基于机器学习算法的城区建筑能耗不确定性及敏感性研究
项目介绍
AI项目解读
基本信息
- 批准号:51778416
- 项目类别:面上项目
- 资助金额:51.0万
- 负责人:
- 依托单位:
- 学科分类:E0803.建筑物理
- 结题年份:2021
- 批准年份:2017
- 项目状态:已结题
- 起止时间:2018-01-01 至2021-12-31
- 项目参与者:尹宝泉; 许英淑; 宋继田; 孟庆新; 杨松; 刘云亮; 孟献昊; 傅兴;
- 关键词:
项目摘要
Reliable and accurate building energy prediction is the key foundation of energy system planning, system configuration, operating economy and carbon emission calculation for community buildings. The uncertainty of variables in energy systems leads to the difficulty of estimating energy use for community buildings. Therefore, this project will implement forward/inverse uncertainty and sensitivity analysis by coupling ensemble and deep machine learning methods to quantify influential factors, calibrate energy models, propagate uncertainty, and optimize energy saving measures at community scale. The construction of variable uncertainty database for community building energy systems can be very useful to further analyze energy performance of community buildings. The probabilistic energy models can be created by extracting coordinates and attributes from geographical information system based on approximate Bayesian computation to solve the problems of unknown variables in energy analysis models. The mechanics of aleatory and epistemic uncertainty can be explored using the two-dimension Monte Carlo simulation to identify the patterns of change of energy use in community buildings. The global Sobol sensitivity analysis method will be used to determine the key variables influencing energy use and find out effective energy saving measures. This project would create a comprehensive theory of uncertainty analysis of energy patterns of community buildings and also realize the automation of calibrating and creating building energy models. The results from this research can provide accurate and reliable energy use information to form the theoretical foundation of the design and optimization of community building energy systems. This project will promote the development of ecological city community, low-carbon town, and green campus.
准确可靠的建筑能耗预测是城区建筑能源规划、能源系统配置、运行经济性和碳排放评估的重要基础。针对能源系统变量具有高度不确定性,导致城区建筑能耗预测困难的问题,采用正向和逆向不确定性及敏感性分析理论,耦合集成和深度机器学习算法,进行影响因素定量化、能耗模型标定、能耗不确定性传递和节能方案优化四方面研究。建立城区建筑能源系统变量不确定性数据库,为城区建筑能耗分析提供坚实数据基础;提取地理信息系统中建筑属性数据,基于贝叶斯算法,建立动态能耗模型,解决模型标定中未知参数确定难题;利用二维蒙特卡洛模拟,阐明随机和认知不确定能耗机理,揭示城区建筑能耗变化规律;采用全局敏感性法,解析影响能耗关键因素,确定有效节能措施。本研究将建立城区建筑能耗不确定性分析的系统理论,实现城区建筑能耗模型自动建立,提供精准城区建筑能耗预测,为城区能源系统设计及优化奠定理论基础,促进我国生态城区、低碳城镇和绿色校园等的发展。
结项摘要
针对缺少城区建筑能耗系统化不确定性及敏感性分析方法的问题,本项目以建筑热物理为基础,结合机器学习算法,根据正向和逆向不确定性分析方法,研究城区建筑能耗不确定性和敏感性。从5个方面进行深入研究:建筑输入不确定性数据库的建立;基于地理信息系统的城市建筑能耗模型建立;城区建筑能耗的正向不确定传递;基于近似贝叶斯的建筑能耗模型标定;基于序贯抽样的城区建筑能耗敏感性分析。以提供可靠的城区建筑能耗输入参数不确定性数据为目的,选择轻量型SQLite作为不确定性数据库工具,主要包括建筑围护、暖通空调、人行为、天气参数四类参数约440条数据,建立了一个全面、通用、开放、易用的建筑能耗输入参数不确定性数据库;基于地理信息系统,结合建筑能耗模拟软件与R语言环境,以天津科技大学河西校区建筑群为例,提出一套适用于城区规模建筑能耗模拟研究与节能潜力评估的方法,包括地理信息系统数据处理、自动化建模、机器学习代理模型建立、节能方案可视化评估等;基于二维蒙特卡洛不确定性分析理论,结合地理信息系统和机器学习算法,以天津科技大学泰达校区为研究对象,提出一整套基于城区建筑群能耗模拟的正向不确定性研究方法;耦合近似贝叶斯计算和机器学习标定建筑能耗模型,近似贝叶斯计算可在不计算似然函数的情况下得到模型未知参数的分布,机器学习方法通过建立可靠的建筑能耗机器学习模型,解决贝叶斯反演中建筑能耗模型计算成本过高的问题;提出基于序贯抽样的建筑能耗敏感性研究,动态调整建筑能耗模拟抽样次数,在满足敏感性结果收敛要求前提下显著减少模型计算时间,并提供准确稳定的敏感性结果。本研究提出了城区建筑能耗的不确定性和敏感性分析系统性理论方法,在建筑能耗领域有重要的学术价值和理论意义,对我国建筑节能减排工作中绿色城区、生态小镇、绿色校园等的发展有着非常现实的应用价值。
项目成果
期刊论文数量(8)
专著数量(0)
科研奖励数量(0)
会议论文数量(10)
专利数量(3)
Energy and carbon performance of urban buildings using metamodeling variable importance techniques
使用元建模变量重要性技术的城市建筑的能源和碳性能
- DOI:10.1007/s12273-020-0688-0
- 发表时间:2020-09
- 期刊:Building Simulation
- 影响因子:5.5
- 作者:Liu Yunliang;Tian Wei;Zhou Xiang
- 通讯作者:Zhou Xiang
Energy characteristics of urban buildings: Assessment by machine learning
城市建筑的能源特征:机器学习评估
- DOI:10.1007/s12273-020-0608-3
- 发表时间:2020-03
- 期刊:Building Simulation
- 影响因子:5.5
- 作者:Tian Wei;Zhu Chuanqi;Sun Yu;Li Zhanyong;Yin Baoquan
- 通讯作者:Yin Baoquan
Uncertainty and sensitivity analysis of energy assessment for office buildings based on Dempster-Shafer theory
基于Dempster-Shafer理论的办公建筑能源评估不确定性及敏感性分析
- DOI:10.1016/j.enconman.2018.08.086
- 发表时间:2018-10
- 期刊:Energy Conversion and Management
- 影响因子:10.4
- 作者:Wei Tian;Pieter de Wilde;Zhanyong Li;Jitian Song;Baoquan Yin
- 通讯作者:Baoquan Yin
Uncertainty calibration of building energy models by combining approximate Bayesian computation and machine learning algorithms
近似贝叶斯计算与机器学习算法相结合的建筑能源模型不确定度校准
- DOI:10.1016/j.apenergy.2020.115025
- 发表时间:2020-06
- 期刊:Applied Energy
- 影响因子:11.2
- 作者:Chuanqi Zhu;Wei Tian;Baoquan Yin;Zhanyong Li;Jiaxin Shi
- 通讯作者:Jiaxin Shi
抽样方法与样本数量对建筑能耗机器学习模型精度的影响研究
- DOI:10.13614/j.cnki.11-1962/tu.2020.04.29
- 发表时间:2020
- 期刊:建筑科学
- 影响因子:--
- 作者:朱传琪;田玮;史佳鑫;尹宝泉
- 通讯作者:尹宝泉
数据更新时间:{{ journalArticles.updateTime }}
{{
item.title }}
{{ item.translation_title }}
- DOI:{{ item.doi || "--"}}
- 发表时间:{{ item.publish_year || "--" }}
- 期刊:{{ item.journal_name }}
- 影响因子:{{ item.factor || "--"}}
- 作者:{{ item.authors }}
- 通讯作者:{{ item.author }}
数据更新时间:{{ journalArticles.updateTime }}
{{ item.title }}
- 作者:{{ item.authors }}
数据更新时间:{{ monograph.updateTime }}
{{ item.title }}
- 作者:{{ item.authors }}
数据更新时间:{{ sciAawards.updateTime }}
{{ item.title }}
- 作者:{{ item.authors }}
数据更新时间:{{ conferencePapers.updateTime }}
{{ item.title }}
- 作者:{{ item.authors }}
数据更新时间:{{ patent.updateTime }}
其他文献
模式体系谷氨酸-葡萄糖美拉德反应程度研究
- DOI:10.16429/j.1009-7848.2019.03.006
- 发表时间:2019
- 期刊:中国食品学报
- 影响因子:--
- 作者:张凤梅;汤高奇;田玮;柳艳霞;赵改名;赵莉君
- 通讯作者:赵莉君
煅烧蛋壳制取活性石灰的研究
- DOI:--
- 发表时间:2013
- 期刊:耐火与石灰
- 影响因子:--
- 作者:张玉柱;田玮;郝素菊;尹秀甫
- 通讯作者:尹秀甫
图们江中游稻田节肢动物群落特征及影响因素研究
- DOI:10.13478/j.cnki.jasyu.2016.02.002
- 发表时间:2016
- 期刊:延边大学农学学报
- 影响因子:--
- 作者:田玮;熊琪;吴婷婷;杨萌;相恒星;裴洪淑
- 通讯作者:裴洪淑
石灰的微观结构与其活性度的关系
- DOI:--
- 发表时间:2013
- 期刊:河北联合大学学报(自然科学版)
- 影响因子:--
- 作者:蒋武锋;张玉柱;聂丽霞;田玮
- 通讯作者:田玮
基于主成分分析法的煮制时间对卤制鸡肉风味的影响分析
- DOI:--
- 发表时间:2014
- 期刊:食品与发酵工业
- 影响因子:--
- 作者:武苏苏;赵改名;柳艳霞;田玮;孙灵霞
- 通讯作者:孙灵霞
其他文献
{{
item.title }}
{{ item.translation_title }}
- DOI:{{ item.doi || "--" }}
- 发表时间:{{ item.publish_year || "--"}}
- 期刊:{{ item.journal_name }}
- 影响因子:{{ item.factor || "--" }}
- 作者:{{ item.authors }}
- 通讯作者:{{ item.author }}
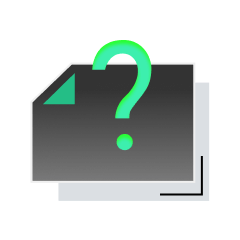
内容获取失败,请点击重试
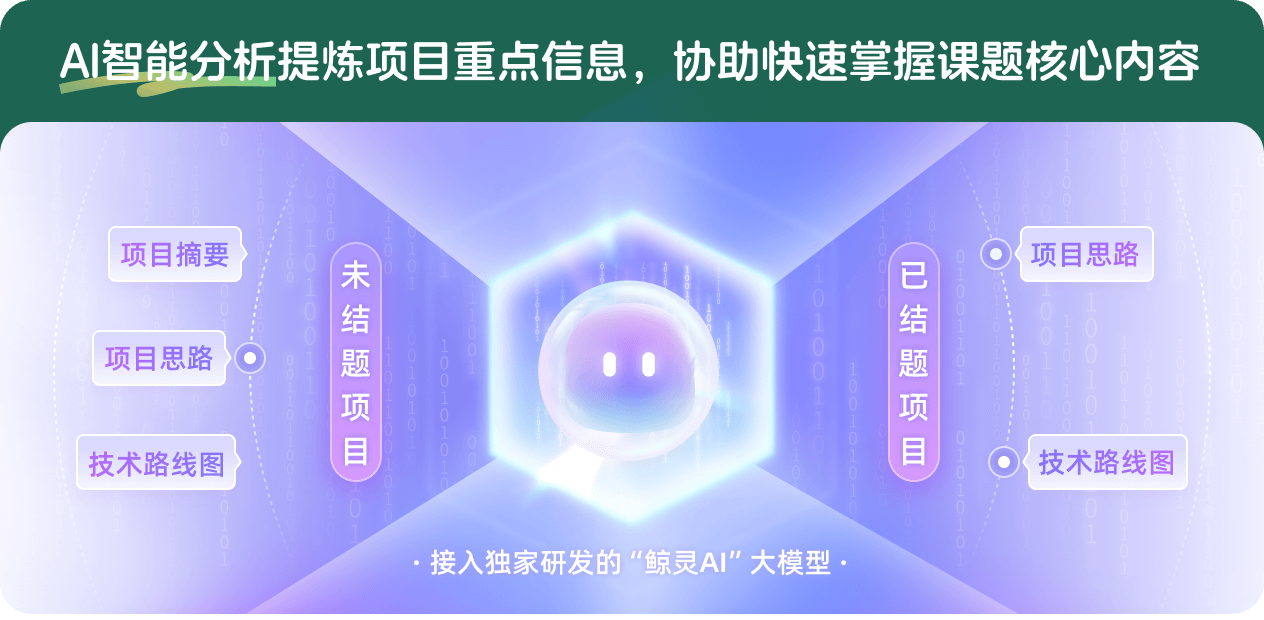
查看分析示例
此项目为已结题,我已根据课题信息分析并撰写以下内容,帮您拓宽课题思路:
AI项目摘要
AI项目思路
AI技术路线图
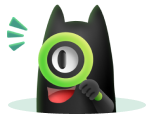
请为本次AI项目解读的内容对您的实用性打分
非常不实用
非常实用
1
2
3
4
5
6
7
8
9
10
您认为此功能如何分析更能满足您的需求,请填写您的反馈:
相似国自然基金
{{ item.name }}
- 批准号:{{ item.ratify_no }}
- 批准年份:{{ item.approval_year }}
- 资助金额:{{ item.support_num }}
- 项目类别:{{ item.project_type }}
相似海外基金
{{
item.name }}
{{ item.translate_name }}
- 批准号:{{ item.ratify_no }}
- 财政年份:{{ item.approval_year }}
- 资助金额:{{ item.support_num }}
- 项目类别:{{ item.project_type }}