面向精准农业的动态作业场景下实时杂草分布密度识别方法研究
项目介绍
AI项目解读
基本信息
- 批准号:31801753
- 项目类别:青年科学基金项目
- 资助金额:23.0万
- 负责人:
- 依托单位:
- 学科分类:C1404.农田草害、鼠害及其他有害生物
- 结题年份:2021
- 批准年份:2018
- 项目状态:已结题
- 起止时间:2019-01-01 至2021-12-31
- 项目参与者:李健; 周婧; 李陈孝; 刘媛媛; 崔菁菁; 朱炽阳; 张奇;
- 关键词:
项目摘要
Variable spraying is an important strategy for realizing site-specific weed management, in which weed distribution identification is the key technology. The traditional weed identification methods are mainly applied to single or narrow-range weed and the speed of algorithm is slow. However, the weed identification under the dynamic operating conditions should recognize the wide-range weed distribution and quantify weed identification results. To solve this problem, this project studies the influence factor of weed distribution density, and introduces BP neural network and adopts learning rule and training mechanism to establish weed distribution model; And combining big data mining technology and support vector machine, weed distribution hierarchy method is established; In on-line system, the weed identification algorithm based on multi-horizontal scanning are proposed to extract dynamic weed distribution parameter in real time. The weed distribution can be calculated by applying weed distribution model and weed distribution hierarchy method. Then the classification of weed distribution is done and the weed identification result is determined, which can optimize the weed distribution model and hierarchy algorithm. The project can provide theoretical and technical support for developing spraying system and further can reduce the using of herbicide.
变量喷药是杂草智能化管理的重要途径,其中杂草分布密度识别是其关键技术。目前的杂草识别方法主要应用于单株或小范围杂草识别,算法处理速度慢,而动态作业场景下杂草识别应能实时准确识别大范围的杂草分布,量化杂草识别结果。针对这一问题,本项目研究杂草分布密度的影响因素,引入弹性BP神经网络的学习规则和训练机理,训练动态杂草样本图像,离线建立动态场景下杂草分布模型;基于支持向量机的大数据深度挖掘技术,研究杂草分布密度分级算法,离线形成杂草分布分级方法。基于边缘检测多水平扫描算法,研究在线杂草分布参数提取算法。在线提取杂草分布参数,应用离线构建的杂草分布模型及杂草分级方法,离线与在线结合,计算杂草分布密度,对杂草分布情况分级,量化杂草识别结果,优化杂草分布模型及分级算法。本项目的实施将为杂草智能化管理及变量喷药系统提供理论及技术基础,促进除草剂的减量使用。
结项摘要
变量喷药技术是杂草智能化管理的重要手段,其中杂草识别是一个研究热点和难点。变量喷药技术要求杂草识别能够在动态作业场景下实施,要求杂草识别算法适应范围广、鲁棒性好、速度快。. 本项目主要针对动态作业场景下的杂草识别技术开展研究,以苗期玉米作物中的杂草为研究对象,主要研究了杂草识别预处理及实时杂草分布参数提取算法,提取动态场景下杂草分布模型参数;研究了杂草分布模型和杂草分级方法,对杂草分布情况分级,确定杂草识别结果。. 项目组研究了顺序搜索粒子群阈值分割算法,缩小粒子群搜索范围,并将最大类间方差法中的粒子方差值作为粒子群优化中的适应度进行计算,确定阈值后,再以该阈值为中心小范围搜索最优值,完成对农田图像的分割。研究了基于Harris绝对特征角点的田间杂草密度快速检测方法,该方法不需要直接对作物行中心线识别、拟合,减少了大量算法负载,节省算法处理空间,处理一幅2748*576像素的图像,该方法仅用时782ms。项目组构建了基于轻量卷积网络的杂草识别模型,改进后的Xception模型测试准确率达98.63%,且模型规模仅为83.5MB,便于模型的部署和移植;改进的Xception模型的单张图片检测平均耗时仅63.8ms,具有更快的检测速度。该模型泛化能力强,识别准确率高,模型规模小,识别检测速度快,适宜于农业田间生产中对杂草实时识别需求。项目组同时研究了基于迁移学习的深度可分离卷积神经网络杂草识别方法,该方法在分类准确率和训练速度上都有显著提高,该模型的测试识别准确率达到99.63%,每轮训练的时间成本为208s。. 本项目研究的面向精准农业的动态作业场景下杂草识别方法,能够快速准确识别玉米作物中的杂草并对杂草分布进行分级,为农田杂草识别系统及变量喷药技术的应用奠定理论基础并提供了技术方法,具有广阔的应用前景及生态意义。
项目成果
期刊论文数量(9)
专著数量(0)
科研奖励数量(0)
会议论文数量(0)
专利数量(2)
An Improved Grey Wolf Optimizer Based on Differential Evolution and OTSU Algorithm
基于差分进化和OTSU算法的改进灰狼优化器
- DOI:10.3390/app10186343
- 发表时间:2020-09-01
- 期刊:APPLIED SCIENCES-BASEL
- 影响因子:2.7
- 作者:Liu, Yuanyuan;Sun, Jiahui;Zhou, Xiaokang
- 通讯作者:Zhou, Xiaokang
A Real-Time Weed Mapping and Precision Herbicide Spraying System for Row Crops.
中耕作物实时杂草测绘和精准除草剂喷洒系统
- DOI:10.3390/s18124245
- 发表时间:2018-12-03
- 期刊:Sensors (Basel, Switzerland)
- 影响因子:--
- 作者:Xu Y;Gao Z;Khot L;Meng X;Zhang Q
- 通讯作者:Zhang Q
Weed Recognition for Depthwise Separable Network Based on Transfer Learning
基于迁移学习的深度可分离网络杂草识别
- DOI:10.32604/iasc.2021.015225
- 发表时间:2021
- 期刊:Intelligent Automation & Soft Computing
- 影响因子:2
- 作者:徐艳蕾;翟钰婷;赵宾;周阳
- 通讯作者:周阳
基于灰狼优化PID控制的变量喷药系统研究
- DOI:10.13427/j.cnki.njyi.2020.05.019
- 发表时间:2020
- 期刊:农机化研究
- 影响因子:--
- 作者:王新东;徐艳蕾;张奇;孟笑天;何润
- 通讯作者:何润
基于轻量卷积网络的田间自然环境杂草识别方法
- DOI:10.13229/j.cnki.jdxbgxb20200733
- 发表时间:2021
- 期刊:吉林大学学报(工学版)
- 影响因子:--
- 作者:徐艳蕾;何润;翟钰婷;赵宾;李陈孝
- 通讯作者:李陈孝
数据更新时间:{{ journalArticles.updateTime }}
{{
item.title }}
{{ item.translation_title }}
- DOI:{{ item.doi || "--"}}
- 发表时间:{{ item.publish_year || "--" }}
- 期刊:{{ item.journal_name }}
- 影响因子:{{ item.factor || "--"}}
- 作者:{{ item.authors }}
- 通讯作者:{{ item.author }}
数据更新时间:{{ journalArticles.updateTime }}
{{ item.title }}
- 作者:{{ item.authors }}
数据更新时间:{{ monograph.updateTime }}
{{ item.title }}
- 作者:{{ item.authors }}
数据更新时间:{{ sciAawards.updateTime }}
{{ item.title }}
- 作者:{{ item.authors }}
数据更新时间:{{ conferencePapers.updateTime }}
{{ item.title }}
- 作者:{{ item.authors }}
数据更新时间:{{ patent.updateTime }}
其他文献
A New AC Driving Method for Current-Programmed
一种新的电流编程交流驱动方法
- DOI:--
- 发表时间:--
- 期刊:半导体学报
- 影响因子:--
- 作者:司玉娟;徐艳蕾;郎六琪;陈新发;刘式墉
- 通讯作者:刘式墉
其他文献
{{
item.title }}
{{ item.translation_title }}
- DOI:{{ item.doi || "--" }}
- 发表时间:{{ item.publish_year || "--"}}
- 期刊:{{ item.journal_name }}
- 影响因子:{{ item.factor || "--" }}
- 作者:{{ item.authors }}
- 通讯作者:{{ item.author }}
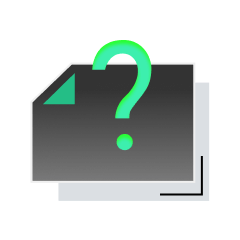
内容获取失败,请点击重试
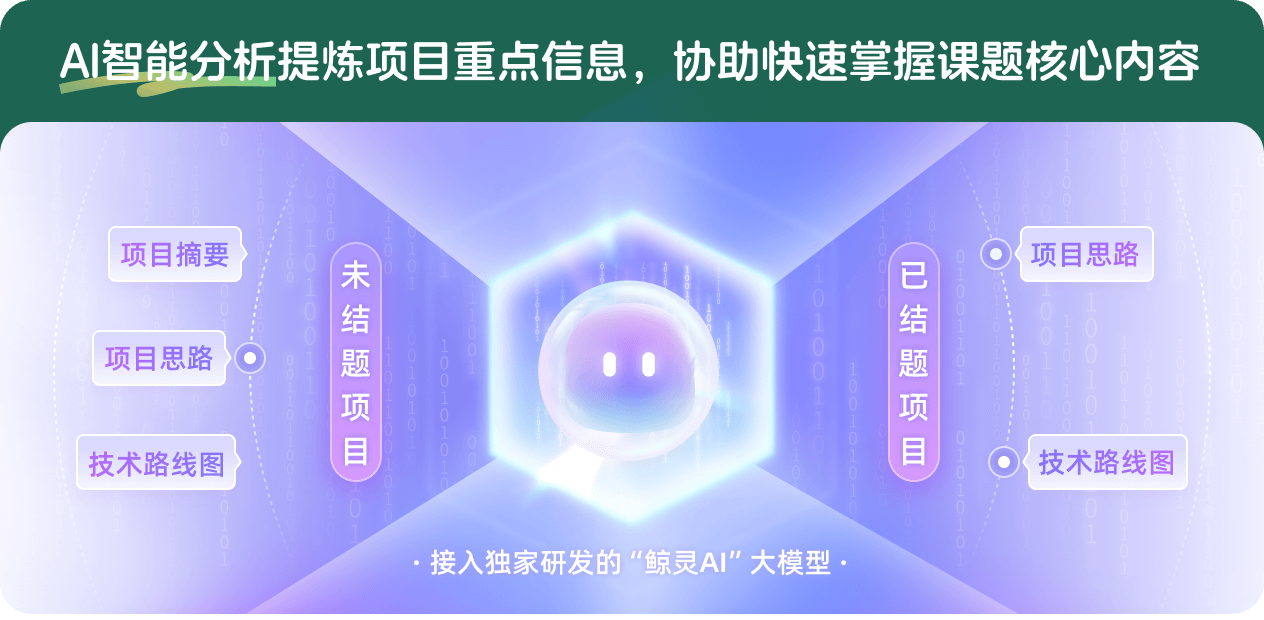
查看分析示例
此项目为已结题,我已根据课题信息分析并撰写以下内容,帮您拓宽课题思路:
AI项目摘要
AI项目思路
AI技术路线图
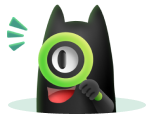
请为本次AI项目解读的内容对您的实用性打分
非常不实用
非常实用
1
2
3
4
5
6
7
8
9
10
您认为此功能如何分析更能满足您的需求,请填写您的反馈:
相似国自然基金
{{ item.name }}
- 批准号:{{ item.ratify_no }}
- 批准年份:{{ item.approval_year }}
- 资助金额:{{ item.support_num }}
- 项目类别:{{ item.project_type }}
相似海外基金
{{
item.name }}
{{ item.translate_name }}
- 批准号:{{ item.ratify_no }}
- 财政年份:{{ item.approval_year }}
- 资助金额:{{ item.support_num }}
- 项目类别:{{ item.project_type }}