铁路沿线风速短时智能预测模型及其大数据嵌入方法研究
项目介绍
AI项目解读
基本信息
- 批准号:61873283
- 项目类别:面上项目
- 资助金额:63.0万
- 负责人:
- 依托单位:
- 学科分类:F0303.系统建模理论与仿真技术
- 结题年份:2022
- 批准年份:2018
- 项目状态:已结题
- 起止时间:2019-01-01 至2022-12-31
- 项目参与者:熊小慧; 李燕飞; 陆海博; 米希伟; 王孝楠; 段超; 黄家豪; 杨宇翔; 金楷荣;
- 关键词:
项目摘要
Strong wind is one of the main natural disasters that cause the railway accidents. Strong wind along the railway makes the vibrating phenomenon of the running trains. It leads to the obvious increasing of the derailment and over-turning accidents, which endangers the safety of railways seriously. Since the wind speed along the railway shows strong random and nonlinear characteristics, it is still an unsolved scientific problem to describe the space-time changing law of the wind speed signals accurately and to realize the wind speed high-precision multi-step forecasting. This project will adopt the hybrid researching methods include the spot investigation, modeling calculation, embedded development and experimental evaluation to carry out the study on the intelligent railway short-time wind speed time series forecasting models and their big data embedding method. The main contents of the project include: ①Studying on the decomposition & reconstruction, reduction and clustering calculation of the non-stationary wind speed time series from the independent wind measurement stations along the railway, the changing law of the wind speed time series from the single point and the building of intelligent wind speed forecasting models for each independent wind measurement station; ②Studying on the spatial correlation identification of the wind speed time series from the associated wind measurement stations along the railway and the construction of association zones for these associated stations, the spatial correlation characteristics of the multi-point wind speed time series and the building of the spatial depth wind speed forecasting models for these associated wind measuring stations; ③Development of the software modules for the built wind speed forecasting models in the big data embedding environment based on the proposed forecasting algorithms. Evaluation of the built wind speed forecasting models and their related software computing modules. The research results will provide important theoretical and technical support for the development of the railway safety protecting framework under the strong wind environment.
强风是造成铁路行车事故的主要自然灾害之一。铁路沿线强风引发车体剧烈颤动,脱轨和翻车概率徒增,严重危及行车安全。铁路沿线风速呈现强随机非线性特性,对它实现时空演变规律准确描述和高精度多步预测仍是未解决的科学难题。本项目拟采用现场调研、建模计算、嵌入开发与实验评估相结合的手段,开展铁路沿线风速短时智能预测模型及其大数据嵌入方法研究,主要内容包括:①开展铁路沿线独立测风站非平稳风速时序的分解重构、降维和聚类计算,揭示铁路沿线单点风速时序的时间演变规律,建立各独立测风站的风速时间智能预测模型;②开展铁路沿线关联测风站的风速空间相关性辨识及关联单元构建,探明铁路沿线多点风速时序的空间关联特性,建立关联测风站的风速空间深度预测模型;③基于预测算法成果,开发大数据环境下风速预测模型嵌入软件模块。对预测模型和软件模块开展性能评估。研究结果为构建大风环境铁路行车安全保障体系提供理论基础和技术支撑。
结项摘要
铁路沿线风速预测是保障大风环境列车行车安全的重要措施之一。本项目聚焦铁路沿线风速高质量预测,取得如下成果:.(1)开展了铁路沿线风速特征预处理研究,使用二次分解算法精细划分了风速时序波动特征,筛选了风速显著特征,完成了风速聚类计算;.(2)开展了铁路沿线独立测风站风速混合智能预测研究,提出了融合多小波分析的多目标集成风速预测算法,实现了混合Boost提升算法与后处理滤波的风速大步长预测,建立了“修正-检验-描述”闭环的风速不确定性预测模型;.(3)开展了铁路沿线关联测风站风速混合预测研究,构建了融合“时域滤波-空间筛选-集成预测”多测风站混合风速预测模型,完成了WRF风速空间预测计算,并提出了面向WRF后处理的深度降尺度神经网络;.(4)开展了铁路沿线风速大数据预测研究,建立了风速强化学习与高分辨率大数据预测模型库,开发了风速Spark大数据预测软件模块DCPELM。.项目出版Elsevier、Springer等专著3部,其中1部获斯普林格·自然“中国新发展奖”;发表SCI论文46篇,其中ESI 1%高被引论文2篇;授权日本/俄罗斯/澳大利亚发明专利5项、中国发明专利4项;获法国巴黎国际发明展览会专利金奖2项、国家发明展览会专利金奖1项;培养博士/硕士研究生6名,博士后1名。
项目成果
期刊论文数量(46)
专著数量(3)
科研奖励数量(4)
会议论文数量(0)
专利数量(9)
A vanishing moment ensemble model for wind speed multi-step prediction with multi-objective base model selection
多目标基础模型选择的风速多步预测消失矩集合模型
- DOI:10.1016/j.apenergy.2019.114367
- 发表时间:2020-03
- 期刊:Applied Energy
- 影响因子:11.2
- 作者:刘辉;段铸
- 通讯作者:段铸
Data processing strategies in wind energy forecasting models and applications: A comprehensive review
风能预测模型和应用中的数据处理策略:综合综述
- DOI:10.1016/j.apenergy.2019.04.188
- 发表时间:2019-09-01
- 期刊:APPLIED ENERGY
- 影响因子:11.2
- 作者:Liu, Hui;Chen, Chao
- 通讯作者:Chen, Chao
An improved non-intrusive load disaggregation algorithm and its application
一种改进的非侵入式负载分解算法及其应用
- DOI:10.1016/j.scs.2019.101918
- 发表时间:2020-02-01
- 期刊:SUSTAINABLE CITIES AND SOCIETY
- 影响因子:11.7
- 作者:Liu, Hui;Yu, Chengming;Wang, Ziqi
- 通讯作者:Wang, Ziqi
Spatial air quality index prediction model based on decomposition, adaptive boosting, and three-stage feature selection: A case study in China
基于分解、自适应提升和三阶段特征选择的空间空气质量指数预测模型:以中国为例
- DOI:10.1016/j.jclepro.2020.121777
- 发表时间:2020-08-20
- 期刊:JOURNAL OF CLEANER PRODUCTION
- 影响因子:11.1
- 作者:Liu, Hui;Chen, Chao
- 通讯作者:Chen, Chao
Dynamic ensemble wind speed prediction model based on hybrid deep reinforcement learning
基于混合深度强化学习的动态集合风速预测模型
- DOI:10.1016/j.aei.2021.101290
- 发表时间:2021-04-10
- 期刊:ADVANCED ENGINEERING INFORMATICS
- 影响因子:8.8
- 作者:Chen, Chao;Liu, Hui
- 通讯作者:Liu, Hui
数据更新时间:{{ journalArticles.updateTime }}
{{
item.title }}
{{ item.translation_title }}
- DOI:{{ item.doi || "--"}}
- 发表时间:{{ item.publish_year || "--" }}
- 期刊:{{ item.journal_name }}
- 影响因子:{{ item.factor || "--"}}
- 作者:{{ item.authors }}
- 通讯作者:{{ item.author }}
数据更新时间:{{ journalArticles.updateTime }}
{{ item.title }}
- 作者:{{ item.authors }}
数据更新时间:{{ monograph.updateTime }}
{{ item.title }}
- 作者:{{ item.authors }}
数据更新时间:{{ sciAawards.updateTime }}
{{ item.title }}
- 作者:{{ item.authors }}
数据更新时间:{{ conferencePapers.updateTime }}
{{ item.title }}
- 作者:{{ item.authors }}
数据更新时间:{{ patent.updateTime }}
其他文献
Simulation study of the influence of leak electrons on the discharge characteristics of a cusped field thruster
漏电子对尖点场推进器放电特性影响的仿真研究
- DOI:10.1088/2058-6272/aaf674
- 发表时间:2019-01
- 期刊:Plasma Science and Technology
- 影响因子:1.7
- 作者:刘辉;牛翔;伍环;于达仁
- 通讯作者:于达仁
太阳局部高分辨观测像的日球坐标自动标定
- DOI:10.1360/n972019-00092
- 发表时间:2019
- 期刊:科学通报
- 影响因子:--
- 作者:季凯帆;刘辉;金振宇;尚振宏;强振平
- 通讯作者:强振平
基于VSC的双馈风电场群宽频带次同步谐振抑制作用分析与实验
- DOI:10.16081/j.epae.201909043
- 发表时间:2019
- 期刊:电力自动化设备
- 影响因子:--
- 作者:廖坤玉;肖湘宁;罗超;陶顺;杨志超;于弘洋;刘宗烨;刘辉
- 通讯作者:刘辉
基于多元回归分析的泥水盾构施工参数分析
- DOI:10.16670/j.cnki.cn11-5823/tu.2016.03.18
- 发表时间:2016
- 期刊:土木建筑工程信息技术
- 影响因子:--
- 作者:廖利钊;陶冶;刘辉
- 通讯作者:刘辉
跨界协同创新收益分配
- DOI:10.3969/j.issn.1005-2542.2020.02.012
- 发表时间:2020
- 期刊:系统管理学报
- 影响因子:--
- 作者:刘辉;沈焱;李仕明;王晓明
- 通讯作者:王晓明
其他文献
{{
item.title }}
{{ item.translation_title }}
- DOI:{{ item.doi || "--" }}
- 发表时间:{{ item.publish_year || "--"}}
- 期刊:{{ item.journal_name }}
- 影响因子:{{ item.factor || "--" }}
- 作者:{{ item.authors }}
- 通讯作者:{{ item.author }}
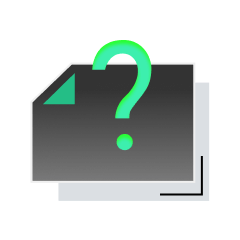
内容获取失败,请点击重试
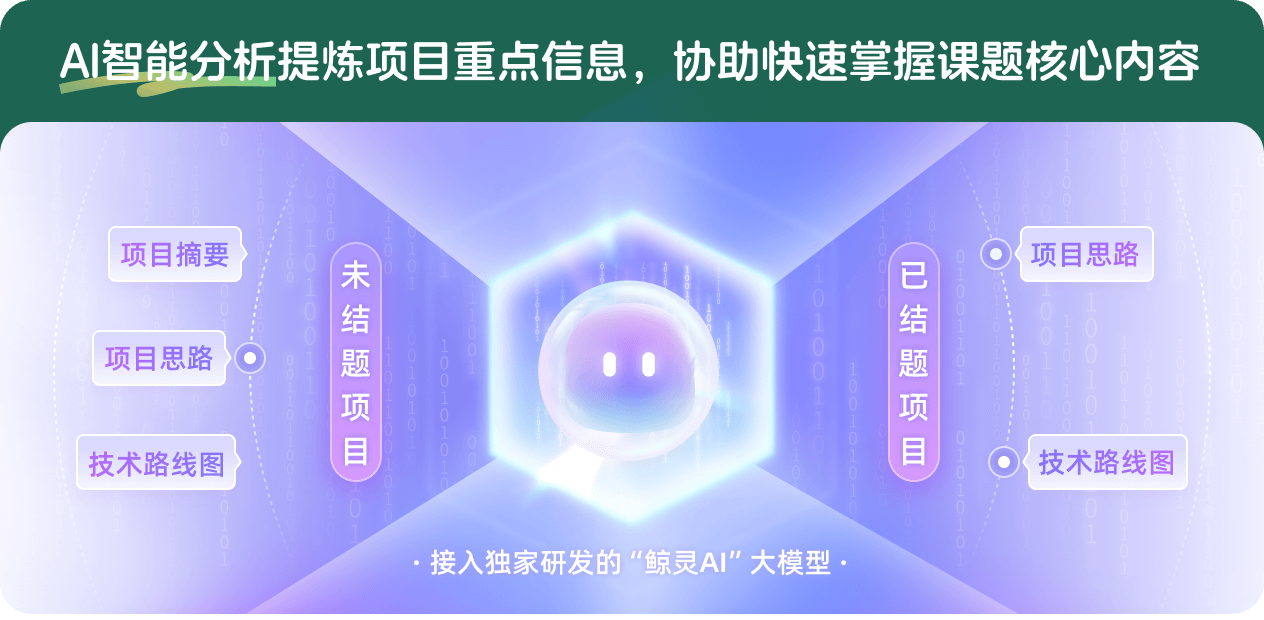
查看分析示例
此项目为已结题,我已根据课题信息分析并撰写以下内容,帮您拓宽课题思路:
AI项目摘要
AI项目思路
AI技术路线图
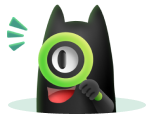
请为本次AI项目解读的内容对您的实用性打分
非常不实用
非常实用
1
2
3
4
5
6
7
8
9
10
您认为此功能如何分析更能满足您的需求,请填写您的反馈:
刘辉的其他基金
地铁车站室内环境PM2.5浓度扩散模拟及其多点映射实验研究
- 批准号:5211101257
- 批准年份:2021
- 资助金额:0.00 万元
- 项目类别:国际(地区)合作与交流项目
地铁车站室内环境PM2.5浓度扩散模拟及其多点映射实验研究
- 批准号:52111530232
- 批准年份:2021
- 资助金额:3 万元
- 项目类别:国际(地区)合作与交流项目
地铁站台候车区PM2.5浓度短时多步预测及其空间关联规律研究
- 批准号:52072412
- 批准年份:2020
- 资助金额:58 万元
- 项目类别:面上项目
基于ATRP的双重环境敏感性聚合物刷的构筑及响应行为研究
- 批准号:21376271
- 批准年份:2013
- 资助金额:80.0 万元
- 项目类别:面上项目
铁路沿线风速超前多步高精度预测方法研究
- 批准号:51308553
- 批准年份:2013
- 资助金额:25.0 万元
- 项目类别:青年科学基金项目
相似国自然基金
{{ item.name }}
- 批准号:{{ item.ratify_no }}
- 批准年份:{{ item.approval_year }}
- 资助金额:{{ item.support_num }}
- 项目类别:{{ item.project_type }}
相似海外基金
{{
item.name }}
{{ item.translate_name }}
- 批准号:{{ item.ratify_no }}
- 财政年份:{{ item.approval_year }}
- 资助金额:{{ item.support_num }}
- 项目类别:{{ item.project_type }}