腹部医学图像分割中可计算变分建模及在肝癌术前评估中的应用
项目介绍
AI项目解读
基本信息
- 批准号:11401231
- 项目类别:青年科学基金项目
- 资助金额:23.0万
- 负责人:
- 依托单位:
- 学科分类:A0604.生物与生命科学中的数学
- 结题年份:2017
- 批准年份:2014
- 项目状态:已结题
- 起止时间:2015-01-01 至2017-12-31
- 项目参与者:王靖; 董芳芳; 张洪博; 王烨; 卢方; 徐猛;
- 关键词:
项目摘要
The segmentation of the liver including tumors and vessels from multi-phase contrast enhanced CT and MR images is a challenging problem in image processing. However, current methods mainly focus on single tissue segmentation from a single phase in a single modality, which generates segmentation results lacking of anatomical meaning. The widely used variational methods endures the bottleneck of segmentation tissues from medical images with complex content, as they are restricted to segment simple images with the assumption of piecewise smooth. This project is to conduct the research of computable variational modeling and fast algorithms based on the combination of the nature of anatomical mechanism and medical data structures, through considering the key problems in the computer assisted intervention of liver cancer as application background. On three key scientific problems of variational models for the segmentation of abdominal images with complex backgrounds, co-segmentation from multi-phase and multi-modality images, and rapid optimization of energy functional with complex constraints, this project is to construct new methods for the segmentation liver, tumor and vessel by solving the key problems in the constructions of segmentation assumption on the complex content of abdominal images, the incorporation of anatomical and local geometrical constraints in variational model, co-segmentation from multi-phase and multi-modality images, and the fast optimization of variational models with complex constraints. Besides, we are to promote the fast application to clinical treatments by establishing a comprehensive validation and evaluation system.
多期增强腹部CT和MR图像中肝脏、肿瘤及血管的分割是当前图像处理中一个具有挑战性的问题。当前的分割方法主要是单模态、单种类图像中的单个器官/组织分割,分割效果往往不佳而且缺乏医学解剖意义。基于变分能量的分割方法在处理内容复杂的医学图像存在理论瓶颈,主要局限于分片光滑等简单图像函数类的分割。本项目以肝癌手术评估规划中的关键问题为应用背景,开展腹部医学图像中基于解剖机理和复杂数据特性的可计算变分建模和快速计算方法的研究。围绕带有复杂背景的腹部图像分割的变分建模方法、多期与多模态腹部图像联合分割方法以及带有复杂约束的变分分割模型的快速优化方法设计三个关键科学问题,突破复杂腹部图像类的建模假设建立、局部几何约束及解剖结构约束模型建立、多期与多模态腹部图像的联合分割、带有复杂约束的变分模型的快速算法设计等关键技术,建立肝脏组织分割的新思路和新方法,并搭建面向快速临床应用的综合验证评价体系。
结项摘要
医学图像中器官或组织的分割是当前图像处理中一个具有挑战性的问题,也是医学图像分析的一个根本性问题,难点在于目标组织的背景负责,边界缺失、含有肿瘤以及多模态数据等。针对这些问题,本项目提出了一系列复杂背景中,特别是针对肝中存在肿瘤的情况下,肝脏的分割方法;这一系列工作中我们还考虑了器官间的结构关系约束、局部几何约束、模型算法设计。具体地,本项目提出了 1) 基于多个区域表征模型的病变肝脏分割方发,成果发表在著名的Medical Physics期刊上;2) 提出了基于深度全卷积神经网络和图割方法的肝组织自动探测和分割方法;3) 提出了基于深度表征学习和全局最优曲面演化的肝脏自动分割方法,成果发表在著名的Physics in Medicine and Biology上;4) 提出了基于深度模型和隐式水平集方法的多器官分割方法;提出了基于测地表征选择和变分能量的病变肝脏分割方法等一些列方法;5)针对多模态数据问题,我们提出了一种新颖的基于结构稀疏化约束的多核学习方法,并给出了非常有效的求解算法,相关成果发表在医学图像计算的顶级会议MICCAI 2016上;6) 针对多数据源的联合学习问题,我们提出了一种多任务学习的方式来有效地利用多模态数据;7)针对研究过程中遇到的图像低分辨问题,我们提出了一种基于结构化低秩约束的图像超分表方法,并给出了一种交替迭代拉格朗日乘子的求解方式。经过充分的数据验证,基于本项目的研究成果和方法具有很好的准确性和鲁棒性,具备较好的临床应用前景。
项目成果
期刊论文数量(11)
专著数量(0)
科研奖励数量(2)
会议论文数量(3)
专利数量(4)
Probability-based method for boosting human action recognition using scene context
使用场景上下文增强人类动作识别的基于概率的方法
- DOI:10.1049/iet-cvi.2015.0420
- 发表时间:2016
- 期刊:IET Computer Vision
- 影响因子:1.7
- 作者:Zhang Hong-Bo;Lei Qing;Chen Duan-Sheng;Zhong Bi-Neng;Peng Jialin;Du Ji-Xiang;Su Song-Zhi
- 通讯作者:Su Song-Zhi
Multi-surface analysis for human action recognition in video.
视频中人体动作识别的多表面分析
- DOI:10.1186/s40064-016-2876-z
- 发表时间:2016
- 期刊:SpringerPlus
- 影响因子:--
- 作者:Zhang HB;Lei Q;Zhong BN;Du JX;Peng J;Hsiao TC;Chen DS
- 通讯作者:Chen DS
Sparse Representation-Based Semi-Supervised Regression for People Counting
基于稀疏表示的半监督回归用于人数统计
- DOI:10.1145/3106156
- 发表时间:2017-08
- 期刊:ACM TRANSACTIONS ON MULTIMEDIA COMPUTING COMMUNICATIONS AND APPLICATIONS
- 影响因子:5.1
- 作者:Zhang Hong-Bo;Zhong Bineng;Lei Qing;Du Ji-Xiang;Peng Jialin;Chen Duansheng;Ke Xiao
- 通讯作者:Ke Xiao
3D liver segmentation using multiple region appearances and graph cuts
使用多区域外观和图形切割进行 3D 肝脏分割
- DOI:10.1118/1.4934834
- 发表时间:2015-12-01
- 期刊:MEDICAL PHYSICS
- 影响因子:3.8
- 作者:Peng, Jialin;Hu, Peijun;Zhang, Hongbo
- 通讯作者:Zhang, Hongbo
Automatic 3D liver segmentation based on deep learning and globally optimized surface evolution
基于深度学习和全局优化表面演化的自动 3D 肝脏分割
- DOI:10.1088/1361-6560/61/24/8676
- 发表时间:2016-12-21
- 期刊:PHYSICS IN MEDICINE AND BIOLOGY
- 影响因子:3.5
- 作者:Hu, Peijun;Wu, Fa;Kong, Dexing
- 通讯作者:Kong, Dexing
数据更新时间:{{ journalArticles.updateTime }}
{{
item.title }}
{{ item.translation_title }}
- DOI:{{ item.doi || "--"}}
- 发表时间:{{ item.publish_year || "--" }}
- 期刊:{{ item.journal_name }}
- 影响因子:{{ item.factor || "--"}}
- 作者:{{ item.authors }}
- 通讯作者:{{ item.author }}
数据更新时间:{{ journalArticles.updateTime }}
{{ item.title }}
- 作者:{{ item.authors }}
数据更新时间:{{ monograph.updateTime }}
{{ item.title }}
- 作者:{{ item.authors }}
数据更新时间:{{ sciAawards.updateTime }}
{{ item.title }}
- 作者:{{ item.authors }}
数据更新时间:{{ conferencePapers.updateTime }}
{{ item.title }}
- 作者:{{ item.authors }}
数据更新时间:{{ patent.updateTime }}
其他文献
城市流动人口生殖健康服务质量评价指标的权重确定方法
- DOI:--
- 发表时间:--
- 期刊:华中科技大学学报(医学版)
- 影响因子:--
- 作者:郭艳平;高峰;刘智勇;彭佳林;李孜;黄锦辉;段建华;高平
- 通讯作者:高平
基于序列间先验约束和多视角信息融合的肝脏CT图像分割
- DOI:--
- 发表时间:2018
- 期刊:电子与信息学报
- 影响因子:--
- 作者:彭佳林;揭萍
- 通讯作者:揭萍
采用共享空间稀疏表示的单幅图像超分辨率方法
- DOI:10.11830/issn.1000-5013.201604051
- 发表时间:2018
- 期刊:华侨大学学报(自然科学版)
- 影响因子:--
- 作者:张建;彭佳林;杜吉祥
- 通讯作者:杜吉祥
Orientation Correction for CT Images via Multitask Deep Convolutional Network
通过多任务深度卷积网络进行 CT 图像方向校正
- DOI:10.11830/issn.1000-5013.201911039
- 发表时间:2020
- 期刊:华侨大学学报(自然科学版)
- 影响因子:--
- 作者:林家庆;韩娟;袁直敏;彭佳林
- 通讯作者:彭佳林
其他文献
{{
item.title }}
{{ item.translation_title }}
- DOI:{{ item.doi || "--" }}
- 发表时间:{{ item.publish_year || "--"}}
- 期刊:{{ item.journal_name }}
- 影响因子:{{ item.factor || "--" }}
- 作者:{{ item.authors }}
- 通讯作者:{{ item.author }}
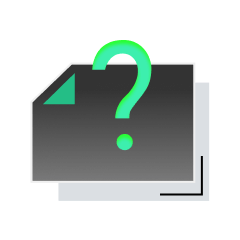
内容获取失败,请点击重试
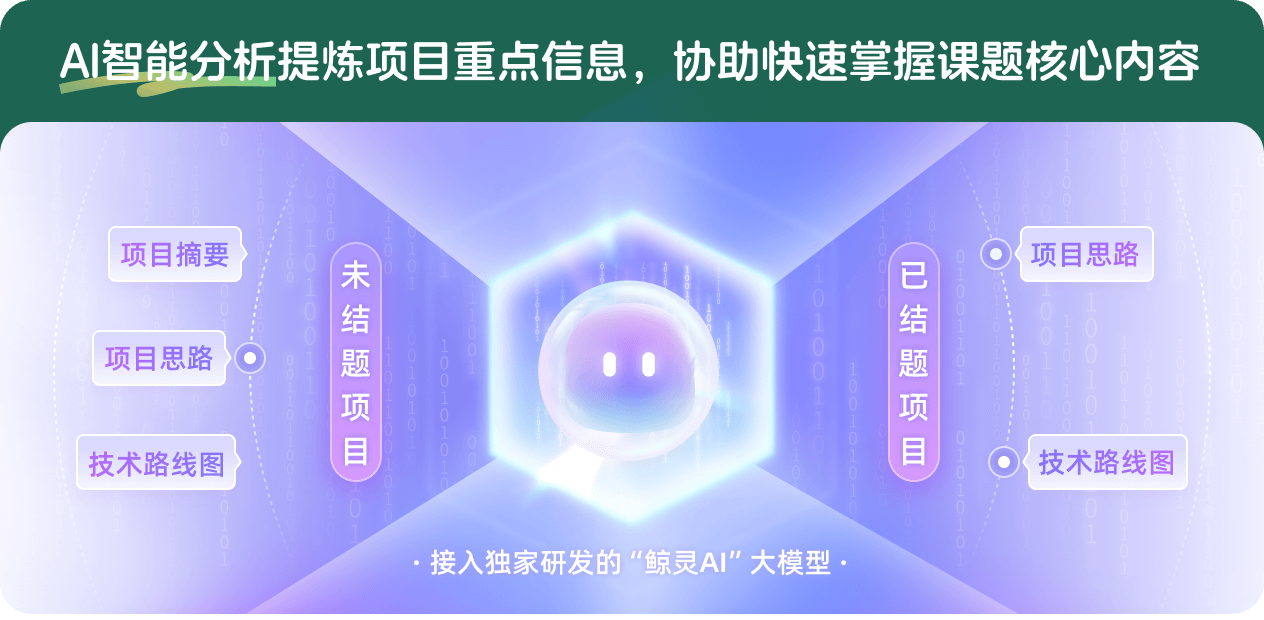
查看分析示例
此项目为已结题,我已根据课题信息分析并撰写以下内容,帮您拓宽课题思路:
AI项目摘要
AI项目思路
AI技术路线图
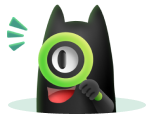
请为本次AI项目解读的内容对您的实用性打分
非常不实用
非常实用
1
2
3
4
5
6
7
8
9
10
您认为此功能如何分析更能满足您的需求,请填写您的反馈:
彭佳林的其他基金
面向肝肿瘤高精度自动语义分割的深度建模及模型跨域迁移算法研究
- 批准号:11771160
- 批准年份:2017
- 资助金额:48.0 万元
- 项目类别:面上项目
相似国自然基金
{{ item.name }}
- 批准号:{{ item.ratify_no }}
- 批准年份:{{ item.approval_year }}
- 资助金额:{{ item.support_num }}
- 项目类别:{{ item.project_type }}
相似海外基金
{{
item.name }}
{{ item.translate_name }}
- 批准号:{{ item.ratify_no }}
- 财政年份:{{ item.approval_year }}
- 资助金额:{{ item.support_num }}
- 项目类别:{{ item.project_type }}