基于刀切经验似然方法的有效统计推断
项目介绍
AI项目解读
基本信息
- 批准号:11201207
- 项目类别:青年科学基金项目
- 资助金额:22.0万
- 负责人:
- 依托单位:
- 学科分类:A0403.贝叶斯统计与统计应用
- 结题年份:2015
- 批准年份:2012
- 项目状态:已结题
- 起止时间:2013-01-01 至2015-12-31
- 项目参与者:周望; 胡潇潇; 冯秀英; 甘燕; 赵瑞;
- 关键词:
项目摘要
Jackknife empirical likelihood, introduced by professor Bing-Yi Jing and his collaborators in 2009, is a new approach which combines two popular nonparametric approaches: the jackknife and the empirical likelihood. One outstanding advantage of the jackknife empirical likelihood method is that it overcomes the computational difficulty of empirical likelihood when treating with nonlinear statistics, and can largely relieve the computational burden. Based on the jackknife empirical likelihood method, this proposal will study the statistical inference for parameters involved in the nonsmooth estimation functions, transformation parameters and regression coefficients of the Box-Cox transformation model with both complete and censored data, nonparametric and semiparametric ROC surfaces. First, we will develop jackknife empirical log likelihood ratio functions for the unkown parameters, and will show that each of the proposed ratio converges in distribution to a chi-squared distribution under some suitable conditions, and hence it can be used to construct confidence regions for the unknown parameters. Second,to confirm the advantage of our new methods, extensive simulation studies will be conducted to compare with other methods proposed in the literature in terms of accuracy and computational efficiency. Third, we will apply these novel methods to some real data sets. The research is expected to overcome some drawbacks involved in the existing methods such as difficult in estimating the asymptotic covariance matrix,time-consuming,etc. Therefore,the proposed interval estimations and hypothesis testing will be more robust, more accurate and more computationally efficient. The methodologies developed in this project will not only enrich the theory of empirical likelihood, but also greatly extend the scope of the applications of empirical likelihood methods.
刀切经验似然是荆炳义等学者于2009年结合刀切法和经验似然提出的一种非参数统计推断方法。该方法的突出优点是克服了经验似然在处理非线性统计量时计算任务十分繁重的困难。本项目拟采用刀切经验似然方法研究非光滑估计函数中感兴趣的参数,Box-Cox转换模型中的转换参数和回归系数,以及非参数和半参数框架下ROC曲面的统计推断。我们将建立未知参数的刀切经验对数似然比函数,并在适当的条件下,证明所提的比依分布收敛于卡方分布,所得结果可以用来构造未知参数的置信域。同时,本项目将进行模拟研究,通过与已有方法在精度和计算效率方面的比较来展现所提方法的优势。这些新方法也将应用于分析一些实际数据。通过本项目研究,可望克服已有方法存在估计量的协方差矩阵难于估计、计算效率低等不足,进而建立一系列更为稳健、精确和计算上有效的区间估计和检验方法。本项目的研究成果将在理论上丰富经验似然的研究,同时也将拓宽其应用领域。
结项摘要
基于刀切经验似然方法,本项目研究了具有U-统计量结构的非光滑估计函数中感兴趣参数的估计问题,提出了刀切经验似然推断方法;在最小绝对相对误差准则下,构造了线性可乘回归模型中回归系数的经验似然区间估计并将其推广至高维场合;研究了多元正态分布的高维均值向量的收缩估计问题,提出了基于Stein无偏风险估计的新估计;将经验似然方法引入复合泊松过程,构建了该过程均值的经验似然置信区间;给出了线性回归模型中误差项方差的刀切经验似然区间估计;考虑了如何有效的检验高维分布是否相同的问题;构建了基于曲线下面积的生物标志物最佳线性组合的刀切经验似然估计。对以上估计和检验,我们证明了相应的极限性质,进行了大量的模拟研究和实际数据分析。这些方法论不仅丰富和发展了经验似然的理论,也将其应用拓宽至生物医学、金融经济等领域,为这些领域提供了更为精确和计算上有效的方法。
项目成果
期刊论文数量(5)
专著数量(0)
科研奖励数量(0)
会议论文数量(0)
专利数量(0)
Empirical likelihood for least absolute relative error regression
最小绝对相对误差回归的经验似然
- DOI:10.1007/s11749-013-0343-5
- 发表时间:2014-03-01
- 期刊:TEST
- 影响因子:1.3
- 作者:Li, Zhouping;Lin, Yuanyuan;Zhou, Wang
- 通讯作者:Zhou, Wang
On SURE-Type Double Shrinkage Estimation
关于SURE型双收缩率估计
- DOI:--
- 发表时间:2015
- 期刊:Journal of the American Statistical Association
- 影响因子:3.7
- 作者:Jing, Bing-Yi;Li,Zhouping;Pan, Guangming;Zhou, Wang
- 通讯作者:Zhou, Wang
On Nonsmooth Estimating Functions via Jackknife Empirical Likelihood
基于折刀经验似然的非光滑估计函数
- DOI:10.1111/sjos.12164
- 发表时间:2016-03
- 期刊:Scandinavian Journal of Statistics
- 影响因子:1
- 作者:Li, Zhouping;Xu,Jinfeng;Zhou,Wang
- 通讯作者:Zhou,Wang
数据更新时间:{{ journalArticles.updateTime }}
{{
item.title }}
{{ item.translation_title }}
- DOI:{{ item.doi || "--"}}
- 发表时间:{{ item.publish_year || "--" }}
- 期刊:{{ item.journal_name }}
- 影响因子:{{ item.factor || "--"}}
- 作者:{{ item.authors }}
- 通讯作者:{{ item.author }}
数据更新时间:{{ journalArticles.updateTime }}
{{ item.title }}
- 作者:{{ item.authors }}
数据更新时间:{{ monograph.updateTime }}
{{ item.title }}
- 作者:{{ item.authors }}
数据更新时间:{{ sciAawards.updateTime }}
{{ item.title }}
- 作者:{{ item.authors }}
数据更新时间:{{ conferencePapers.updateTime }}
{{ item.title }}
- 作者:{{ item.authors }}
数据更新时间:{{ patent.updateTime }}
其他文献
一种结构类型可调的复杂输运网络演化模型及仿真
- DOI:--
- 发表时间:2014
- 期刊:小型微型计算机系统
- 影响因子:--
- 作者:李周平;杨坚争;韩景倜
- 通讯作者:韩景倜
基于复杂分层网络的城际路网级联失效可靠性仿真
- DOI:--
- 发表时间:2015
- 期刊:计算机应用研究
- 影响因子:--
- 作者:李周平;韩景倜;肖宇
- 通讯作者:肖宇
基于分层复杂网络的城际路网空间结构特征
- DOI:--
- 发表时间:2014
- 期刊:公路交通科技
- 影响因子:--
- 作者:李周平;韩景倜;杨坚争;肖宇
- 通讯作者:肖宇
增维非光滑估计方程的刀切经验似然方法
- DOI:--
- 发表时间:2019
- 期刊:中国科学:数学
- 影响因子:--
- 作者:韦阳;李周平;杨帆
- 通讯作者:杨帆
其他文献
{{
item.title }}
{{ item.translation_title }}
- DOI:{{ item.doi || "--" }}
- 发表时间:{{ item.publish_year || "--"}}
- 期刊:{{ item.journal_name }}
- 影响因子:{{ item.factor || "--" }}
- 作者:{{ item.authors }}
- 通讯作者:{{ item.author }}
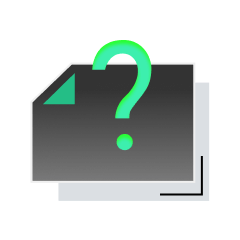
内容获取失败,请点击重试
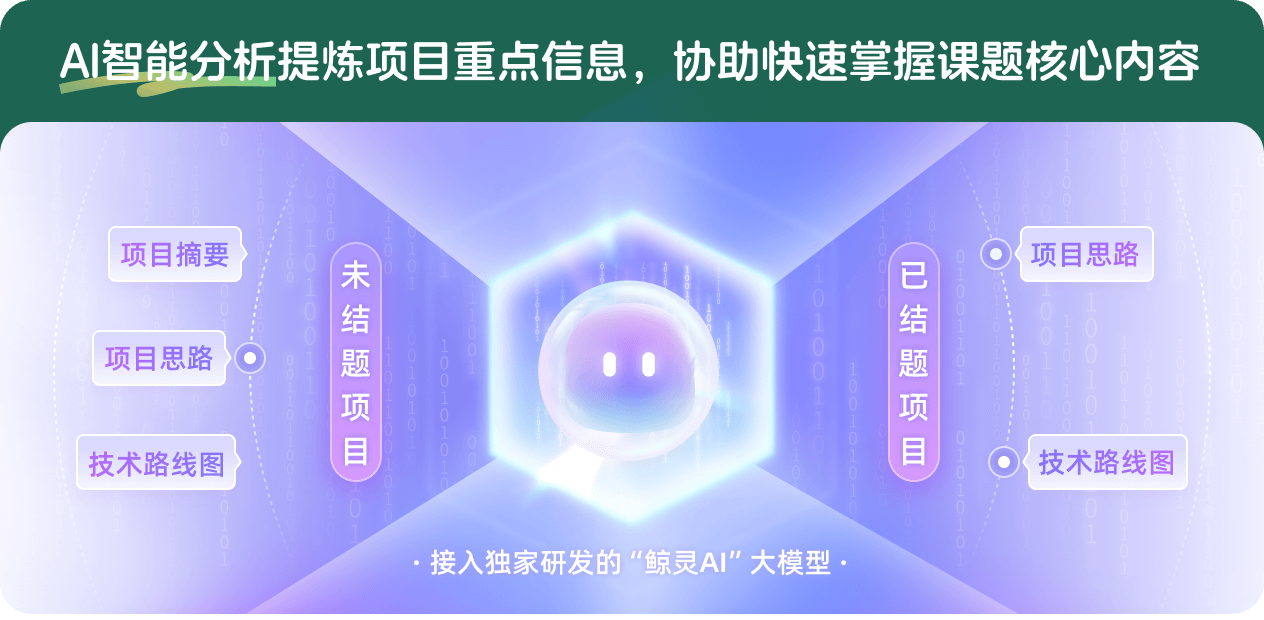
查看分析示例
此项目为已结题,我已根据课题信息分析并撰写以下内容,帮您拓宽课题思路:
AI项目摘要
AI项目思路
AI技术路线图
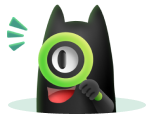
请为本次AI项目解读的内容对您的实用性打分
非常不实用
非常实用
1
2
3
4
5
6
7
8
9
10
您认为此功能如何分析更能满足您的需求,请填写您的反馈:
李周平的其他基金
高维数据分析中的收缩估计与似然推断
- 批准号:11571154
- 批准年份:2015
- 资助金额:50.0 万元
- 项目类别:面上项目
相似国自然基金
{{ item.name }}
- 批准号:{{ item.ratify_no }}
- 批准年份:{{ item.approval_year }}
- 资助金额:{{ item.support_num }}
- 项目类别:{{ item.project_type }}
相似海外基金
{{
item.name }}
{{ item.translate_name }}
- 批准号:{{ item.ratify_no }}
- 财政年份:{{ item.approval_year }}
- 资助金额:{{ item.support_num }}
- 项目类别:{{ item.project_type }}