中英文混合语音识别中声学建模关键技术研究
项目介绍
AI项目解读
基本信息
- 批准号:61701306
- 项目类别:青年科学基金项目
- 资助金额:25.0万
- 负责人:
- 依托单位:
- 学科分类:F0117.多媒体信息处理
- 结题年份:2020
- 批准年份:2017
- 项目状态:已结题
- 起止时间:2018-01-01 至2020-12-31
- 项目参与者:赵勤; 刘玉娟; 关卫国; 魏浩然; 张艳; 冯志民;
- 关键词:
项目摘要
With rapid advances of intelligence speech technologies and the growing international trend of our communication, techniques to obtain excellent Mandarin-English code-mixing speech recognition performance are highly desired. However, conventional related research usually focuses on the inter-sentence bilingual speech recognition cases, and this makes the technology difficult to meet the needs of most users today. In this proposal, we focus on the acoustic modeling technologies for Mandarin-English mix-lingual speech recognition with intra-sentence code-mixing. More specifically, the mix-lingual acoustic modeling techniques based on the deep neural networks will be investigated. Our solution involves three key techniques, including: (1) the data augmentation techniques are proposed to enhance the acoustic environments and to increase the amount of the Mandarin-English code-mixed speech data; (2) the proper mix-lingual lexicon construction and correction approaches are introduced to eliminate the negative effects from the differences between the Mandarin and English phonemes. New approaches are originated from the combination of feedback information of the speech recognition errors, the knowledge of linguistics and phonetics, and the conventional data driven algorithms for phone mapping; (3) the knowledge transfer learning methods are investigated for transferring the linguistic and non-native accent nature of the embedded English speech data. New algorithms for deep feature representation and mix-lingual acoustic model adaptation will be carefully designed. Finally, we will combine all of the key techniques to build a Mandarin-English mix-lingual speech recognition demonstration system to validate our proposed techniques. Furthermore, we plan to cooperate with some relevant enterprises to promote the application values of our Mandarin-English code-mixing speech recognition system.
随着智能语音技术的快速发展以及人们沟通交流的国际化趋势日益加剧,高性能的中英文混合语音识别技术成为迫切需求。然而,传统的中英文语音识别大多注重于研究中英文句间切换的情况,这使得其难以满足当今大多数用户的需求。本项目致力于研究中英文在句内混用的语音识别混合声学建模技术,建立统一的技术框架,针对其中的关键科学问题展开深入探讨。具体内容包括:对中英文混合语音数据量及其声学环境进行增强,提高声学模型深度学习统计建模的准确性和鲁棒性;提出结合语音识别错误中的反馈信息、语音学知识和已有的数据驱动算法,构建更合理的中英文混合发音词典,消除中英文音素差异带来的负面影响;从深度特征表达和声学模型自适应两方面实现非母语英语口音特性和英语语言属性的知识迁移学习,提高混合声学模型对英文发音变异的鲁棒性;搭建一套中英文混合语音识别示范系统,并与相关企业合作进行成果推广及应用。
结项摘要
中英文混合语音识别是指待识别的语音中同时包含中文普通话和英语的语音识别。随着智能语音技术的快速发展以及人们沟通交流国际化趋势日益加剧,高性能的中英文混合语音识别技术成为迫切需求。本项目着重研究了在以中文普通话为主体语中嵌入英语的自动语音识别声学建模中的关键科学问题。主要内容包括:(1)借鉴半监督和主动学习思想,提出了基于异构深度神经网络架构的自动标注算法,实现了大规模多领域的混合语音片段自动标注,对中英文混合语音数据量及其声学环境进行了增强,提高了声学模型深度学习统计建模的准确性和鲁棒性;(2)提出了一种基于声学数据驱动的混合语言发音词典修正方法,同时结合语音识别错误中的反馈信息、语音学知识,构建更合理的中英混合发音词典,在一定程度上解决了非母语英文口音发音变异问题,消除了中英文音素差异带来的负面影响;(3)提出了与语言相关的深度表征抽取方法和基于预训练的端到端混合注意力机制的声学建模方法,大幅度提升了整体语音识别系统的声学建模能力,提高了现有混合语音识别系统的性能; (4)积极与工业界进行产学研合作,阶段性研究成果及时应用到工业界相关产品中,取得了较好社会价值和经济效益,并在此基础上成立校企联合实验室,为智能语音领域协同培养高质量技术人才。综上,本项目的研究方法和技术对混合语音识别的研究起到重要的推动作用,为构建大规模中英文混合连续语音识别应用引擎提供了理论支撑和实践经验。
项目成果
期刊论文数量(6)
专著数量(0)
科研奖励数量(2)
会议论文数量(4)
专利数量(0)
Short-time speaker verification with different speaking style utterances.
不同说话风格话语的短时说话人验证
- DOI:10.1371/journal.pone.0241809
- 发表时间:2020
- 期刊:PloS one
- 影响因子:3.7
- 作者:Mao H;Shi Y;Liu Y;Wei L;Li Y;Long Y
- 通讯作者:Long Y
Mask-based blind source separation and MVDR beamforming in ASR
ASR 中基于掩模的盲源分离和 MVDR 波束形成
- DOI:10.1007/s10772-019-09666-x
- 发表时间:2019-12
- 期刊:International Journal of Speech Technology
- 影响因子:--
- 作者:Renke He;Yanhua Long;Yijie Li;Jiaen Liang
- 通讯作者:Jiaen Liang
Addressing Text-Dependent Speaker Verification Using Singing Speech
使用歌唱语音解决文本相关的说话人验证问题
- DOI:10.3390/app9132636
- 发表时间:2019-06
- 期刊:APPLIED SCIENCES-BASEL
- 影响因子:2.7
- 作者:Yan Shi;Juanjuan Zhou;Yanhua Long;Yijie Li;Hongwei Mao
- 通讯作者:Hongwei Mao
Offline to online speaker adaptation for real-time deep neural network based LVCSR systems
基于 LVCSR 系统的实时深度神经网络的离线到在线扬声器自适应
- DOI:10.1007/s11042-018-6041-2
- 发表时间:2018-11
- 期刊:Multimedia Tools and Applications
- 影响因子:3.6
- 作者:Yanhua Long;Yijie Li;Bo Zhang
- 通讯作者:Bo Zhang
Large-Scale Semi-Supervised Training in Deep Learning Acoustic Model for ASR
ASR 深度学习声学模型的大规模半监督训练
- DOI:10.1109/access.2019.2940961
- 发表时间:2019-09
- 期刊:IEEE Access
- 影响因子:3.9
- 作者:Yanhua Long;Yijie Li;Shuang Wei;Qiaozheng Zhang;Chunxia Yang
- 通讯作者:Chunxia Yang
数据更新时间:{{ journalArticles.updateTime }}
{{
item.title }}
{{ item.translation_title }}
- DOI:{{ item.doi || "--"}}
- 发表时间:{{ item.publish_year || "--" }}
- 期刊:{{ item.journal_name }}
- 影响因子:{{ item.factor || "--"}}
- 作者:{{ item.authors }}
- 通讯作者:{{ item.author }}
数据更新时间:{{ journalArticles.updateTime }}
{{ item.title }}
- 作者:{{ item.authors }}
数据更新时间:{{ monograph.updateTime }}
{{ item.title }}
- 作者:{{ item.authors }}
数据更新时间:{{ sciAawards.updateTime }}
{{ item.title }}
- 作者:{{ item.authors }}
数据更新时间:{{ conferencePapers.updateTime }}
{{ item.title }}
- 作者:{{ item.authors }}
数据更新时间:{{ patent.updateTime }}
其他文献
采用韵律特征的说话人确认系统
- DOI:--
- 发表时间:--
- 期刊:数据采集与处理
- 影响因子:--
- 作者:龙艳花;郭武;戴礼荣
- 通讯作者:戴礼荣
其他文献
{{
item.title }}
{{ item.translation_title }}
- DOI:{{ item.doi || "--" }}
- 发表时间:{{ item.publish_year || "--"}}
- 期刊:{{ item.journal_name }}
- 影响因子:{{ item.factor || "--" }}
- 作者:{{ item.authors }}
- 通讯作者:{{ item.author }}
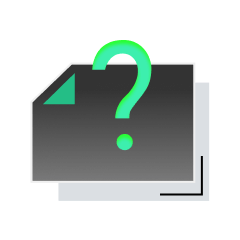
内容获取失败,请点击重试
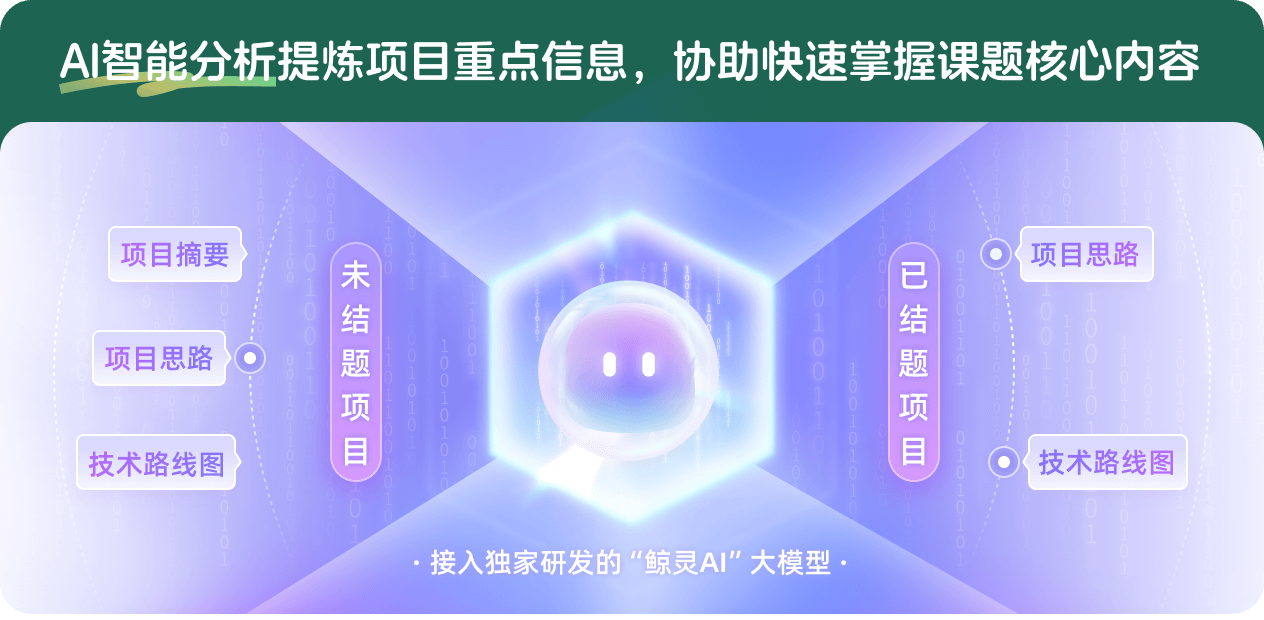
查看分析示例
此项目为已结题,我已根据课题信息分析并撰写以下内容,帮您拓宽课题思路:
AI项目摘要
AI项目思路
AI技术路线图
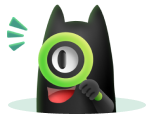
请为本次AI项目解读的内容对您的实用性打分
非常不实用
非常实用
1
2
3
4
5
6
7
8
9
10
您认为此功能如何分析更能满足您的需求,请填写您的反馈:
龙艳花的其他基金
低资源条件下的重口音鲁棒性声学建模关键技术研究
- 批准号:62071302
- 批准年份:2020
- 资助金额:54 万元
- 项目类别:面上项目
相似国自然基金
{{ item.name }}
- 批准号:{{ item.ratify_no }}
- 批准年份:{{ item.approval_year }}
- 资助金额:{{ item.support_num }}
- 项目类别:{{ item.project_type }}
相似海外基金
{{
item.name }}
{{ item.translate_name }}
- 批准号:{{ item.ratify_no }}
- 财政年份:{{ item.approval_year }}
- 资助金额:{{ item.support_num }}
- 项目类别:{{ item.project_type }}