更新时间:{{ patent.updateTime }}
其他文献
线性EV模型参数的联合损失修正估计
- DOI:10.16360/j.cnki.jbnuns.2019.03.003
- 发表时间:2019
- 期刊:北京师范大学学报. 自然科学版
- 影响因子:--
- 作者:李瞳辉;金蛟;童行伟
- 通讯作者:童行伟
其他文献
{{
item.title }}
{{ item.translation_title }}
- DOI:{{ item.doi || "--" }}
- 发表时间:{{ item.publish_year || "--"}}
- 期刊:{{ item.journal_name }}
- 影响因子:{{ item.factor || "--" }}
- 作者:{{ item.authors }}
- 通讯作者:{{ item.author }}
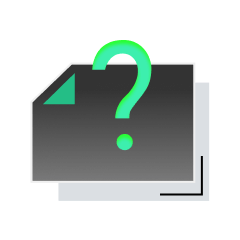
内容获取失败,请点击重试
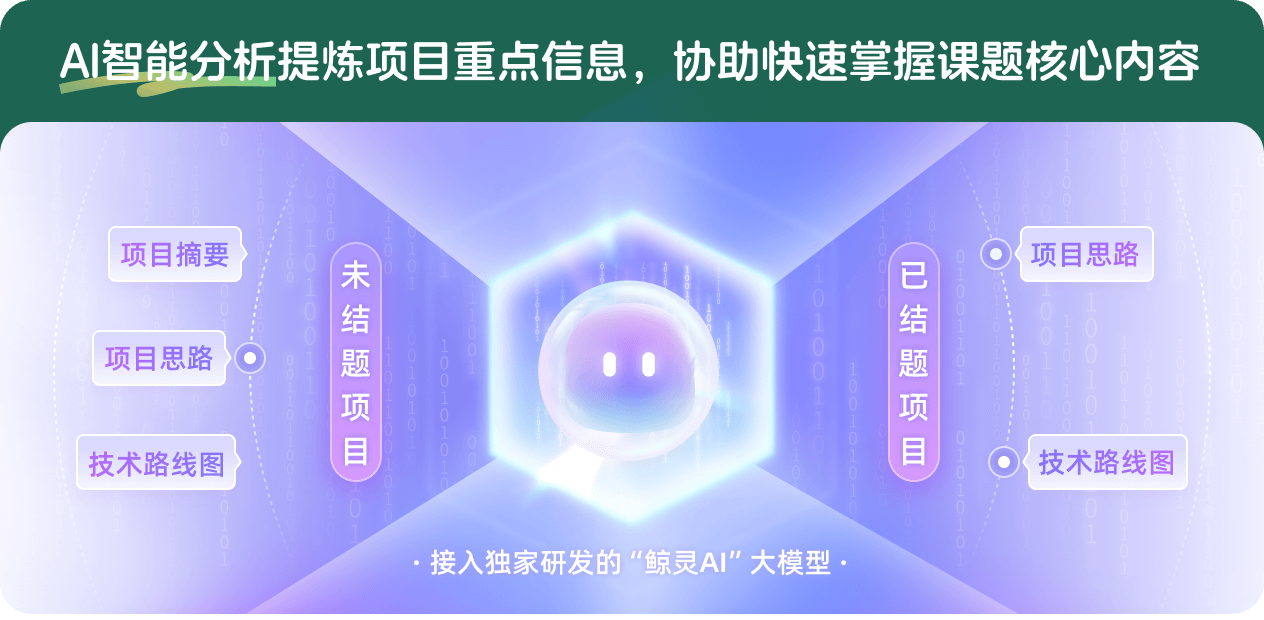
查看分析示例
此项目为已结题,我已根据课题信息分析并撰写以下内容,帮您拓宽课题思路:
AI项目摘要
AI项目思路
AI技术路线图
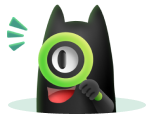
请为本次AI项目解读的内容对您的实用性打分
非常不实用
非常实用
1
2
3
4
5
6
7
8
9
10
您认为此功能如何分析更能满足您的需求,请填写您的反馈:
童行伟的其他基金
生存数据的变点统计推断及其他
- 批准号:12371262
- 批准年份:2023
- 资助金额:43.5 万元
- 项目类别:面上项目
基因多效性的统计推断及其他
- 批准号:
- 批准年份:2019
- 资助金额:52 万元
- 项目类别:面上项目
面板计数数据的统计推断以及应用
- 批准号:10971015
- 批准年份:2009
- 资助金额:24.0 万元
- 项目类别:面上项目
相似国自然基金
{{ item.name }}
- 批准号:{{ item.ratify_no }}
- 批准年份:{{ item.approval_year }}
- 资助金额:{{ item.support_num }}
- 项目类别:{{ item.project_type }}
相似海外基金
{{
item.name }}
{{ item.translate_name }}
- 批准号:{{ item.ratify_no }}
- 财政年份:{{ item.approval_year }}
- 资助金额:{{ item.support_num }}
- 项目类别:{{ item.project_type }}