深度学习的分数阶微分方程建模与快速算法研究
项目介绍
AI项目解读
基本信息
- 批准号:61906144
- 项目类别:青年科学基金项目
- 资助金额:24.0万
- 负责人:
- 依托单位:
- 学科分类:F0603.机器学习
- 结题年份:2022
- 批准年份:2019
- 项目状态:已结题
- 起止时间:2020-01-01 至2022-12-31
- 项目参与者:--
- 关键词:
项目摘要
The difficulty of the deep neural network (DNN) in information propagation, which includes feature diminishing and gradient vanishing/exploding, is currently the most critical problem in deep learning, and heavily impedes the effective training of deep neural network..Build upon the optimal control theory and the fractional order differential equation, this project offers a theoretically solid and computationally efficient network modelling and optimization method to deal with the information propagation difficulty of the DNN. The main contents include the construction of DNNs with long term memory based on the fractional order differential equation; The design of efficient and effective optimization algorithms based on the maximum principle of the optimal control and stable numerical computation methods. The innovation of this project lies in developing a new framework for network modelling via fractional order differential equation and developing advanced network training algorithms on the strength of optimal control theory,these two aspects are well integrated to smooth the information propagation in current DNN. This project is the first to study the deep learning method via fractional order differential equations, it moves one step forward towards bridging the gap between deep learning and optimal control and will provide a solid theoretical basis and advanced numerical method for further studies of the design and optimization of DNN.
深度神经网络的信息传递障碍,包括:正向传播时的信息丢失、反向传播时的梯度消失/爆炸,是目前深度学习方法研究诸多难题中最关键的问题,掣肘着深层网络的有效训练。.本项目从最优控制理论出发,基于分数阶微分方程对深度神经网络及其优化算法进行建模,通过网络结构和优化算法两方面来解决信息传递障碍问题。主要研究内容有:基于分数阶微分方程及差分方法建立具有长期记忆模式的神经网络模型;基于最优控制的庞特里亚金极大值原理及微分方程数值方法设计更加高效的参数优化算法。项目的创新之处是首次通过分数阶微分方程对深度神经网络进行建模,同时基于数值计算的方法设计神经网络的优化算法。在解决信息传递障碍问题的同时为深度学习的进一步研究提供了新的思路,为深度学习理论研究和最优控制理论研究搭建了很好地桥梁。项目的完成将解决目前深层网络难以训练的问题,大大促进深度学习方法的进一步发展,使深度学习理论研究实现新的突破。
结项摘要
根据最优控制理论,本项目利用分数阶最优控制对深度神经网络及其优化算法进行建模 ,通过网络结构和优化算法两方面来解决信息传递障碍问题。项目主要研究以下两个方面内容:基于分数阶微分方程及差分方法建立具有长期记忆模式的神经网络模型;基于最优控制的庞特里亚金极大值原理及微分方程数值方法设计更加高效的参数优化算法。基于上述两个方面的内容,项目首次提出了分数阶微分方程对应的深层神经网络,该方法将图像去噪任务的性能提升0.1dB。为了更好地刻画损失反向传播,提出对偶非自治系统,有效避免了深度学习中损失函数反向传播过冲中梯度消失问题。本项目在解决信息传递障碍问题的同时为深度学习的进一步研究提供了新的思路,为深度学习理论研究和最优控制理论研究搭建了很好地桥梁。项目有效的解决了目前深层网络难以训练的问题,大大促进深度学习方法的进一步发展,使深度学习理论研究实现新的突破。
项目成果
期刊论文数量(4)
专著数量(0)
科研奖励数量(0)
会议论文数量(0)
专利数量(0)
generalized unitarily invariant gauge regularization for fast low-rank matrix recovery
用于快速低秩矩阵恢复的广义酉不变规范正则化
- DOI:10.1109/tnnls.2020.2985850
- 发表时间:2021
- 期刊:IEEE Transactions on Neural Networks and Learning Systems
- 影响因子:10.4
- 作者:Xixi Jia;Xiangchu Feng;Weiwei Wang;Lei Zhang
- 通讯作者:Lei Zhang
PDNet: Progressive denoising network via stochastic supervision on reaction-diffusion–advection equation
PDNet:通过对反应扩散平流方程进行随机监督的渐进式降噪网络
- DOI:10.1016/j.ins.2022.07.138
- 发表时间:2022
- 期刊:Information Sciences
- 影响因子:8.1
- 作者:Xixi Jia;Deyu Meng;Xu;e Zhang;Xiangchu Feng
- 通讯作者:Xiangchu Feng
Dual non-autonomous deep convolutional neural network for image denoising
用于图像去噪的双非自主深度卷积神经网络
- DOI:10.1016/j.ins.2021.05.001
- 发表时间:2021-05
- 期刊:Information Sciences
- 影响因子:8.1
- 作者:Xixi Jia;Xiangchu Feng;Sanyang Liu
- 通讯作者:Sanyang Liu
Weight Decay With Tailored Adam on Scale-Invariant Weights for Better Generalization.
使用定制 Adam 在尺度不变权重上进行权重衰减,以实现更好的泛化。
- DOI:10.1109/tnnls.2022.3213536
- 发表时间:2022
- 期刊:IEEE transactions on neural networks and learning systems
- 影响因子:10.4
- 作者:Xixi Jia;Xiangchu Feng;Hongwei Yong;Deyu Meng
- 通讯作者:Deyu Meng
数据更新时间:{{ journalArticles.updateTime }}
{{
item.title }}
{{ item.translation_title }}
- DOI:{{ item.doi || "--"}}
- 发表时间:{{ item.publish_year || "--" }}
- 期刊:{{ item.journal_name }}
- 影响因子:{{ item.factor || "--"}}
- 作者:{{ item.authors }}
- 通讯作者:{{ item.author }}
数据更新时间:{{ journalArticles.updateTime }}
{{ item.title }}
- 作者:{{ item.authors }}
数据更新时间:{{ monograph.updateTime }}
{{ item.title }}
- 作者:{{ item.authors }}
数据更新时间:{{ sciAawards.updateTime }}
{{ item.title }}
- 作者:{{ item.authors }}
数据更新时间:{{ conferencePapers.updateTime }}
{{ item.title }}
- 作者:{{ item.authors }}
数据更新时间:{{ patent.updateTime }}
其他文献
方向扩散方程修正BM3D图像去噪改进算法
- DOI:--
- 发表时间:2017
- 期刊:西安电子科技大学学报
- 影响因子:--
- 作者:冯象初;李晓晖;王卫卫;贾西西
- 通讯作者:贾西西
图像恢复问题的梯度稀疏化正则方法
- DOI:--
- 发表时间:2017
- 期刊:系统工程与电子技术
- 影响因子:--
- 作者:赵晨萍;冯象初;王卫卫;贾西西
- 通讯作者:贾西西
其他文献
{{
item.title }}
{{ item.translation_title }}
- DOI:{{ item.doi || "--" }}
- 发表时间:{{ item.publish_year || "--"}}
- 期刊:{{ item.journal_name }}
- 影响因子:{{ item.factor || "--" }}
- 作者:{{ item.authors }}
- 通讯作者:{{ item.author }}
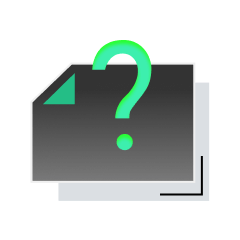
内容获取失败,请点击重试
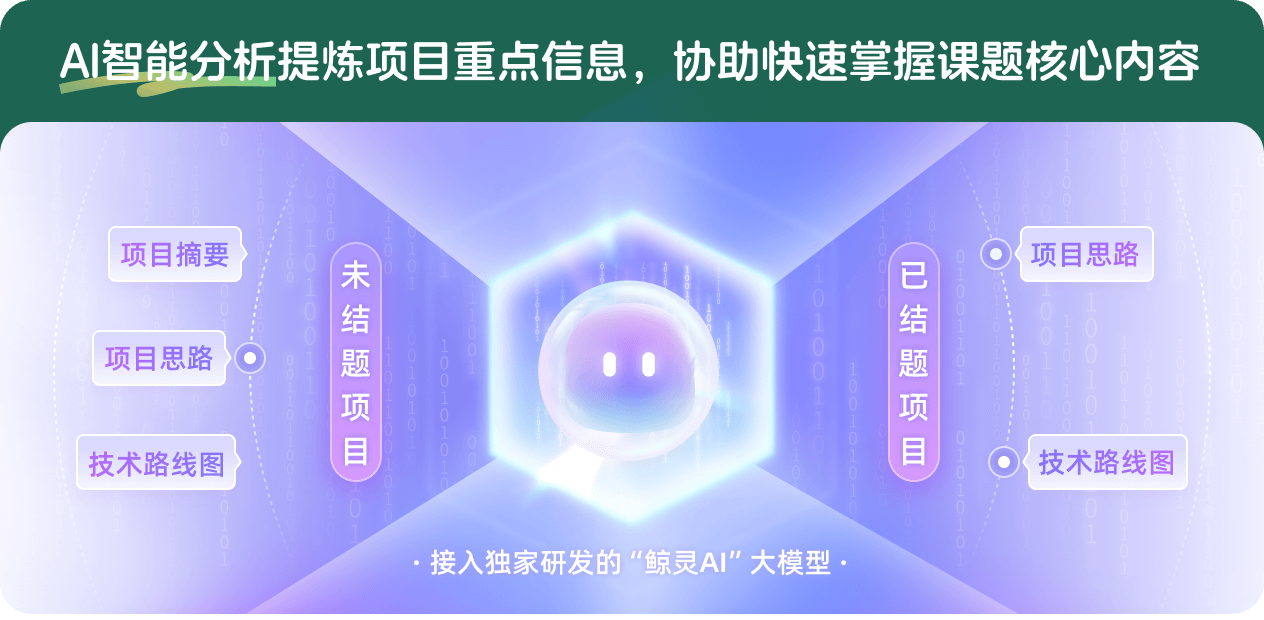
查看分析示例
此项目为已结题,我已根据课题信息分析并撰写以下内容,帮您拓宽课题思路:
AI项目摘要
AI项目思路
AI技术路线图
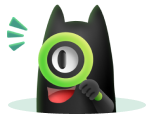
请为本次AI项目解读的内容对您的实用性打分
非常不实用
非常实用
1
2
3
4
5
6
7
8
9
10
您认为此功能如何分析更能满足您的需求,请填写您的反馈:
贾西西的其他基金
分数阶微分引导的深度学习方法研究
- 批准号:62372359
- 批准年份:2023
- 资助金额:50 万元
- 项目类别:面上项目
相似国自然基金
{{ item.name }}
- 批准号:{{ item.ratify_no }}
- 批准年份:{{ item.approval_year }}
- 资助金额:{{ item.support_num }}
- 项目类别:{{ item.project_type }}
相似海外基金
{{
item.name }}
{{ item.translate_name }}
- 批准号:{{ item.ratify_no }}
- 财政年份:{{ item.approval_year }}
- 资助金额:{{ item.support_num }}
- 项目类别:{{ item.project_type }}