基于几何与概率的机器学习算法验证框架
项目介绍
AI项目解读
基本信息
- 批准号:61872371
- 项目类别:面上项目
- 资助金额:63.0万
- 负责人:
- 依托单位:
- 学科分类:F0201.计算机科学的基础理论
- 结题年份:2022
- 批准年份:2018
- 项目状态:已结题
- 起止时间:2019-01-01 至2022-12-31
- 项目参与者:谭庆平; 刘新旺; 于恒彪; 周戈; 张卓;
- 关键词:
项目摘要
With the improvement of the performance of modern computer, machine learning algorithms based on statistics have made a series of breakthroughs, it gives renewed impetus to the development of artificial intelligence. Nowadays, machine learning receives a wide application in almost every field of national economy, as a result, the approach to explaining and controlling of such technique is in urgent need, and this challenging problem attracts more and more attention in academy. Reasons of such difficulty partly are: lacks of approach describing the input/output spaces; the inherit complexity of learning algorithms; and the complexity of evaluation. The outcome of a machine learning algorithm is in general a mapping or a function, which is one of the central objects studied in geometry. Hence, geometry yields a powerful tool to characterize the properties of input/output spaces. In addition, the effect of machine learning only makes sense in a statistical perspective, therefore, we need to use probability in doing such evaluation. In this project, our goal is to establish a formal verification framework for machine learning algorithms based on geometry and probability theory, by giving the modeling approaches, defining the specification languages, and presenting the verification algorithms.
随着计算机性能的日趋提高,基于统计的机器学习方法取得了极大的突破,它也推动了新一轮人工智能的发展。随着其在国民经济领域的广泛应用,对相应算法的可解释性与可控制性的需求日渐强烈,这个具有挑战性的问题也逐渐引起学术界的关注。机器学习算法难解释性源于:对算法输入、输出空间的结构刻画有效手段的缺乏性;学习过程的复杂性;以及学习效果评价的复杂性。多数学习算法的结果本身是一个映射(函数),而这正是几何学中某些分支(如拓扑学)的主要研究内容,因此是学习算法的空间、映射提供刻画的有力工具。此外,学习算法的评估一般是一个统计意义上的结果,这就需要使用概率论中相关结果作为工具。本项目拟给出一个基于几何及概率的机器学习算法验证框架,给出相应的建模方法,定义规约逻辑,并给出相应的验证算法。
结项摘要
机器学习方法(如神经网络算法、聚类算法)的广泛应用使其受到工业界和学术界密切的关注。因此,很多学者尝试从验证和可解释性等方面对机器学习算法本身进行研究。本项目定义了若干能够描述安全性、鲁棒性、可达性等更一般的规约逻辑,并基于数学方法(主要是几何方法、概率方法)建立了针对前向神经网络和循环神经网络的模型检验的算法框架,使得其能够定性或定量的验证,并实现了相应的模型检验工具。基于Koopman方法用若干算子对神经网络进行近似分解,并能够对网络块的贡献度给出评估。项目同时关注机器学习算法本身:基于最优化方法等,对多视图聚类问题给出了一系列新的算法及改进,取得了较好的实验并效果。与此同时,本项目还给出了规约语言 PmuTL(概率mu-演算)acconjunctive 公式可靠完备公理系统、证明了高斯分布 KL-散度的构成拟距离空间、给出了 MDP 博弈上针对 GR(1) 性质的高效综合算法、研究了多方安全计算策略的自动生成等问题。
项目成果
期刊论文数量(4)
专著数量(0)
科研奖励数量(0)
会议论文数量(17)
专利数量(0)
An Axiom System of Probabilistic Mu-Calculus
概率Mu微积分的公理系统
- DOI:10.26599/tst.2020.9010054
- 发表时间:2022
- 期刊:Tsinghua Science and Technology
- 影响因子:6.6
- 作者:Liu Wanwei;Xu Junnan;Jansen David N;Turrini Andrea;Zhang Lijun
- 通讯作者:Zhang Lijun
Computing Sufficient and Necessary Conditions in CTL: A Forgetting Approach
计算 CTL 中的充分必要条件:遗忘方法
- DOI:10.1016/j.ins.2022.10.124
- 发表时间:2022
- 期刊:Information Sciences
- 影响因子:8.1
- 作者:Renyan Feng;Erman Acar;Yisong Wang;Wanwei Liu;Stefan Schlobach;Weiping Ding
- 通讯作者:Weiping Ding
Verifying ReLU Neural Networks from a Model Checking Perspective
从模型检查的角度验证 ReLU 神经网络
- DOI:10.1007/s11390-020-0546-7
- 发表时间:2020-11
- 期刊:JOURNAL OF COMPUTER SCIENCE AND TECHNOLOGY
- 影响因子:0.7
- 作者:Liu Wan-Wei;Song Fu;Zhang Tang-Hao-Ran;Wang Ji
- 通讯作者:Wang Ji
Probabilistic synthesis against GR(1) winning condition
针对 GR(1) 获胜条件的概率综合
- DOI:10.1007/s11704-020-0076-z
- 发表时间:2021-10
- 期刊:Frontiers of Computer Science
- 影响因子:4.2
- 作者:Zhao Wei;Li Rui;Liu Wanwei;Dong Wei;Liu Zhiming
- 通讯作者:Liu Zhiming
数据更新时间:{{ journalArticles.updateTime }}
{{
item.title }}
{{ item.translation_title }}
- DOI:{{ item.doi || "--"}}
- 发表时间:{{ item.publish_year || "--" }}
- 期刊:{{ item.journal_name }}
- 影响因子:{{ item.factor || "--"}}
- 作者:{{ item.authors }}
- 通讯作者:{{ item.author }}
数据更新时间:{{ journalArticles.updateTime }}
{{ item.title }}
- 作者:{{ item.authors }}
数据更新时间:{{ monograph.updateTime }}
{{ item.title }}
- 作者:{{ item.authors }}
数据更新时间:{{ sciAawards.updateTime }}
{{ item.title }}
- 作者:{{ item.authors }}
数据更新时间:{{ conferencePapers.updateTime }}
{{ item.title }}
- 作者:{{ item.authors }}
数据更新时间:{{ patent.updateTime }}
其他文献
基于约束的多面体抽象域的弱接合
- DOI:--
- 发表时间:--
- 期刊:软件学报
- 影响因子:--
- 作者:刘万伟;陈立前;王戟
- 通讯作者:王戟
一种基于变量可达向量的链表抽象方法
- DOI:--
- 发表时间:--
- 期刊:软件学报
- 影响因子:--
- 作者:李仁见;刘万伟;陈立前;王戟;LI Ren-Jian~(1+),LIU Wan-Wei~2,CHEN Li-Qian~1,WANG
- 通讯作者:LI Ren-Jian~(1+),LIU Wan-Wei~2,CHEN Li-Qian~1,WANG
其他文献
{{
item.title }}
{{ item.translation_title }}
- DOI:{{ item.doi || "--" }}
- 发表时间:{{ item.publish_year || "--"}}
- 期刊:{{ item.journal_name }}
- 影响因子:{{ item.factor || "--" }}
- 作者:{{ item.authors }}
- 通讯作者:{{ item.author }}
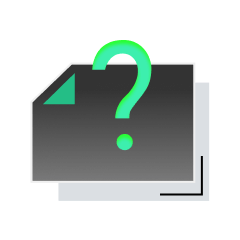
内容获取失败,请点击重试
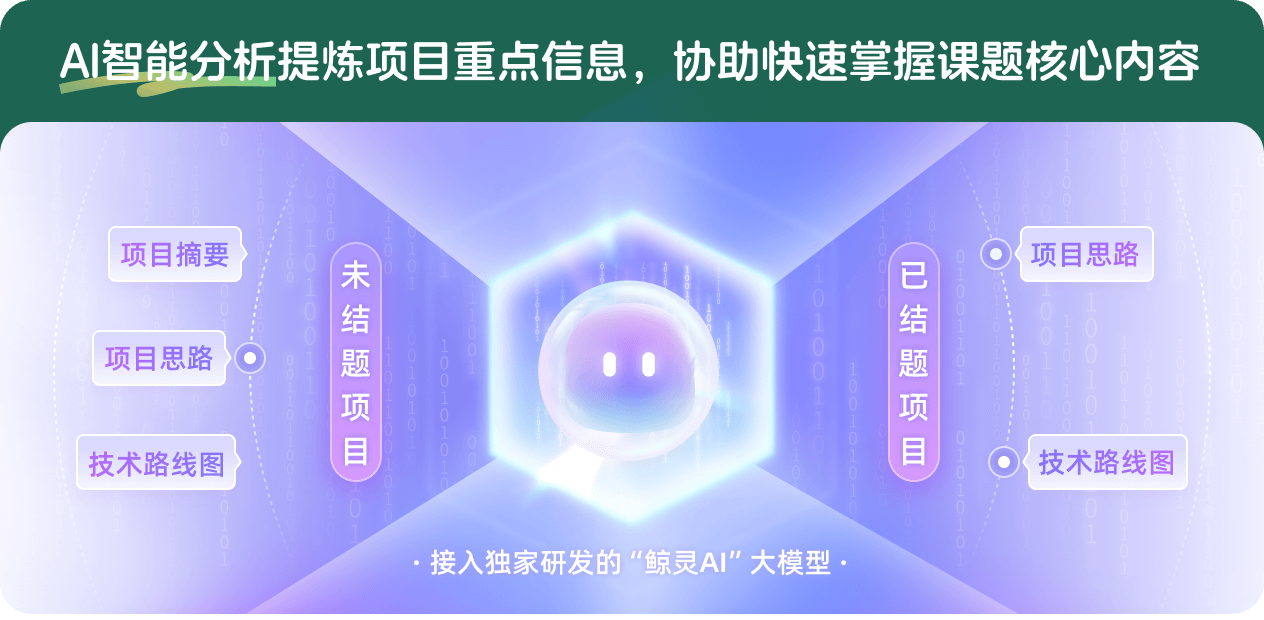
查看分析示例
此项目为已结题,我已根据课题信息分析并撰写以下内容,帮您拓宽课题思路:
AI项目摘要
AI项目思路
AI技术路线图
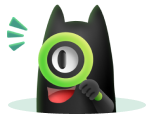
请为本次AI项目解读的内容对您的实用性打分
非常不实用
非常实用
1
2
3
4
5
6
7
8
9
10
您认为此功能如何分析更能满足您的需求,请填写您的反馈:
刘万伟的其他基金
基于 SAT 的扩展时序逻辑的符号化模型检验
- 批准号:61103012
- 批准年份:2011
- 资助金额:23.0 万元
- 项目类别:青年科学基金项目
相似国自然基金
{{ item.name }}
- 批准号:{{ item.ratify_no }}
- 批准年份:{{ item.approval_year }}
- 资助金额:{{ item.support_num }}
- 项目类别:{{ item.project_type }}
相似海外基金
{{
item.name }}
{{ item.translate_name }}
- 批准号:{{ item.ratify_no }}
- 财政年份:{{ item.approval_year }}
- 资助金额:{{ item.support_num }}
- 项目类别:{{ item.project_type }}