基于等价非凸Lipschitz模型的低秩优化问题的DC算法研究
项目介绍
AI项目解读
基本信息
- 批准号:11701186
- 项目类别:青年科学基金项目
- 资助金额:20.0万
- 负责人:
- 依托单位:
- 学科分类:A0405.连续优化
- 结题年份:2020
- 批准年份:2017
- 项目状态:已结题
- 起止时间:2018-01-01 至2020-12-31
- 项目参与者:沈力; 张东东;
- 关键词:
项目摘要
Low rank optimization problems have a wide range of applications in a diverse set of fields such as statistics, control and system identification, signal and image processing, machine learning, and so on. In this project, for this class of discontinuous and nonconvex optimization problems, we shall start with their equivalent nonconvex Lipschitz continuous models and conduct the sequential convex relaxation algorithm research for them by constructing effective DC (difference of convex functions) decomposition. The research includes the following several aspects: (1) to construct a class of special nonconvex Lipschitz continuous surrogates for the rank function, establish the equivalent nonconvex Lipschitz continuous forms for low rank matrix optimization problems, and then characterize the properties of stationary points and local optimal solutions of the equivalent Lipschitz continuous models; (2) to propose the DC algorithms by using the effective DC decomposition of the equivalent nonconvex Lipschitz continuous models, characterize the global error bounds for the iterate sequence of the DC algorithms, and analyze the convergence of the error bound sequence in a statistical sense; thereby providing the theoretical guarantees for the proposed sequential convex relaxation algorithms; (3) to design effective algorithms for solving the subproblems involved in the DC algorithms, and analyze their rate of local convergence and global iteration complexity bounds; (4) to write the codes for the proposed DC algorithms, and test their performance via numerical experiments. The research results of this project will not only provide effective computational tools for low-rank matrix optimization problems, but also will enrich the theory of the DC algorithm, thereby having an important theoretical and practical significance.
低秩优化问题在统计、控制与系统辨识、信号和图像处理、机器学习等诸多领域中有着十分广泛而重要的应用。本课题拟从低秩优化问题的等价非凸Lipschitz模型入手,通过构造其有效的DC(凸函数的差)分解来开展此类不连续非凸优化问题的序列凸松弛算法研究。主要研究内容包括:(1)构造秩函数的特殊Lipschitz连续非凸代理,建立低秩优化问题的等价非凸Lipschitz模型,并刻画其稳定点和局部最优解的性质;(2)寻求等价非凸Lipschitz模型的有效DC分解,设计相应的DC算法;分析DC算法点列的全局误差界以及误差界序列在统计意义下的收敛性,为所提出的凸松弛算法提供理论保证;(3) 设计求解凸松弛子问题的有效算法,分析算法的局部收敛率和全局迭代复杂界;(4)编写程序代码并进行数值试验。本课题的研究不仅将为低秩优化问题提供有效的计算方法,还将丰富DC算法的理论,具有重要理论意义和应用价值。
结项摘要
低秩和零模优化问题在统计、控制与系统辨识、信号和图像处理、机器学习等诸多领域中有着十分广泛且重要的应用。在本项目中,从低秩和零模优化问题的等价非凸 Lipschitz 连续模型入手,我们通过等价模型的可分性结构和 DC (凸函数的差) 分解来开展此类不连续非凸优化问题的多阶段凸松弛算法研究。主要取得以下三方面的成果:(1) 建立秩函数和零模函数的变分刻画,由此将低秩和零模优化问题等价的转化为带有广义互补约束的数学规划问题 (MPECs),并证明了将互补约束罚到目标函数上得到的罚问题是精确的,通过消去精确罚模型中的对偶变量,可以得到低秩和零模优化问题的等价非凸 Lipschitz 连续代理模型,从而为此类优化问题的算法设计提供了有效的模型基础;(2) 系统地研究了矩阵秩正则极小化问题及其等价 MPEC 模型、精确罚模型、等价非凸 Lipschitz 连续代理模型的各类稳定点及它们之间的关系,为进一步算法的设计提供了模型和理论指导;(3) 观察到精确罚问题具有很好的可分性结构,通过交替求解精确罚问题,设计了一类求解低秩和零模优化问题的多阶段凸松弛算法,针对低秩矩阵恢复和群稀疏向量恢复问题,在适当的限制特征值条件下建立了算法产生的点列的误差界,并证明了误差界序列在统计意义下是几何收敛的,从而为所提出的多阶段凸松弛算法提供了理论保证,数值结果表明提出的算法是有效的。注意到,我们的算法是基于等价非凸 Lipschitz 连续模型设计的,因而,可以有效地克服现有序列凸松弛算法对非凸连续代理模型逼近原问题程度的依赖。此外,我们还将提出的多阶段凸松弛算法应用到了稀疏分位数回归问题、稀疏 EV (error-in-variables) 回归问题以及低秩矩阵恢复的列稀疏正则分解模型,都取得了很好的理论和数值效果。本项目的研究不仅为低秩和零模优化问题提供了有效的计算模型和方法,而且还丰富了非凸非连续优化算法的理论,具有重要理论意义和应用价值。
项目成果
期刊论文数量(8)
专著数量(0)
科研奖励数量(1)
会议论文数量(0)
专利数量(0)
Error bounds for non-polyhedral convex optimization and applications to linear convergence of FDM and PGM
非多面体凸优化的误差界及其在 FDM 和 PGM 线性收敛中的应用
- DOI:10.1016/j.amc.2019.04.048
- 发表时间:2019-10
- 期刊:Applied Mathematics and Computation
- 影响因子:4
- 作者:Liu Yulan;Bi Shujun
- 通讯作者:Bi Shujun
Two-stage convex relaxation approach to low-rank and sparsity regularized least squares loss
低秩稀疏正则最小二乘损失的两阶段凸松弛方法
- DOI:10.1007/s10898-017-0573-2
- 发表时间:2017-10
- 期刊:Journal of Global Optimization
- 影响因子:1.8
- 作者:Han Le;Bi Shujun;Han L
- 通讯作者:Han L
Isolated calmness of solution mappings and exact recovery conditions for nuclear norm optimization problems
核范数优化问题解映射的孤立平静和精确恢复条件
- DOI:10.1080/02331934.2020.1723584
- 发表时间:2020-02
- 期刊:Optimization
- 影响因子:2.2
- 作者:Liu Yulan;Pan Shaohua;Bi Shujun
- 通讯作者:Bi Shujun
A multi-stage convex relaxation approach to noisy structured low-rank matrix recovery
一种用于噪声结构化低秩矩阵恢复的多阶段凸松弛方法
- DOI:10.1007/s12532-020-00177-4
- 发表时间:2017-03
- 期刊:Mathematical Programming Computation
- 影响因子:6.3
- 作者:Bi Shujun;Pan Shaohua;Sun Defeng
- 通讯作者:Sun Defeng
SEVERAL CLASSES OF STATIONARY POINTS FOR RANK REGULARIZED MINIMIZATION PROBLEMS
秩正则最小化问题的几类驻点
- DOI:10.1137/19m1270987
- 发表时间:2020
- 期刊:SIAM Journal on Optimization
- 影响因子:3.1
- 作者:Liu Yulan;Bi Shujun;Pan Shaohua
- 通讯作者:Pan Shaohua
数据更新时间:{{ journalArticles.updateTime }}
{{
item.title }}
{{ item.translation_title }}
- DOI:{{ item.doi || "--"}}
- 发表时间:{{ item.publish_year || "--" }}
- 期刊:{{ item.journal_name }}
- 影响因子:{{ item.factor || "--"}}
- 作者:{{ item.authors }}
- 通讯作者:{{ item.author }}
数据更新时间:{{ journalArticles.updateTime }}
{{ item.title }}
- 作者:{{ item.authors }}
数据更新时间:{{ monograph.updateTime }}
{{ item.title }}
- 作者:{{ item.authors }}
数据更新时间:{{ sciAawards.updateTime }}
{{ item.title }}
- 作者:{{ item.authors }}
数据更新时间:{{ conferencePapers.updateTime }}
{{ item.title }}
- 作者:{{ item.authors }}
数据更新时间:{{ patent.updateTime }}
其他文献
其他文献
{{
item.title }}
{{ item.translation_title }}
- DOI:{{ item.doi || "--" }}
- 发表时间:{{ item.publish_year || "--"}}
- 期刊:{{ item.journal_name }}
- 影响因子:{{ item.factor || "--" }}
- 作者:{{ item.authors }}
- 通讯作者:{{ item.author }}
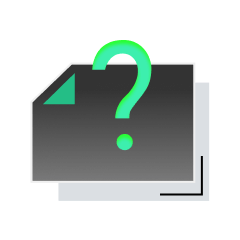
内容获取失败,请点击重试
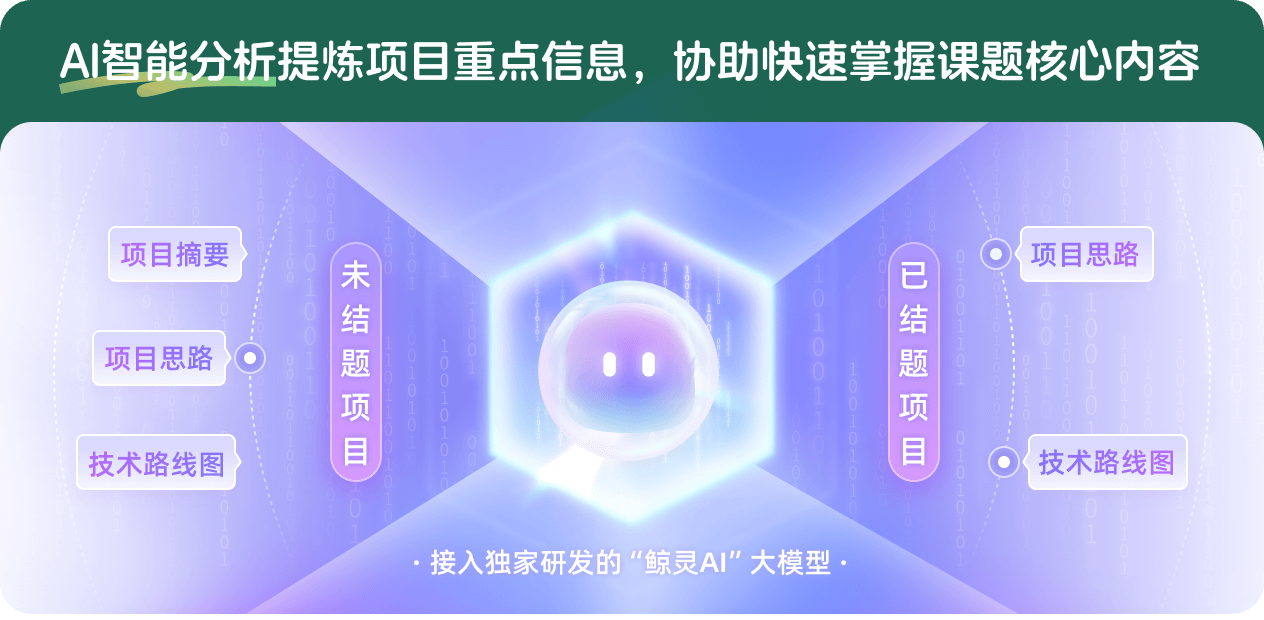
查看分析示例
此项目为已结题,我已根据课题信息分析并撰写以下内容,帮您拓宽课题思路:
AI项目摘要
AI项目思路
AI技术路线图
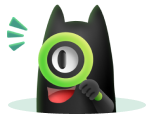
请为本次AI项目解读的内容对您的实用性打分
非常不实用
非常实用
1
2
3
4
5
6
7
8
9
10
您认为此功能如何分析更能满足您的需求,请填写您的反馈:
贲树军的其他基金
稀疏和低秩优化的非精确恢复理论及迭代加权算法研究
- 批准号:12371323
- 批准年份:2023
- 资助金额:43.5 万元
- 项目类别:面上项目
相似国自然基金
{{ item.name }}
- 批准号:{{ item.ratify_no }}
- 批准年份:{{ item.approval_year }}
- 资助金额:{{ item.support_num }}
- 项目类别:{{ item.project_type }}
相似海外基金
{{
item.name }}
{{ item.translate_name }}
- 批准号:{{ item.ratify_no }}
- 财政年份:{{ item.approval_year }}
- 资助金额:{{ item.support_num }}
- 项目类别:{{ item.project_type }}