大洋岩石圈俯冲变质作用中的元素地球化学行为和地球动力学意义——以西天山超高压变质带为例
项目介绍
AI项目解读
基本信息
- 批准号:41572047
- 项目类别:面上项目
- 资助金额:75.0万
- 负责人:
- 依托单位:
- 学科分类:D0204.岩石学
- 结题年份:2019
- 批准年份:2015
- 项目状态:已结题
- 起止时间:2016-01-01 至2019-12-31
- 项目参与者:王晓红; 胡妍; 李继永; 陈硕;
- 关键词:
项目摘要
The element geochemical behaviors and their controls during seafloor subduction-zone metamorphism remain debatable, e.g., what other controls (except subduction-zone metamorphic dehydration) on different element geochemical behaviors. In addition, because element geochemical behaviors are complex, to fully understand element geochemical behaviors during subduction-zone metamorphism is crucial for understanding the relationship of seafloor subduction-zone metamorphism with arc magmatism and oceanic island basalts. As one ultrahigh pressure metamorphic belt, eclogite-facies rocks from Chinese Western Tianshan honestly recorded the geochemical processes and consequences of seafloor subduction to a depth no less than 75 km. Therefore, it is an ideal study area for seafloor subduction-zone metamorphism. Recently, we got several big blueschist-eclogite rock samples intervened by different types of veins from Western Tianshan. Together with our previous studies on eclogite-facies rocks from Western Tianshan, this proposal with detailed petrological and geochemical studies aims to reveal other controls on element geochemical behaviors during seafloor subduction-zone metamorphism, e.g., the presence and stability of element-hosted minerals, variable physicochemical properties of fluids as well as the way of fluid flow. Furthermore, the understanding of the large-scale mass transfer may help to improve our understandings on the “true” mechanism for geochemical characteristics of arc magmas and the “real” contribution of the residual subducted materials to mantle compositional heterogeneity.
大洋岩石圈俯冲变质作用中的元素地球化学行为及其控制因素尚存在争议:除俯冲变质脱水外,还有哪些影响因素导致元素表现出不同的地球化学行为。此外,由于元素地球化学行为复杂,所以大洋岩石圈俯冲与岛弧岩浆、洋岛玄武岩之间的成因关系也存在争议。因此,充分认识俯冲变质作用中的元素地球化学行为是解决这一问题的关键。中国西天山是全球少数超高压变质带之一,其榴辉岩真实记录了大洋岩石圈俯冲到至少75km深度时的地球化学过程与结果,是研究大洋岩石圈俯冲的理想对象。本项目在西天山榴辉岩相岩石已有研究基础上,对含丰富脉体的蓝片岩-榴辉岩进行详细的岩石学研究,分析脉体和围岩的全岩-矿物主、微量元素组成,探讨除俯冲变质脱水外元素地球化学行为的其他控制因素(如相关变质矿物的存在和稳定性、流体多变的物理-化学性质与流动方式),认识大规模物质迁移过程,从而进一步揭示岛弧岩浆地球化学特征的成因及残余俯冲物质对地幔不均一性的贡献。
结项摘要
大洋岩石圈俯冲变质作用的地球化学过程复杂,而大洋岩石圈俯冲作用与俯冲带岩浆作用、地幔成分不均一性之间的成因关系也存在争议,充分认识俯冲变质作用中的元素地球化学行为、揭示其控制因素是解决这些问题的关键。中国西南天山是全球少数大洋型超高压变质带之一,其榴辉岩相岩石真实记录了大洋岩石圈俯冲到至少75 km深度时的地球化学过程与结果,所以本项目主要集中于对西南天山蓝片岩-榴辉岩相岩石这一研究大洋岩石圈俯冲作用的理想对象。通过对比全球其他(超)高压变质带蓝片岩-榴辉岩的元素地球化学特征,结合对全球岛弧岩浆岩地球化学特征的讨论,系统揭示了俯冲带元素复杂的地球化学行为,探讨了变质温度、压力、原岩成分对俯冲作用地球化学过程的影响。同时,由于脉体是曾经流体运移的通道,富含不同类型脉体的蓝片岩-榴辉岩能够反映流体影响下俯冲带开放体系的地球化学过程,本项目结合详细的岩石学研究,分析了脉体和围岩的全岩-矿物主、微量元素组成,阐明了俯冲带开放体系中元素的地球化学行为、证明了元素赋存变质矿物的存在和稳定性对大洋岩石圈俯冲时元素地球化学行为的控制作用,揭示了不同俯冲阶段流体对俯冲变质岩的地球化学影响。而通过研究西南天山首次发现的榴辉岩相大理岩和变基性岩界面大量顺层生长的榍石,揭示了俯冲碳酸盐对俯冲带地球化学过程的影响和对全球碳循环的意义。并基于对俯冲带变质作用地球化学过程的理解,进一步制约了大洋岩石圈俯冲变质作用对岛弧岩浆地球化学特征的成因和对地幔组分不均一性的贡献。
项目成果
期刊论文数量(4)
专著数量(0)
科研奖励数量(0)
会议论文数量(0)
专利数量(0)
Effects of decarbonation on elemental behaviors during subduction-zone metamorphism: Evidence from a titanite-rich contact between eclogite-facies marble and omphacitite
俯冲带变质作用过程中脱碳作用对元素行为的影响:来自榴辉岩相大理岩和绿辉石之间富钛矿接触的证据
- DOI:10.1016/j.jseaes.2017.01.006
- 发表时间:2017-03
- 期刊:Journal of Asian Earth Sciences
- 影响因子:3
- 作者:Yuanyuan Xiao;Yaoling Niu;Hong-Fu Zhang;Kuo-Lung Wang;Yoshiyuki Iizuka;Jinyan Lin;Yulong Tan;Yongjiang Xu
- 通讯作者:Yongjiang Xu
Different stages of chemical alteration on metabasaltic rocks in the subduction channel: Evidence from the Western Tianshan metamorphic belt, NW China
俯冲通道中变玄武岩不同阶段的化学蚀变:来自中国西北天山变质带的证据
- DOI:10.1016/j.jseaes.2017.06.001
- 发表时间:2017-09
- 期刊:Journal of Asian Earth Sciences
- 影响因子:3
- 作者:Xiao Yuanyuan;Niu Yaoling;Wang Kuo-Lung;Iizuka Yoshiyuki;Lin Jinyan;Wang Dong;Tan Yulong;Wang Guodong
- 通讯作者:Wang Guodong
Geochemical behaviours of chemical elements during subduction-zone metamorphism and geodynamic significance
俯冲带变质作用过程中化学元素的地球化学行为及地球动力学意义
- DOI:10.1080/00206814.2016.1147987
- 发表时间:2016-02
- 期刊:International Geology Review
- 影响因子:2.6
- 作者:Niu, Yaoling;Wang, Kuo-Lung;Lee, Der-Chuen;Iizuka, Yoshiyuki
- 通讯作者:Iizuka, Yoshiyuki
大洋岩石圈俯冲过程中流体活动的岩石地球化学记录:以中国西南天山(超)高压变质岩为例
- DOI:--
- 发表时间:2019
- 期刊:海洋科学
- 影响因子:--
- 作者:王栋;肖媛媛;牛耀龄
- 通讯作者:牛耀龄
数据更新时间:{{ journalArticles.updateTime }}
{{
item.title }}
{{ item.translation_title }}
- DOI:{{ item.doi || "--"}}
- 发表时间:{{ item.publish_year || "--" }}
- 期刊:{{ item.journal_name }}
- 影响因子:{{ item.factor || "--"}}
- 作者:{{ item.authors }}
- 通讯作者:{{ item.author }}
数据更新时间:{{ journalArticles.updateTime }}
{{ item.title }}
- 作者:{{ item.authors }}
数据更新时间:{{ monograph.updateTime }}
{{ item.title }}
- 作者:{{ item.authors }}
数据更新时间:{{ sciAawards.updateTime }}
{{ item.title }}
- 作者:{{ item.authors }}
数据更新时间:{{ conferencePapers.updateTime }}
{{ item.title }}
- 作者:{{ item.authors }}
数据更新时间:{{ patent.updateTime }}
其他文献
微生物代谢组学及其在土壤环境中的研究进展
- DOI:--
- 发表时间:2017
- 期刊:微生物前沿
- 影响因子:--
- 作者:肖媛媛;李海波;李英华;杨忠新
- 通讯作者:杨忠新
常用生存分析模型及其对时依性协变量效应的估计方法
- DOI:--
- 发表时间:2016
- 期刊:中国卫生统计
- 影响因子:--
- 作者:肖媛媛;许传志;赵耐青
- 通讯作者:赵耐青
Fe-Al改性硅藻土的制备及其对土壤Cd污染固定化效果
- DOI:10.13227/j.hjkx.201712031
- 发表时间:2018
- 期刊:环境科学
- 影响因子:--
- 作者:杨雨中;朱健;肖媛媛;谭蓉;王平;陈润华;徐海音;杨雄
- 通讯作者:杨雄
血小板计数与胰腺癌患者的总生存期的关联性研究
- DOI:--
- 发表时间:2016
- 期刊:Cancer Medicine
- 影响因子:4
- 作者:肖媛媛
- 通讯作者:肖媛媛
丹酚酸A对大鼠血浆中同型半胱氨酸调节作用的药动学-药效学结合模型
- DOI:--
- 发表时间:2012
- 期刊:中国药科大学学报
- 影响因子:--
- 作者:陈渊成;王琰;肖媛媛;柳晓泉
- 通讯作者:柳晓泉
其他文献
{{
item.title }}
{{ item.translation_title }}
- DOI:{{ item.doi || "--" }}
- 发表时间:{{ item.publish_year || "--"}}
- 期刊:{{ item.journal_name }}
- 影响因子:{{ item.factor || "--" }}
- 作者:{{ item.authors }}
- 通讯作者:{{ item.author }}
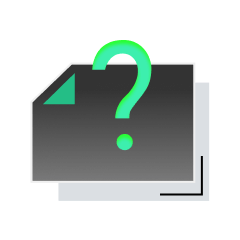
内容获取失败,请点击重试
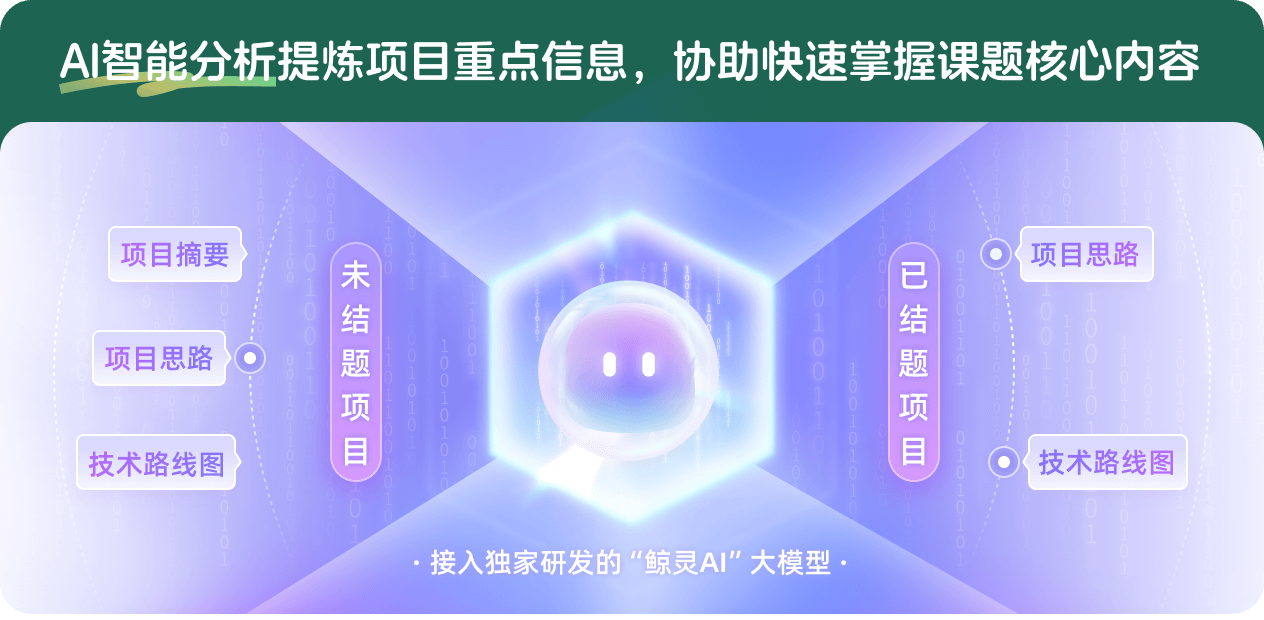
查看分析示例
此项目为已结题,我已根据课题信息分析并撰写以下内容,帮您拓宽课题思路:
AI项目摘要
AI项目思路
AI技术路线图
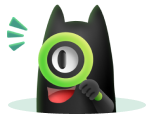
请为本次AI项目解读的内容对您的实用性打分
非常不实用
非常实用
1
2
3
4
5
6
7
8
9
10
您认为此功能如何分析更能满足您的需求,请填写您的反馈:
肖媛媛的其他基金
玻安岩成因研究及其地球动力学意义——以塞浦路斯Troodos地区、北祁连山大岔大坂地区及Bonin弧前Hahajima海山玻安岩为例
- 批准号:41776069
- 批准年份:2017
- 资助金额:71.0 万元
- 项目类别:面上项目
相似国自然基金
{{ item.name }}
- 批准号:{{ item.ratify_no }}
- 批准年份:{{ item.approval_year }}
- 资助金额:{{ item.support_num }}
- 项目类别:{{ item.project_type }}
相似海外基金
{{
item.name }}
{{ item.translate_name }}
- 批准号:{{ item.ratify_no }}
- 财政年份:{{ item.approval_year }}
- 资助金额:{{ item.support_num }}
- 项目类别:{{ item.project_type }}