基于部分感知模型的贝叶斯强化学习理论及方法
项目介绍
AI项目解读
基本信息
- 批准号:61772355
- 项目类别:面上项目
- 资助金额:65.0万
- 负责人:
- 依托单位:
- 学科分类:F06.人工智能
- 结题年份:2021
- 批准年份:2017
- 项目状态:已结题
- 起止时间:2018-01-01 至2021-12-31
- 项目参与者:朱斐; 傅启明; 钟珊; 王浩; 钱伟晟; 翟建伟; 章鹏; 徐进; 梁斌;
- 关键词:
项目摘要
Based on fast model learning, this project proposes a method of Bayesian reinforcement learning with partially observable Markov decision processes. This method solves the problems that the environment is partially observable and the knowledge of the model is unknown. The main contents of study are as follows: i. In the discrete state space, we intend to propose a method of Bayesian dynamic programming, based on intelligent model learning. This method may solve the problems that the noise of partially observable models impacts the computation of value functions, such as the convergent speed and accuracy. ii. In partially observable models, it is difficult to predict the unknown states. This leads to the problems that we obtain a suboptimal policy, not the optimal one. To solve this problem, we intend to construct a Bayesian model of dynamic decision network based on discrete state space. iii. The calculation of optimal value functions rely on the model of environment, but the model is partially observable at the beginning. To solve this problem, we intend to present a method to optimize the model of the environment by cross entropy. iv. We intend to propose a method of adaptive Bayesian programming based on Gaussian processes. It can solve the problems of 'curse of dimensionality' and 'curse of history' in the continuous state space, with the partially observable models. v. For the problems with POMDPs, if we want to extend the discrete state space to the continuous one, there are a lot of problems, such as the computational complexity and performance of convergence. We intend to propose a method without discretization. vi. We intend to design a system to realize the aforementioned theory and optimized algorithms, and apply to the problems of robot navigation. Therefore, partially observable model-based Bayesian reinforcement study, has a certain theoretical value and a wide range of application prospects.
本项目在环境部分感知且环境模型未知的情况下,提出基于快速模型学习的贝叶斯强化学习方法。主要内容包括:1. 针对模型部分感知对值函数计算带来的噪声干扰等问题,提出一种基于智能模型学习的贝叶斯动态规划方法。 2. 针对部分感知模型中未知状态难以预测,导致求解最优策略时出现扰动等问题,提出基于离散状态空间来构造动态决策网络的贝叶斯模型。3.针对计算最优值函数依赖环境模型等问题,提出通过交叉熵优化环境模型的方法。4. 针对在部分感知模型下,连续状态空间强化学习出现的“维数灾”和“经验灾”问题,提出基于高斯过程的自适应贝叶斯规划方法。5.针对离散状态的部分马氏问题扩展到连续状态空间时,出现的计算复杂等问题,提出一种在连续状态空间中采取非离散化解决问题的方法。6. 将理论应用于智能机器人导航等问题。因此基于部分感知模型的贝叶斯强化学习研究,既具有一定的理论价值,又具有广泛的应用前景。
结项摘要
本项目在环境部分感知且环境模型未知的情况下,提出基于快速模型学习的贝叶斯强化学习方法。主要内容包括:(1) 针对模型部分感知对值函数计算带来的噪声干扰等问题,提出一种基于智能模型学习的贝叶斯动态规划方法。 (2) 针对部分感知模型中难以预测未知状态,导致求解最优策略时出现扰动等问题,提出基于离散状态空间构造动态决策网络的贝叶斯模型。(3) 针对计算最优值函数依赖环境模型,提出通过交叉熵优化环境模型的方法。(4) 针对在部分感知模型下,连续状态空间强化学习出现的“维数灾”和“经验灾”问题,提出基于高斯过程的自适应贝叶斯规划方法。(5) 针对离散状态的部分马氏问题扩展到连续状态空间时,出现的计算复杂等问题,提出一种在连续状态空间中采取非离散化解决问题的方法。(6) 将理论应用于智能机器人导航等问题。因此基于部分感知模型的贝叶斯强化学习研究,既具有一定的理论价值,又具有广泛的应用前景。
项目成果
期刊论文数量(16)
专著数量(0)
科研奖励数量(0)
会议论文数量(0)
专利数量(4)
Safe Q-Learning Method Based on Constrained Markov Decision Processes
基于约束马尔可夫决策过程的安全Q-Learning方法
- DOI:10.1109/access.2019.2952651
- 发表时间:2019
- 期刊:IEEE Access
- 影响因子:3.9
- 作者:YangYang Ge;Fei Zhu;XingHong Ling;Quan Liu
- 通讯作者:Quan Liu
ARAIL: Learning to rank from incomplete demonstrations
ARAIL:从不完整的演示中学习排名
- DOI:10.1016/j.ins.2021.02.001
- 发表时间:2021-07
- 期刊:Information Sciences
- 影响因子:8.1
- 作者:DaYou Xu;Fei Zhu;Quan Liu;PeiYao Zhao
- 通讯作者:PeiYao Zhao
带最大熵修正的行动者评论家算法
- DOI:--
- 发表时间:2020
- 期刊:计算机学报
- 影响因子:--
- 作者:姜玉斌;刘全;胡智慧
- 通讯作者:胡智慧
一种用于基于方面情感分析的深度分层网络模型
- DOI:--
- 发表时间:2018
- 期刊:计算机学报
- 影响因子:--
- 作者:刘全;梁斌;徐进;周倩
- 通讯作者:周倩
基于重要性采样的优势估计器
- DOI:--
- 发表时间:2019
- 期刊:通信学报
- 影响因子:--
- 作者:刘全;姜玉斌;胡智慧
- 通讯作者:胡智慧
数据更新时间:{{ journalArticles.updateTime }}
{{
item.title }}
{{ item.translation_title }}
- DOI:{{ item.doi || "--"}}
- 发表时间:{{ item.publish_year || "--" }}
- 期刊:{{ item.journal_name }}
- 影响因子:{{ item.factor || "--"}}
- 作者:{{ item.authors }}
- 通讯作者:{{ item.author }}
数据更新时间:{{ journalArticles.updateTime }}
{{ item.title }}
- 作者:{{ item.authors }}
数据更新时间:{{ monograph.updateTime }}
{{ item.title }}
- 作者:{{ item.authors }}
数据更新时间:{{ sciAawards.updateTime }}
{{ item.title }}
- 作者:{{ item.authors }}
数据更新时间:{{ conferencePapers.updateTime }}
{{ item.title }}
- 作者:{{ item.authors }}
数据更新时间:{{ patent.updateTime }}
其他文献
补体调节蛋白与IgA肾病
- DOI:10.3760/cma.j.issn.1674-4756.2013.12.059
- 发表时间:2013-06
- 期刊:中国实用医刊
- 影响因子:--
- 作者:刘全;邢国兰
- 通讯作者:邢国兰
犬贾第虫病毒(长春株)全基因组
- DOI:--
- 发表时间:--
- 期刊:畜牧兽医学报,2006,37(4):408-411
- 影响因子:--
- 作者:陈丽凤;李建华;张西臣*;刘全
- 通讯作者:刘全
双精英协同进化遗传算法
- DOI:--
- 发表时间:2012
- 期刊:软件学报
- 影响因子:--
- 作者:刘全;王晓燕;傅启明;张永刚;章晓芳
- 通讯作者:章晓芳
一种基于资格迹的并行强化学习算法
- DOI:--
- 发表时间:2012
- 期刊:苏州大学学报(自然科学版)
- 影响因子:--
- 作者:杨旭东;刘全;李瑾
- 通讯作者:李瑾
高瓦斯煤层高位钻孔瓦斯抽采技术试验研究
- DOI:--
- 发表时间:2012
- 期刊:煤炭技术
- 影响因子:--
- 作者:赵杰;刘健;王新颖;刘全
- 通讯作者:刘全
其他文献
{{
item.title }}
{{ item.translation_title }}
- DOI:{{ item.doi || "--" }}
- 发表时间:{{ item.publish_year || "--"}}
- 期刊:{{ item.journal_name }}
- 影响因子:{{ item.factor || "--" }}
- 作者:{{ item.authors }}
- 通讯作者:{{ item.author }}
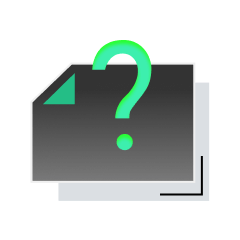
内容获取失败,请点击重试
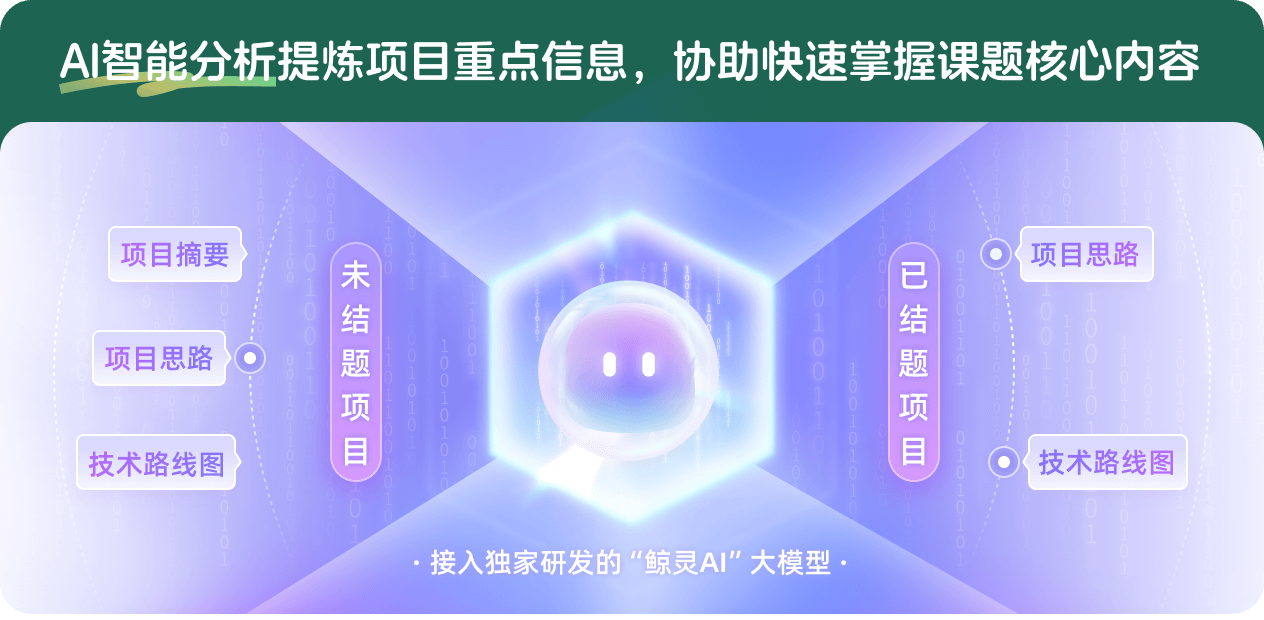
查看分析示例
此项目为已结题,我已根据课题信息分析并撰写以下内容,帮您拓宽课题思路:
AI项目摘要
AI项目思路
AI技术路线图
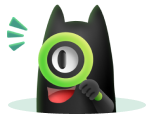
请为本次AI项目解读的内容对您的实用性打分
非常不实用
非常实用
1
2
3
4
5
6
7
8
9
10
您认为此功能如何分析更能满足您的需求,请填写您的反馈:
刘全的其他基金
面向大规模强化学习任务的预测控制理论与方法研究
- 批准号:62376179
- 批准年份:2023
- 资助金额:49 万元
- 项目类别:面上项目
基于模糊逻辑的大规模强化学习理论及方法
- 批准号:61472262
- 批准年份:2014
- 资助金额:82.0 万元
- 项目类别:面上项目
基于贝叶斯推理的模糊逻辑强化学习模型研究
- 批准号:61272005
- 批准年份:2012
- 资助金额:61.0 万元
- 项目类别:面上项目
面向tableau模型的逻辑强化学习理论及方法研究
- 批准号:61070223
- 批准年份:2010
- 资助金额:35.0 万元
- 项目类别:面上项目
近红外波段堆垛结构三维光子晶体的制备和研究
- 批准号:60907017
- 批准年份:2009
- 资助金额:22.0 万元
- 项目类别:青年科学基金项目
基于tableau的非经典逻辑经典化的自动定理证明研究
- 批准号:60873116
- 批准年份:2008
- 资助金额:35.0 万元
- 项目类别:面上项目
相似国自然基金
{{ item.name }}
- 批准号:{{ item.ratify_no }}
- 批准年份:{{ item.approval_year }}
- 资助金额:{{ item.support_num }}
- 项目类别:{{ item.project_type }}
相似海外基金
{{
item.name }}
{{ item.translate_name }}
- 批准号:{{ item.ratify_no }}
- 财政年份:{{ item.approval_year }}
- 资助金额:{{ item.support_num }}
- 项目类别:{{ item.project_type }}