基于迁移学习的社交网络虚假新闻检测关键技术研究
项目介绍
AI项目解读
基本信息
- 批准号:61906039
- 项目类别:青年科学基金项目
- 资助金额:24.0万
- 负责人:
- 依托单位:
- 学科分类:F0606.自然语言处理
- 结题年份:2022
- 批准年份:2019
- 项目状态:已结题
- 起止时间:2020-01-01 至2022-12-31
- 项目参与者:--
- 关键词:
项目摘要
The spread of fake news in social networks would cause serious damage to politic, economy and society. The fake news can be detected by using classification models based on content and propagation structure. One of the significant challenges for fake news detection is the lack of data. For instance, the posts in social networks are usually short texts, which contain limited information. It is impractical to get labeled posts and propagation logs for the newly emerged events. As a result, it is difficult to train an effective model for detecting fake news. To tackle the challenge of data sparsity, we propose a fake news detection model based on transfer learning. Specifically, we learn from the posts and propagation structures for the past fake news and transfer the learned knowledge to detect fake news for new events. In this proposal, we first extract common features that are shared among fake news for different events and event-specific features. Then we investigate the propagation of fake news in social networks and identify the key opinion leaders. We propose a classifier to identify fake news based on their propagation structure. Finally, we propose a mix model based on transfer learning to combine the two previous models. The key challenges in this study include multi-model feature analysis, propagation model analysis, stance classification, graph convolutional neural network based propagation graph classification and transfer learning, etc. Our system can be applied to help improve the network ecosystem.
社交网络中虚假新闻的传播对政治、经济和社会有巨大的潜在危害,通过构建基于新闻内容和传播结构的分类模型可以实现虚假新闻的检测。然而,学习分类模型需要大量的标注数据,社交网络中很多事件对应的新闻,尤其是新出现的新闻,并不能收集到足够多的与事件相关的标注数据。为此,本项目拟研究基于迁移学习的虚假新闻检测方法,从已经大量传播、有较多标注数据的过往虚假新闻上学习虚假新闻的泛化特征,并用于提高针对特定事件的虚假新闻的检测上。研究不同事件对应的虚假新闻在其文本内容和传播结构上与真实新闻的泛化区别,研究具体事件对应的虚假新闻在其文本内容和传播结构上与真实新闻的独特区别,研究基于文本和传播结构的混合虚假新闻检测模型。重点研究所遇到的关键科学问题:多模态特征提取、传播能力计算、立场分析、基于图卷积神经网络的传播图分类和迁移学习算法等。取得一些创新性的研究成果,以期为网络生态环境的健康提供关键技术支撑。
结项摘要
社交网络中虚假信息对政治、经济和社会有巨大的潜在危害,寻求积极有效地方法对虚假信息进行监管治理对于构建生态健康的网络舆论环境、维护社会治安、应对信息战和舆论战至关重要。本项目研究内容主要包括(1)研究互联网中的虚假信息检测。提出了一种无监督的谣言检测模型ptVAE。 ptVAE由三个主要组件组成:用户意见模块,传播特征模块和交叉对齐模块;提出一种基于CharacterBert-CRF模型的诈骗词库构建方法,基于诈骗网站和内容和源码自动生成诈骗词库。(2)研究了词嵌入集成模型,集成多个已有的词向量(例如word2vec, GloVe)或者词向量模型(例如BERT)和知识图谱。(3)研究了基于图数据挖掘的表示学习和异常行为检测方法。提出了一种新的模型AER-AD,AER-AD主要包括表示模块和预测模块两个模块;提出了一种基于随机森林和深度自编码高斯混合模型的无监督入侵检测方法RF-DAGMM。(4)研究了社交网络中的信息传播模型,由于双曲空间对嵌入树或者复杂网络结构的天然优势,区别于传统的欧几里得空间建模方法,本项目研究了双曲空间中信息传播。在本项目资助下,共发表论文7篇,申请专利3项。此外,本项目还有2篇论文在审,2篇文章正在修改。其中,以本项目为第一标注且项目申请人为第一/通讯作者的论文包括:IEEE Transactions on Knowledge and Data Engineering (CCF A类期刊)论文2篇,Proceeding of the VLDB Endowment(CCF A类会议)论文1篇,Remoting Sensing (JCR 1区期刊)论文1篇。
项目成果
期刊论文数量(4)
专著数量(0)
科研奖励数量(0)
会议论文数量(3)
专利数量(3)
H-Diffu: Hyperbolic Representations for Information Diffusion Prediction
H-Diffu:信息扩散预测的双曲表示
- DOI:10.1109/tkde.2022.3209067
- 发表时间:2023-09
- 期刊:IEEE Transactions on Knowledge and Data Engineering
- 影响因子:8.9
- 作者:Shanshan Feng;Kaiqi Zhao;Lanting Fang;Kaiyu Feng;Wei Wei;Xutao Li;Ling Shao
- 通讯作者:Ling Shao
基于免疫仿生机理和图神经网络的网络异常检测方法
- DOI:--
- 发表时间:2021
- 期刊:信息网络安全
- 影响因子:--
- 作者:秦中元;胡宁;方兰婷
- 通讯作者:方兰婷
Representing Spatial Data with Graph Contrastive Learning
用图对比学习表示空间数据
- DOI:10.3390/rs15040880
- 发表时间:2023-02
- 期刊:Remote Sensing
- 影响因子:5
- 作者:Lanting Fang;Ze Kou;Yulian Yang;Tao Li
- 通讯作者:Tao Li
A Knowledge-Enriched Ensemble Method for Word Embedding and Multi-Sense Embedding
一种用于词嵌入和多义嵌入的知识丰富的集成方法
- DOI:10.1109/tkde.2022.3159539
- 发表时间:2023-06
- 期刊:IEEE Transactions on Knowledge and Data Engineering
- 影响因子:8.9
- 作者:Lanting Fang;Yong Luo;Kaiyu Feng;Kaiqi Zhao;Aiqun Hu
- 通讯作者:Aiqun Hu
数据更新时间:{{ journalArticles.updateTime }}
{{
item.title }}
{{ item.translation_title }}
- DOI:{{ item.doi || "--"}}
- 发表时间:{{ item.publish_year || "--" }}
- 期刊:{{ item.journal_name }}
- 影响因子:{{ item.factor || "--"}}
- 作者:{{ item.authors }}
- 通讯作者:{{ item.author }}
数据更新时间:{{ journalArticles.updateTime }}
{{ item.title }}
- 作者:{{ item.authors }}
数据更新时间:{{ monograph.updateTime }}
{{ item.title }}
- 作者:{{ item.authors }}
数据更新时间:{{ sciAawards.updateTime }}
{{ item.title }}
- 作者:{{ item.authors }}
数据更新时间:{{ conferencePapers.updateTime }}
{{ item.title }}
- 作者:{{ item.authors }}
数据更新时间:{{ patent.updateTime }}
其他文献
其他文献
{{
item.title }}
{{ item.translation_title }}
- DOI:{{ item.doi || "--" }}
- 发表时间:{{ item.publish_year || "--"}}
- 期刊:{{ item.journal_name }}
- 影响因子:{{ item.factor || "--" }}
- 作者:{{ item.authors }}
- 通讯作者:{{ item.author }}
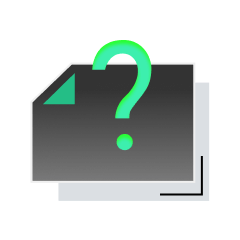
内容获取失败,请点击重试
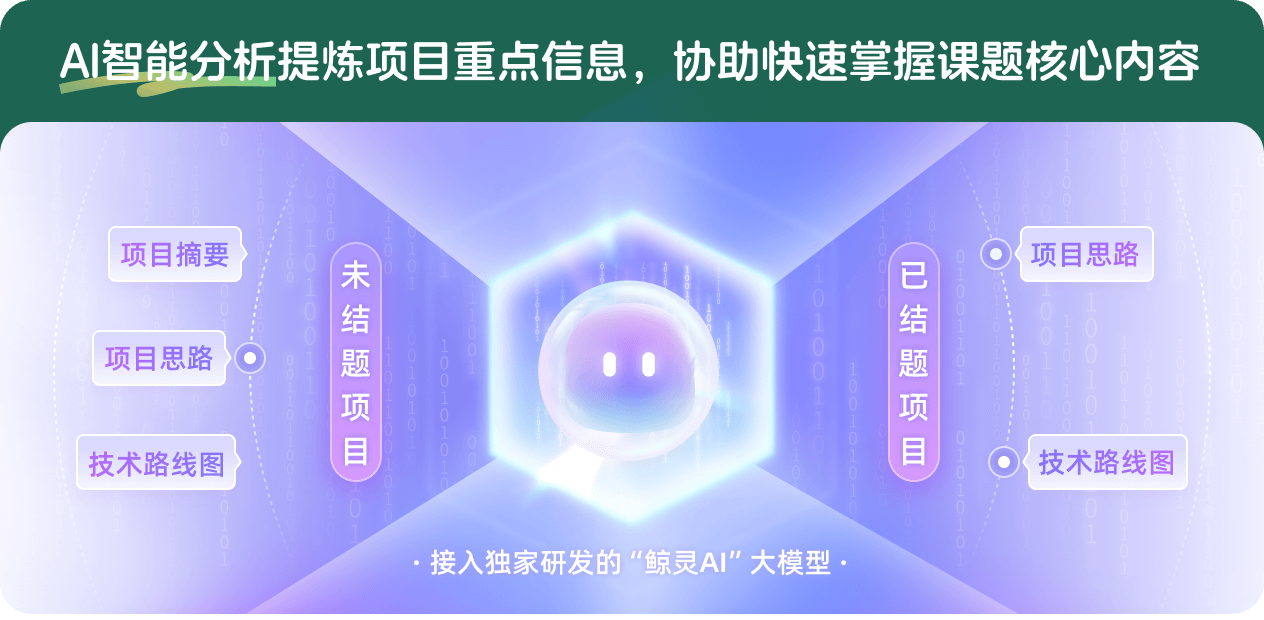
查看分析示例
此项目为已结题,我已根据课题信息分析并撰写以下内容,帮您拓宽课题思路:
AI项目摘要
AI项目思路
AI技术路线图
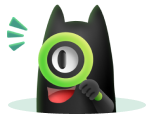
请为本次AI项目解读的内容对您的实用性打分
非常不实用
非常实用
1
2
3
4
5
6
7
8
9
10
您认为此功能如何分析更能满足您的需求,请填写您的反馈:
相似国自然基金
{{ item.name }}
- 批准号:{{ item.ratify_no }}
- 批准年份:{{ item.approval_year }}
- 资助金额:{{ item.support_num }}
- 项目类别:{{ item.project_type }}
相似海外基金
{{
item.name }}
{{ item.translate_name }}
- 批准号:{{ item.ratify_no }}
- 财政年份:{{ item.approval_year }}
- 资助金额:{{ item.support_num }}
- 项目类别:{{ item.project_type }}