基于深度集成学习的多模态肺结节检测研究
项目介绍
AI项目解读
基本信息
- 批准号:61902282
- 项目类别:青年科学基金项目
- 资助金额:23.0万
- 负责人:
- 依托单位:
- 学科分类:F0213.生物信息计算与数字健康
- 结题年份:2022
- 批准年份:2019
- 项目状态:已结题
- 起止时间:2020-01-01 至2022-12-31
- 项目参与者:--
- 关键词:
项目摘要
Lung cancer is one of the most malignant tumors with the highest cancer morbidity and mortality worldwide. Given the complexity and heterogeneous of lung medical images, single-mode lung medical imaging has certain limitations in the characteristic expression of pulmonary nodules. Over-fitting easily occurs because the size of lung medical imaging is generally small. Single deep neural network model has problems of high variance, low deviation, and being sensitive to parameters, which can easily affect the predictions. It is imperative that deep learning algorithms based pulmonary nodule detection model needs to be established in terms of the current theoretical development and application requirements. The major research contents include: (1) Develop the pulmonary nodule detection method of multimode feature fusion, integrate the diverse and complementary information of multimode images, to fully characterize the intrinsic properties of pulmonary nodules; (2)Build multi-scale input pattern recognition and prediction model for pulmonary nodule detection, and effectively explore the consistent imaging features most relevant to the disease, to adapt to the differences of individual and nodule size; (3)Construct a convolution neural network model of multisource heterogeneous based knowledge transfer learning, to advance the learning performance of deep learning model for small-scale lung images; (4)Develop a classification method based on deep ensemble learning for lung nodules by weighting the contributions to the integrated prediction of multiple neural network models, to improve the detection performance of the proposed model.
肺癌是世界范围内癌症发病率和死亡率最高的恶性肿瘤之一。鉴于肺部医学影像的复杂性和异质性,单一模态的医学影像在肺结节特征表达上具有一定的局限性。肺部医学影像数据样本量普遍偏小易造成模型训练过拟合。单个深度神经网络模型具有高方差、低偏差、参数敏感等问题,易对预测结果造成影响。因此,当前的理论发展和应用需求都迫切需要在深度学习的基础上发展新的肺结节检测方法。本项目的主要研究内容包括:(1)发展基于多模态特征融合的肺结节检测方法,融合多模态图像获取的差异性和互补性信息,全面刻画结节的内在固有特性;(2)构建多尺度输入肺结节预测模型,有效挖掘适应个体和结节尺寸差异的与疾病最相关的一致性影像学特征;(3)构建基于多源异构知识迁移的深度学习模型,提高面向小规模肺部影像的深度学习模型的学习性能;(4)发展基于深度集成学习模型的肺结节分类方法,自适应加权多个神经网络模型对集成预测的贡献,提高模型检测性能。
结项摘要
鉴于肺部医学影像的复杂性和异质性,针对传统基于肺部医学影像的计算机辅助检测/诊断系统的局限性,在互联网图像大数据的驱动下,面向肺结节精确检测和诊断难点、肺部医学图像数据量偏小、多尺度特征提取和多模态数据融合等问题,研究和探索适用于目标任务的基于深度学习模型的新的算法和模型。具体研究内容包括:(1)利用深度学习网络的特征自学习能力,基于迁移学习理论,研究并提出基于残差网络的深度特征提取和肺结节分类模型,减少传统特征提取工作量及样本量不充足和人工干预的影响,提高分类精度。(2)针对肺结节精确诊断难点,将肺结节良恶性分类问题类比为细粒度分类任务,同时结合卷积注意力机制的优势,提出基于注意力机制特征提取的细粒度肺结节良恶性分类模型,模型在区域建议模块和监督模块的相互作用与指导下提取信息量最大的区域进行特征表达,作为诊断依据。(3)鉴于多头自注意力机制的局部信息建模和长程信息建模能力,研究并构建基于多头自注意力机制的肺结节良恶性分类模型,模型能够同时提取图像的局部和全局特征,且更加关注重点区域,抑制无效信息。(4)为了减少周围组织对结节良恶性判定带来的干扰,研究设计了一种基于多模式特征融合网络的肺结节良恶性分类方法,以此来挖掘更多有价值的重要特征,提升算法分类性能。(5)围绕项目研究目标结合实际应用需求,还提出基于深度学习的空间注意力叠加和多层特征融合方法、提出了一种面向新型冠状病毒的多尺度自动检测网络、提出了一种面向开集图像分类的归一化最大边缘损失等一系列新的理论和方法,进一步扩展了相关研究内容和应用领域。这些研究工作为计算机辅助医学影像诊断的进一步发展进行了理论和方法上的探索。
项目成果
期刊论文数量(5)
专著数量(0)
科研奖励数量(0)
会议论文数量(2)
专利数量(0)
Improving Pneumonia Classification and Lesion Detection Using Spatial Attention Superposition and Multilayer Feature Fusion
利用空间注意力叠加和多层特征融合改进肺炎分类和病变检测
- DOI:10.3390/electronics11193102
- 发表时间:2022-09
- 期刊:Electronics
- 影响因子:2.9
- 作者:Kang Li;Fengbo Zheng;Panpan Wu;Qiuyuan Wang;Gongbo Liang;Lifen Jiang
- 通讯作者:Lifen Jiang
Normalized Maximal Margin Loss for Open-Set Image Classification
开放集图像分类的归一化最大边缘损失
- DOI:10.1109/access.2021.3068042
- 发表时间:2021-01-01
- 期刊:IEEE ACCESS
- 影响因子:3.9
- 作者:Ma, Chunmei;Sun, Huazhi;Sun, Jingwei
- 通讯作者:Sun, Jingwei
A new multi-focus image fusion method based on multi-classification focus learning and multi-scale decomposition
一种基于多分类焦点学习和多尺度分解的多焦点图像融合新方法
- DOI:10.1007/s10489-022-03658-2
- 发表时间:2022-04
- 期刊:Applied Intelligence
- 影响因子:5.3
- 作者:Lifeng Ma;Yanxiang Hu;Bo Zhang;Jiaqi Li;Zhijie Chen;Wenhao Sun
- 通讯作者:Wenhao Sun
Human Activity Recognition with Multimodal Sensing of Wearable Sensors
利用可穿戴传感器的多模态传感进行人类活动识别
- DOI:10.53106/199115992021123206003
- 发表时间:2021-12
- 期刊:Journal of Computers (Taiwan)
- 影响因子:--
- 作者:Chunmei Ma;Hui Zhao;Ying Li;Panpan Wu;Tao Zhang;Bojue Wang
- 通讯作者:Bojue Wang
Classification of Lung Nodules Based on Deep Residual Networks and Migration Learning
基于深度残差网络和迁移学习的肺结节分类
- DOI:10.1155/2020/8975078
- 发表时间:2020-03
- 期刊:Computational Intelligence and Neuroscience
- 影响因子:--
- 作者:Panpan Wu;Xuanchao Sun;Ziping Zhao;Haishuai Wang;Shirui Pan;Bjoern Schuller
- 通讯作者:Bjoern Schuller
数据更新时间:{{ journalArticles.updateTime }}
{{
item.title }}
{{ item.translation_title }}
- DOI:{{ item.doi || "--"}}
- 发表时间:{{ item.publish_year || "--" }}
- 期刊:{{ item.journal_name }}
- 影响因子:{{ item.factor || "--"}}
- 作者:{{ item.authors }}
- 通讯作者:{{ item.author }}
数据更新时间:{{ journalArticles.updateTime }}
{{ item.title }}
- 作者:{{ item.authors }}
数据更新时间:{{ monograph.updateTime }}
{{ item.title }}
- 作者:{{ item.authors }}
数据更新时间:{{ sciAawards.updateTime }}
{{ item.title }}
- 作者:{{ item.authors }}
数据更新时间:{{ conferencePapers.updateTime }}
{{ item.title }}
- 作者:{{ item.authors }}
数据更新时间:{{ patent.updateTime }}
其他文献
不同种类夏绿肥对设施土壤的改良效应
- DOI:--
- 发表时间:2021
- 期刊:中国土壤与肥料
- 影响因子:--
- 作者:程文龙;李敏;韩上;卜容燕;王慧;唐杉;武盼盼;张军;葛自兵;武际
- 通讯作者:武际
金纳米旋转椭球的光吸收和散射特性优化
- DOI:--
- 发表时间:2020
- 期刊:光学学报
- 影响因子:--
- 作者:夏伊丁·亚库普;帕尔哈提江·吐尔孙;武盼盼
- 通讯作者:武盼盼
去除取样法估算鳄蜥的种群数量
- DOI:10.19692/j.cnki.gfs.2019.01.020
- 发表时间:2019
- 期刊:广西林业科学
- 影响因子:--
- 作者:罗树毅;阳春生;武盼盼;何家松;秦建汉;谢鸿鑫;武正军
- 通讯作者:武正军
金纳米旋转椭球的折射率传感特性分析与优化
- DOI:--
- 发表时间:2019
- 期刊:光散射学报
- 影响因子:--
- 作者:夏伊丁·亚库普;帕尔哈提江·吐尔孙;武盼盼
- 通讯作者:武盼盼
老年脑卒中患者的心理适应:基于认知适应理论 Psychological Adjustment of Elderly Stroke Survivors: Based on the Theory of Cognitive Adaptation
老年脑卒中幸存者的心理调节:基于认知适应理论
- DOI:--
- 发表时间:2017
- 期刊:
- 影响因子:--
- 作者:任奕凝;武盼盼;邵景进;肖露霞
- 通讯作者:肖露霞
其他文献
{{
item.title }}
{{ item.translation_title }}
- DOI:{{ item.doi || "--" }}
- 发表时间:{{ item.publish_year || "--"}}
- 期刊:{{ item.journal_name }}
- 影响因子:{{ item.factor || "--" }}
- 作者:{{ item.authors }}
- 通讯作者:{{ item.author }}
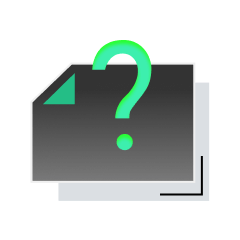
内容获取失败,请点击重试
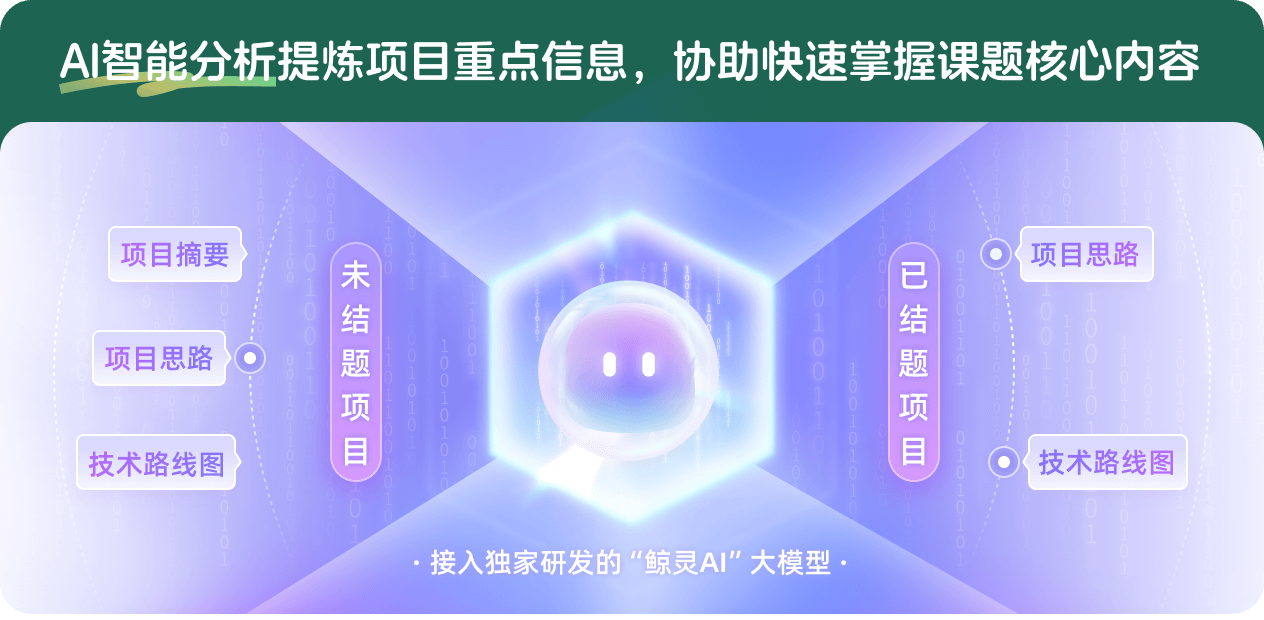
查看分析示例
此项目为已结题,我已根据课题信息分析并撰写以下内容,帮您拓宽课题思路:
AI项目摘要
AI项目思路
AI技术路线图
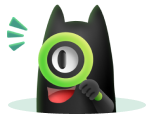
请为本次AI项目解读的内容对您的实用性打分
非常不实用
非常实用
1
2
3
4
5
6
7
8
9
10
您认为此功能如何分析更能满足您的需求,请填写您的反馈:
相似国自然基金
{{ item.name }}
- 批准号:{{ item.ratify_no }}
- 批准年份:{{ item.approval_year }}
- 资助金额:{{ item.support_num }}
- 项目类别:{{ item.project_type }}
相似海外基金
{{
item.name }}
{{ item.translate_name }}
- 批准号:{{ item.ratify_no }}
- 财政年份:{{ item.approval_year }}
- 资助金额:{{ item.support_num }}
- 项目类别:{{ item.project_type }}