联合虚实SAR影像的灾区地物紧缩极化-全极化信息迁移与解译研究
项目介绍
AI项目解读
基本信息
- 批准号:41901284
- 项目类别:青年科学基金项目
- 资助金额:22.0万
- 负责人:
- 依托单位:
- 学科分类:D0113.遥感科学
- 结题年份:2022
- 批准年份:2019
- 项目状态:已结题
- 起止时间:2020-01-01 至2022-12-31
- 项目参与者:--
- 关键词:
项目摘要
Synthetic Aperture Radar (SAR) can image all-weather and all-time, and its full polarization (FP) mode can obtain the complete backscattering information of targets, so it is an ideal sensor to observe the disaster areas. However, in terms of sensor system, the imaging width and spatial resolution in the FP mode are very limited; in terms of image data, historical images accumulated in the past are often difficult to apply to the current disaster interpretation task, because of the differences of incidence angle, working frequency, temporal and spatial attributes, etc., between historical images and the current image. This project focuses on the above problems, and plans to design a two-stage coupling deep network under the framework of adversarial training, which can make full use of real/virtual SAR data to construct an accurate reconstruction model from compact polarization (CP) data to FP data, and thus the contradiction between polarization information diversity and wide-range/high-resolution will be alleviated; by introducing the transfer learning, this project plans to propose a cross-image information sharing method based on the statistical characteristics of FP data, which will make the historical data more useful. Finally, the disaster interpretation task is completed by combining current/historical data. This project can not only improve the practical application potential of SAR data and provide support for disaster assessment and rescue activities, but also provide reference for the follow-up research and development of CP-SAR satellite in China. It has important scientific significance and application value.
合成孔径雷达(SAR)可全天时全天候成像,其全极化模式(FP)更可获取目标完整后向散射信息,因而是观测灾区的理想传感器。但在系统方面,FP观测的幅宽与空间分辨率十分受限;在数据方面,长期积累的历史影像往往由于与当前影像间存在入射角、工作频率、时空属性等因素差异而导致历史信息难以应用于当前灾害解译工作。本项目聚焦于上述问题开展研究,拟在对抗训练框架下设计二段耦合式深度网络,充分利用真实/虚拟SAR数据构建从紧缩极化(CP)数据到FP数据的精确重构模型,缓解极化信息多样性与大幅宽、高分辨率间的矛盾;在迁移学习思想引导下,拟基于FP数据统计特性建立适用于FP数据的跨影像信息共享方法,降低历史数据的应用门槛;最终,联合当前/历史数据完成灾害解译工作。本项目既可提高雷达数据实际应用潜力,为灾情评估、救援活动提供支撑,又可对后续我国研发CP模式雷达卫星提供参考,具有重要的科学意义和应用价值。
结项摘要
合成孔径雷达(SAR)可全天时全天候成像,其全极化模式(FP)更可获取目标完整后向散射信息,因而是观测灾区的理想传感器。但在技术上,SAR在FP模式下观测幅宽与空间分辨率受限;在实践上,新旧平台获取的FP影像存在成像参数差异,导致二者难以协同应用于灾害解译。本项目针对上述问题开展了一系列研究工作:首先在对抗学习框架下构建了适用于SAR复数域数据输入形式的深度网络模型,利用真实SAR影像和模拟SAR影像联合完成网络训练,实现从紧缩极化(CP)数据到FP数据的重构,缓解了FP影像大幅宽与高分辨率难以兼顾的问题;随后在迁移学习思想引导下,结合SAR数据统计分布设计了特殊的复数域Bregman散度形式及其优化求解策略,以此在成像参数差异条件下实现跨影像信息共享,提高了历史数据重用效率和新旧影像协同效果;最后,基于FP影像开展了房屋倒塌、海洋溢油和城市内涝等典型灾害解译。本项目的研究成果可以为提高SAR数据应用潜力以及灾情评估和相关的灾后救援活动提供支撑,具有较高的科学意义和应用价值。
项目成果
期刊论文数量(3)
专著数量(0)
科研奖励数量(0)
会议论文数量(0)
专利数量(3)
Soil moisture retrieval over agricultural fields from L-band multi-incidence and multitemporal PolSAR observations using polarimetric decomposition techniques
使用极化分解技术从 L 波段多事件和多时相 PolSAR 观测中反演农田土壤湿度
- DOI:10.1016/j.rse.2021.112485
- 发表时间:2021
- 期刊:Remote Sensing of Environment
- 影响因子:13.5
- 作者:Hongtao Shi;Lingli Zhao;Jie Yang;Juan M. Lopez-Sanchez;Jinqi Zhao;Weidong Sun;Lei Shi;Pingxiang Li
- 通讯作者:Pingxiang Li
A Novel Change Detection Method Based on Statistical Distribution Characteristics Using Multi-Temporal PolSAR Data
一种基于多时相PolSAR数据统计分布特征的变化检测新方法
- DOI:10.3390/s20051508
- 发表时间:2020-03
- 期刊:Sensors (Switzerland)
- 影响因子:--
- 作者:Jinqi Zhao;Yonglei Chang;Jie Yang;Yufen Niu;Zhong Lu;Pingxiang Li
- 通讯作者:Pingxiang Li
Scatter Matrix Based Domain Adaptation for Bi-Temporal Polarimetric SAR Images
基于散点矩阵的双时态极化 SAR 图像域自适应
- DOI:10.3390/rs12040658
- 发表时间:2020-02-01
- 期刊:REMOTE SENSING
- 影响因子:5
- 作者:Sun, Weidong;Li, Pingxiang;Zhao, Lingli
- 通讯作者:Zhao, Lingli
数据更新时间:{{ journalArticles.updateTime }}
{{
item.title }}
{{ item.translation_title }}
- DOI:{{ item.doi || "--"}}
- 发表时间:{{ item.publish_year || "--" }}
- 期刊:{{ item.journal_name }}
- 影响因子:{{ item.factor || "--"}}
- 作者:{{ item.authors }}
- 通讯作者:{{ item.author }}
数据更新时间:{{ journalArticles.updateTime }}
{{ item.title }}
- 作者:{{ item.authors }}
数据更新时间:{{ monograph.updateTime }}
{{ item.title }}
- 作者:{{ item.authors }}
数据更新时间:{{ sciAawards.updateTime }}
{{ item.title }}
- 作者:{{ item.authors }}
数据更新时间:{{ conferencePapers.updateTime }}
{{ item.title }}
- 作者:{{ item.authors }}
数据更新时间:{{ patent.updateTime }}
其他文献
其他文献
{{
item.title }}
{{ item.translation_title }}
- DOI:{{ item.doi || "--" }}
- 发表时间:{{ item.publish_year || "--"}}
- 期刊:{{ item.journal_name }}
- 影响因子:{{ item.factor || "--" }}
- 作者:{{ item.authors }}
- 通讯作者:{{ item.author }}
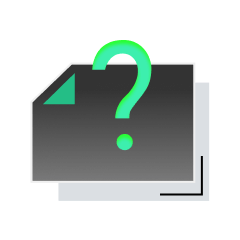
内容获取失败,请点击重试
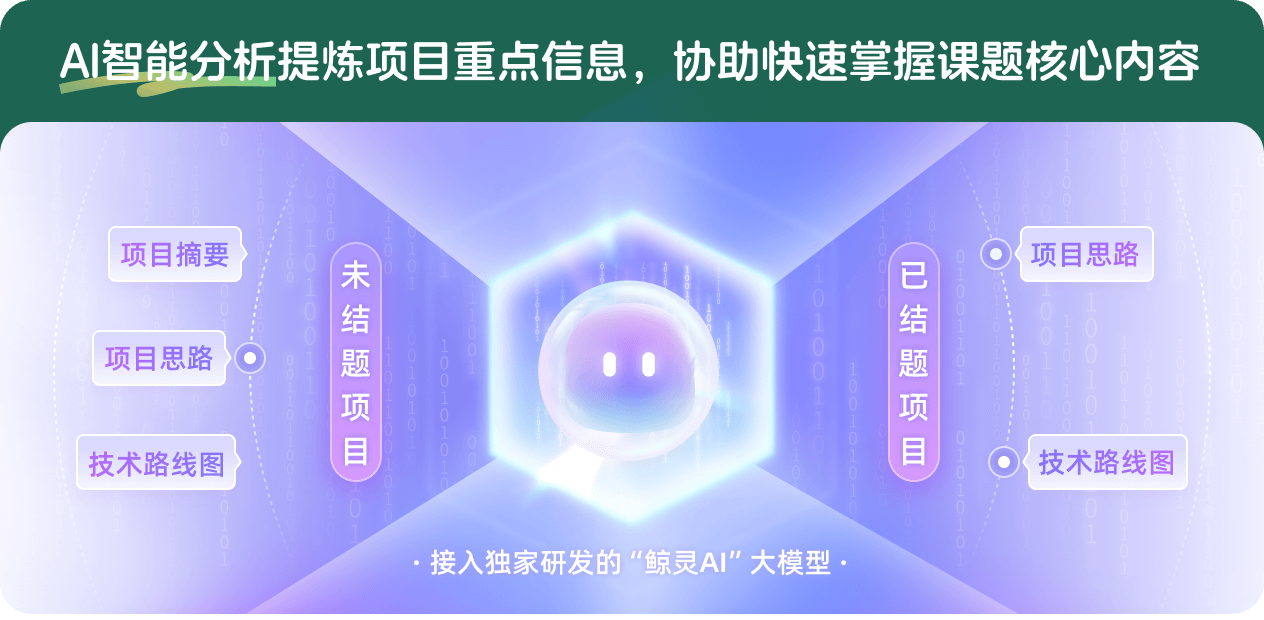
查看分析示例
此项目为已结题,我已根据课题信息分析并撰写以下内容,帮您拓宽课题思路:
AI项目摘要
AI项目思路
AI技术路线图
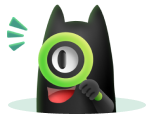
请为本次AI项目解读的内容对您的实用性打分
非常不实用
非常实用
1
2
3
4
5
6
7
8
9
10
您认为此功能如何分析更能满足您的需求,请填写您的反馈:
相似国自然基金
{{ item.name }}
- 批准号:{{ item.ratify_no }}
- 批准年份:{{ item.approval_year }}
- 资助金额:{{ item.support_num }}
- 项目类别:{{ item.project_type }}
相似海外基金
{{
item.name }}
{{ item.translate_name }}
- 批准号:{{ item.ratify_no }}
- 财政年份:{{ item.approval_year }}
- 资助金额:{{ item.support_num }}
- 项目类别:{{ item.project_type }}