不完全信息环境下指挥控制组织结构识别与发现方法
项目介绍
AI项目解读
基本信息
- 批准号:71701205
- 项目类别:青年科学基金项目
- 资助金额:17.0万
- 负责人:
- 依托单位:
- 学科分类:G0112.信息系统与管理
- 结题年份:2020
- 批准年份:2017
- 项目状态:已结题
- 起止时间:2018-01-01 至2020-12-31
- 项目参与者:刘忠; 王涛; 周鋆; 成清; 张家铭; 杨婷婷; 范长俊; 梁星星;
- 关键词:
项目摘要
Nowadays, the Command and Control (C2) organization idetection and detection is one of the most important issues on social networks. The theory analysis about how the organization forms has great value in theory and practice. Especially, the military or terrorist organization which are more antagonistic, can not be observed in time and completely. Therefore they are always under incomplete information and asymmetric environment. The detecting their organization structure rapidly and effecively plays a crucial role in the network-centric warfare. There are a huge number of studies on Command Control information networks, such as gravity analysis of C2 networks, link prediction on C2 relationships and pattern recognition on role and function of the elements in C2 networks. However, it’s hardly to get the complete and unencrypted information so that the many studies can’t be used to predict missing links and analysis on the C2 networks in practice. In this project, the imcomplete information, such as encrypted messages, are studied to reconstruct the C2 networks. The link prediction methods are proposed to identify the missing and false links by the message's basic information itself, such as length of message, sending and receiving addresses, delay and transfer protocols, etc. Based the topology of the C2 network restored by link prediction, we will explore the role recognition for hierarchy network and the structure mining. By this way , the architecture and C2 relationships of enemy can be analyzed for battlefield awareness.
指挥控制组织结构的识别与发现是社会网络研究领域的热点,不仅具有巨大的理论价值而且在实际有着广泛的应用,特别是针对诸如恐怖组织、军事行动等对抗性强的组织,快速准确的指挥控制结构发现是遏制犯罪、保障国家安全的重要环节。然而,这些网络与传统的社会网络不同,他们在对抗过程中往往刻意隐蔽自己的组织结构、传输信息等,使得其具有明显的信息不完备性、部分可观测性及非对称对抗等特点,导致结构识别和发现存在困难。本课题针对强对抗网络,研究不完全信息下的指控网络结构特征,研究结构还原和小组织发现等基础算法,为指控网络实时分析提供理论支撑。
结项摘要
本课题聚焦如何利用指控网络中不完备、非对称数据,实现军事作战组织、恐怖组织等强对抗网络的拓扑还原与关键节点预测,探索指控组织宏观整体效能与指控实体微观变化之间的本质关联与作用规律,开展了链路预测、虚假信息过滤及角色识别问题,主要研究创新点包括四个方面。.(1)针对层级指控网络内部不同类型节点的关联特征,以及指控网络与使命任务的关系,研究层级指控网络的表示,提出了一种基于使命任务的层级指控网络演化模型,便于进行边预测及角色分组;.(2)根据网络中传输的不完整信息,针对层次结构模型提出了一种拓扑还原算法,引入边聚类算法中的分割密度指标,实现链路预测还原程度的度量;.(3)针对大量冗余或虚假信息,基于随机分块理论构建了过滤层级指控网络中虚假边的模型,提出了一种平衡稳定的MH抽样算法实现模型求解;.(4)针对还原后的层级指控网络,依据各类指挥控制节点的业务特点,基于分层最小支配集提出了一种边聚类算法,实现指挥控制节点角色的识别,支撑对层级指控网络的拓扑重构。
项目成果
期刊论文数量(31)
专著数量(0)
科研奖励数量(3)
会议论文数量(6)
专利数量(1)
Artificial Neural Networks for Chemistry Representation in Numerical Simulation of the Flamelet-Based Models for Turbulent Combustion
基于小火焰的湍流燃烧模型数值模拟中化学表示的人工神经网络
- DOI:10.1109/access.2020.2990943
- 发表时间:2020
- 期刊:IEEE Access
- 影响因子:3.9
- 作者:Zhang Jiarui;Huang Honglan;Xia Zhixun;Ma Likun;Duan Yifan;Feng Yanghe;Huang Jincai
- 通讯作者:Huang Jincai
Active one-shot learning by a deep Q-network strategy
通过深度 Q 网络策略进行主动一次性学习
- DOI:10.1016/j.neucom.2019.11.017
- 发表时间:2020-03
- 期刊:Neurocomputing
- 影响因子:6
- 作者:Chen Li;Huang Honglan;Feng Yanghe;Cheng Guangquan;Huang Jincai;Liu Zhong
- 通讯作者:Liu Zhong
CSAN: A neural network benchmark model for crime forecasting in spatio-temporal scale
CSAN:时空尺度犯罪预测的神经网络基准模型
- DOI:10.1016/j.knosys.2019.105120
- 发表时间:2020-02
- 期刊:Knowledge-Based Systems
- 影响因子:8.8
- 作者:Wang Qi;Jin Guangyin;Zhao Xia;Feng Yanghe;Huang Jincai
- 通讯作者:Huang Jincai
Construction and confirmatory factor analysis of the core cognitive ability index system of ship C2 system operators
船舶C2系统操作人员核心认知能力指标体系构建及验证性因素分析
- DOI:10.1371/journal.pone.0237339
- 发表时间:2020-08-24
- 期刊:PLOS ONE
- 影响因子:3.7
- 作者:Li, Ning;Huang, Jincai;Feng, Yanghe
- 通讯作者:Feng, Yanghe
An unsupervised ensemble framework for node anomaly behavior detection in social network
社交网络中节点异常行为检测的无监督集成框架
- DOI:10.1007/s00500-019-04547-6
- 发表时间:2020
- 期刊:Soft Computing
- 影响因子:4.1
- 作者:Cheng Qing;Zhou Yun;Feng Yanghe;Liu Zhong
- 通讯作者:Liu Zhong
数据更新时间:{{ journalArticles.updateTime }}
{{
item.title }}
{{ item.translation_title }}
- DOI:{{ item.doi || "--"}}
- 发表时间:{{ item.publish_year || "--" }}
- 期刊:{{ item.journal_name }}
- 影响因子:{{ item.factor || "--"}}
- 作者:{{ item.authors }}
- 通讯作者:{{ item.author }}
数据更新时间:{{ journalArticles.updateTime }}
{{ item.title }}
- 作者:{{ item.authors }}
数据更新时间:{{ monograph.updateTime }}
{{ item.title }}
- 作者:{{ item.authors }}
数据更新时间:{{ sciAawards.updateTime }}
{{ item.title }}
- 作者:{{ item.authors }}
数据更新时间:{{ conferencePapers.updateTime }}
{{ item.title }}
- 作者:{{ item.authors }}
数据更新时间:{{ patent.updateTime }}
其他文献
基于能力素质模型的联合作战保障人才培养目标设计方法研究
- DOI:--
- 发表时间:2018
- 期刊:高等教育研究学报
- 影响因子:--
- 作者:王涛;冯旸赫;舒宇;吕济民;刘嘉敏
- 通讯作者:刘嘉敏
面向多旅行商问题的多目标模拟退火算法研究
- DOI:--
- 发表时间:2017
- 期刊:南京师大学报(自然科学版)
- 影响因子:--
- 作者:梁星星;马扬;冯旸赫
- 通讯作者:冯旸赫
其他文献
{{
item.title }}
{{ item.translation_title }}
- DOI:{{ item.doi || "--" }}
- 发表时间:{{ item.publish_year || "--"}}
- 期刊:{{ item.journal_name }}
- 影响因子:{{ item.factor || "--" }}
- 作者:{{ item.authors }}
- 通讯作者:{{ item.author }}
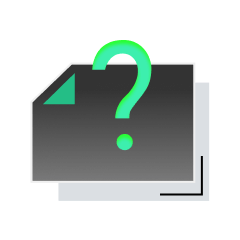
内容获取失败,请点击重试
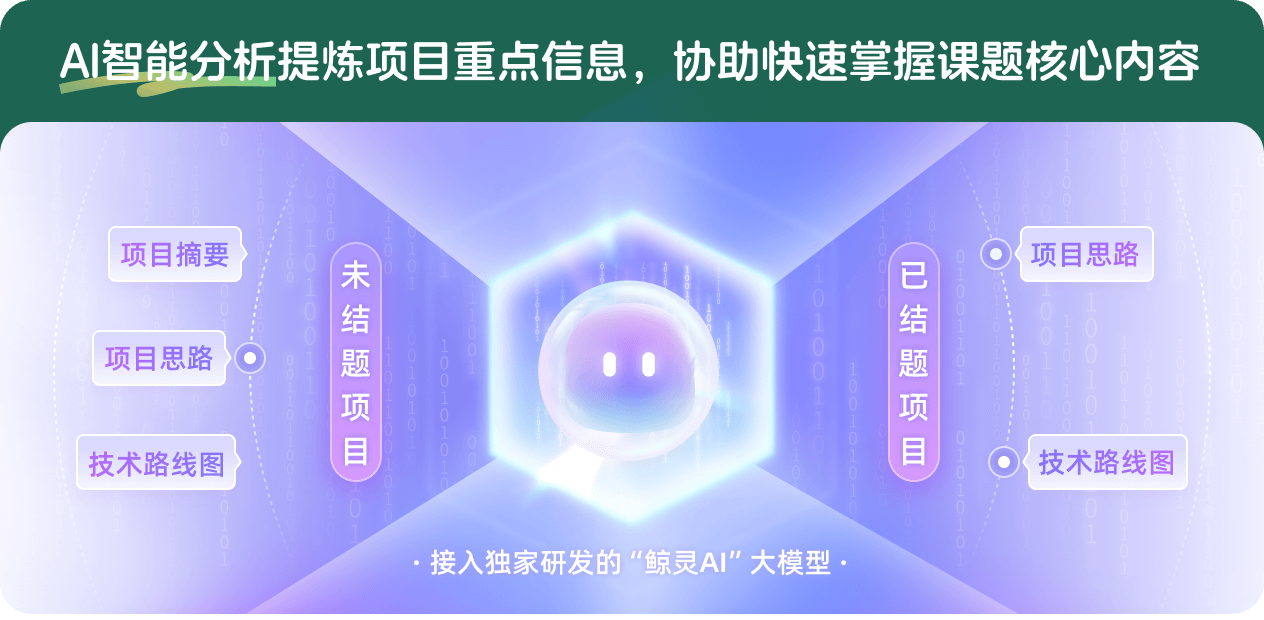
查看分析示例
此项目为已结题,我已根据课题信息分析并撰写以下内容,帮您拓宽课题思路:
AI项目摘要
AI项目思路
AI技术路线图
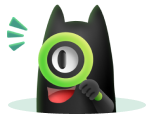
请为本次AI项目解读的内容对您的实用性打分
非常不实用
非常实用
1
2
3
4
5
6
7
8
9
10
您认为此功能如何分析更能满足您的需求,请填写您的反馈:
冯旸赫的其他基金
复杂对抗环境中先验知识与强化学习结合的智能规划方法
- 批准号:62273352
- 批准年份:2022
- 资助金额:52.00 万元
- 项目类别:面上项目
复杂对抗环境中先验知识与强化学习结合的智能规划方法
- 批准号:
- 批准年份:2022
- 资助金额:51 万元
- 项目类别:面上项目
相似国自然基金
{{ item.name }}
- 批准号:{{ item.ratify_no }}
- 批准年份:{{ item.approval_year }}
- 资助金额:{{ item.support_num }}
- 项目类别:{{ item.project_type }}
相似海外基金
{{
item.name }}
{{ item.translate_name }}
- 批准号:{{ item.ratify_no }}
- 财政年份:{{ item.approval_year }}
- 资助金额:{{ item.support_num }}
- 项目类别:{{ item.project_type }}