基于非均匀采样数据的连续时间系统辨识研究
项目介绍
AI项目解读
基本信息
- 批准号:61703311
- 项目类别:青年科学基金项目
- 资助金额:28.0万
- 负责人:
- 依托单位:
- 学科分类:F0303.系统建模理论与仿真技术
- 结题年份:2020
- 批准年份:2017
- 项目状态:已结题
- 起止时间:2018-01-01 至2020-12-31
- 项目参与者:张世荣; 张磊; 李伟; 唐俊珂; 江磊;
- 关键词:
项目摘要
This project investigates control-oriented identification of industrial processes from irregularly sampled data. Two issues related to this problem are considered, namely: (1) Optimal identification in the presence of colored noise, where optimal means that the model parameter estimates are unbiased and have minimum variance. To this end, most available methods identify discrete-time models of colored noise and apply them to prewhiten the colored noise to achieve the conditions for optimal parameter estimation. However, the traditional treatment is not suitable for irregular sampling since the obtained noise models are typically time varying. To avoid this difficulty, we suggest to model the plant and noise processes in the continuous-time domain, since continuous-time model parameters are independent of sampling period. The main task in this work is to solve the problem of continuous-time noise model identification, and to show the advantages of irregular sampling compared with traditional regular sampling; (2) Time delay system identification. Time delay is an important feature of most physical processes. The use of time delay models has been a common practice to obtain better representations of these processes. By injecting a pure time delay to a linear model, the sluggish property of the system can be well described. A time delay model is generally more difficult to identify due to the nonlinearity introduced by the time delay. The initialization is a critical issue since the loss function is usually multi-modal and local convergence is a common problem. To make the initialization easier, we present several guidelines for the choice of initial parameters, and possible approaches to smooth the loss function shapes. The project aims to enrich the existing theory on industrial process identification, contributing more identification methods to industrial process identification and control design technology under irregular sampling.
本项目围绕非均匀采样和连续时间系统辨识,开展对以下两个科学问题的研究:(1)抗扰辨识,研究在有色噪声干扰下获得系统传递函数模型参数无偏最小方差估计的新方法。为了在非均匀采样下得到时不变模型,提出在连续时间域内对系统进行建模。重点研究辨识连续噪声模型的有效方法,探讨并证明非均匀采样在提高参数估计收敛性能方面的优越之处。讨论如何将连续噪声模型辨识算法与传统辨识算法相结合进而辨识连续Box-Jenkins模型,为非均匀采样系统的控制器设计提供更准确的模;(2)时滞系统辨识,辨识带时滞参数的连续传递函数模型,以实现对大时滞系统动态响应特性更为准确的描述。针对时滞估计中存在的局部收敛问题,提出一系列方法来平滑目标函数,增强算法对初始参数选择的鲁棒性,提高时滞估计的全局收敛性能。本项目成果将进一步丰富现有的工业过程辨识理论,对于提高非均匀采样下工业过程辨识建模和控制技术水平具重要参考意义。
结项摘要
许多实际系统带有固有的时延。准确地估计时延和系统模型参数对于系统分析,预测或控制设计非常重要。但时延的非线性特性使得模型辨识问题更具挑战性,导致模型参数估计容易陷入局部最优。本项目提出了几种辨识方法用于解决时延系统辨识问题,并对算法进行的深入的理论分析,详细阐述了如何将提出的算法用于无线电能传输系统建模。仿真和实验验证结果表明,提出的方法能够很好的处理多(单)输入单输出、时变参数系统辨识问题。
项目成果
期刊论文数量(14)
专著数量(0)
科研奖励数量(0)
会议论文数量(0)
专利数量(0)
Data-driven modeling of wireless power transfer systems with multiple transmitters
具有多个发射器的无线功率传输系统的数据驱动建模
- DOI:10.1109/tpel.2020.2989155
- 发表时间:2020
- 期刊:IEEE Transactions on Power Electronics
- 影响因子:6.7
- 作者:Chen Fengwei;Young Peter C.;Garnier Hugues;Deng Qijun;Kazimierczuk Marian K.
- 通讯作者:Kazimierczuk Marian K.
Frequency domain identification of continuous-time output-error models with time-delay from relay feedback tests
继电器反馈测试中时滞连续时间输出误差模型的频域识别
- DOI:10.1016/j.automatica.2018.09.025
- 发表时间:2018-12
- 期刊:Automatica
- 影响因子:6.4
- 作者:Chen Fengwei;Garnier Hugues;Gilson Marion;Zhuan Xiangtao
- 通讯作者:Zhuan Xiangtao
An Improved Consistent Subspace Identification Method Using Parity Space for State-space Models
状态空间模型奇偶空间的改进一致子空间辨识方法
- DOI:10.1007/s12555-018-0499-6
- 发表时间:2019
- 期刊:International Journal of Control Automation and Systems
- 影响因子:3.2
- 作者:Hou Jie;Chen Fengwei;Li Penghua;Zhu Zhiqin;Liu Fei
- 通讯作者:Liu Fei
Issues in separable identification of continuous-time models with time-delay
时滞连续时间模型的可分离辨识问题
- DOI:10.1016/j.automatica.2018.04.014
- 发表时间:2018-08-01
- 期刊:AUTOMATICA
- 影响因子:6.4
- 作者:Chen, Fengwei;Zhuan, Xiangtao;Gilson, Marion
- 通讯作者:Gilson, Marion
Gray-Box Parsimonious Subspace Identification of Hammerstein-Type Systems
Hammerstein 型系统的灰盒简约子空间辨识
- DOI:10.1109/tie.2020.3026286
- 发表时间:2021-10-01
- 期刊:IEEE TRANSACTIONS ON INDUSTRIAL ELECTRONICS
- 影响因子:7.7
- 作者:Hou, Jie;Chen, Fengwei;Zhu, Zhiqin
- 通讯作者:Zhu, Zhiqin
数据更新时间:{{ journalArticles.updateTime }}
{{
item.title }}
{{ item.translation_title }}
- DOI:{{ item.doi || "--"}}
- 发表时间:{{ item.publish_year || "--" }}
- 期刊:{{ item.journal_name }}
- 影响因子:{{ item.factor || "--"}}
- 作者:{{ item.authors }}
- 通讯作者:{{ item.author }}
数据更新时间:{{ journalArticles.updateTime }}
{{ item.title }}
- 作者:{{ item.authors }}
数据更新时间:{{ monograph.updateTime }}
{{ item.title }}
- 作者:{{ item.authors }}
数据更新时间:{{ sciAawards.updateTime }}
{{ item.title }}
- 作者:{{ item.authors }}
数据更新时间:{{ conferencePapers.updateTime }}
{{ item.title }}
- 作者:{{ item.authors }}
数据更新时间:{{ patent.updateTime }}
其他文献
其他文献
{{
item.title }}
{{ item.translation_title }}
- DOI:{{ item.doi || "--" }}
- 发表时间:{{ item.publish_year || "--"}}
- 期刊:{{ item.journal_name }}
- 影响因子:{{ item.factor || "--" }}
- 作者:{{ item.authors }}
- 通讯作者:{{ item.author }}
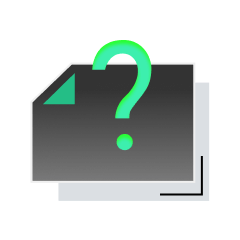
内容获取失败,请点击重试
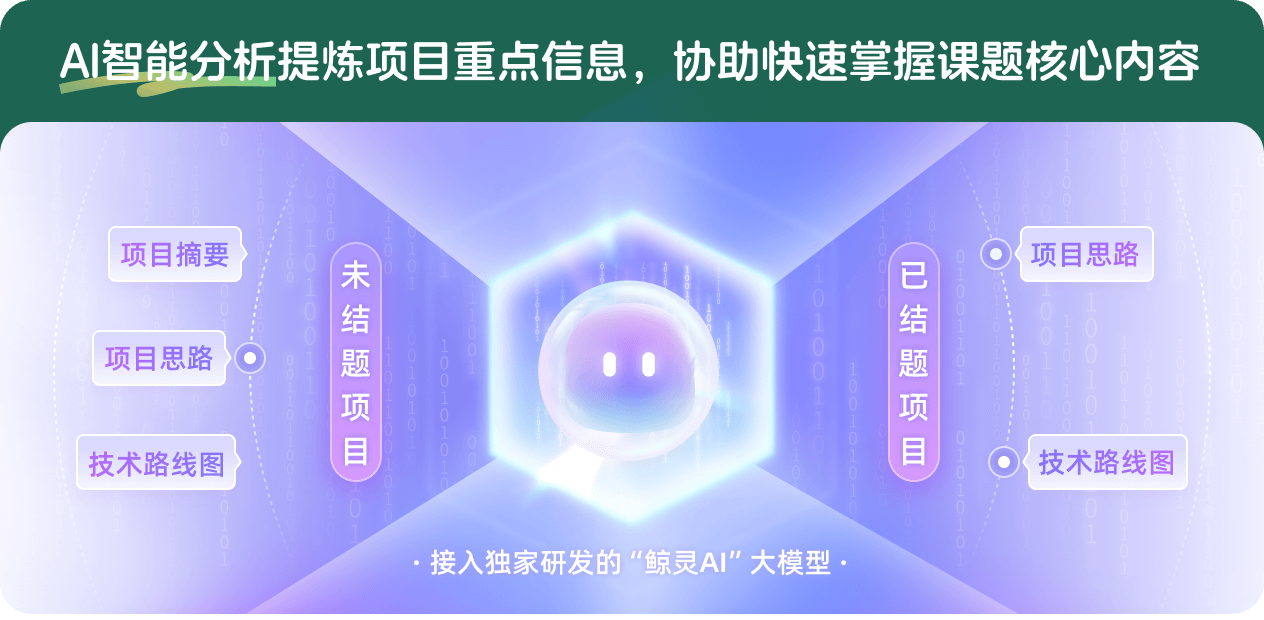
查看分析示例
此项目为已结题,我已根据课题信息分析并撰写以下内容,帮您拓宽课题思路:
AI项目摘要
AI项目思路
AI技术路线图
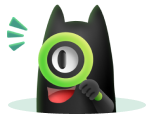
请为本次AI项目解读的内容对您的实用性打分
非常不实用
非常实用
1
2
3
4
5
6
7
8
9
10
您认为此功能如何分析更能满足您的需求,请填写您的反馈:
陈丰伟的其他基金
面向电动汽车无线充电的系统辨识理论与应用
- 批准号:
- 批准年份:2020
- 资助金额:60 万元
- 项目类别:面上项目
相似国自然基金
{{ item.name }}
- 批准号:{{ item.ratify_no }}
- 批准年份:{{ item.approval_year }}
- 资助金额:{{ item.support_num }}
- 项目类别:{{ item.project_type }}
相似海外基金
{{
item.name }}
{{ item.translate_name }}
- 批准号:{{ item.ratify_no }}
- 财政年份:{{ item.approval_year }}
- 资助金额:{{ item.support_num }}
- 项目类别:{{ item.project_type }}