复杂网络拓扑与参数的辨识
项目介绍
AI项目解读
基本信息
- 批准号:61573205
- 项目类别:面上项目
- 资助金额:65.0万
- 负责人:
- 依托单位:
- 学科分类:F0303.系统建模理论与仿真技术
- 结题年份:2019
- 批准年份:2015
- 项目状态:已结题
- 起止时间:2016-01-01 至2019-12-31
- 项目参与者:张彩虹; 张震; 刘华波; 高艳普; 袁金云; 王莹莹; 温超; 刘叶;
- 关键词:
项目摘要
A complex network is an abstraction of a complex dynamic system by representing its subsystems as nodes and interconnections between subsystems as edges, identifying the network topology and the subsystem dynamics plays an important role in the research of complex networks. This project aims to study the identification methods to recover the topology structure and to determine the subsystem dynamics of a class of complex networks, based on the system observation data. The main work includes: (1) adopt the block-oriented structure to model the topology and the node dynamics of complex networks, mine correlation variables between sub-blocks, study the simplified expression method of the model, so as to solve the modeling problem of complex networks; (2) study the maximum likelihood identification methods based on the Expectation Maximization (EM) algorithm, so as to solve the identification problem of block-oriented network models containing different forms of parameters; (3) study the key variable selection methods and the information compression methods of reducing parameter dimensions, based on the sparse principle of LASSO technology and the compressive sensing technology, so as to improve the accuracy and computational efficiency of the identification algorithm; (4) decompose the large-scale coupled system into many subsystems based on the hierarchical identification principle, and study the highly efficient online identification methods for the large-scale coupled network by combining with the heuristic search method, so as to reduce complexity and computational cost of the identification algorithm. The achieved results have important applications in chemical industrial processes and multi-agent systems.
复杂网络是将复杂动力学系统的子系统及其相互连接抽象为节点和边形成的,其拓扑结构与子系统的动态特性辨识是复杂网络研究的重要内容。本项目从系统辨识角度,基于系统观测数据,研究复杂网络拓扑与子系统动态特性的辨识方法。内容包括:(1)利用模块结构建立复杂网络拓扑及节点动力学模型,挖掘模块间关联变量,探讨模块化模型的简化表达方法,以解决复杂网络模型化问题;(2)基于最大期望EM算法,研究网络的极大似然辨识方法,以解决存在多种形式参数的复杂模块化网络模型的辨识问题;(3)基于稀疏原理的LASSO技术和压缩感知技术,研究主要变量选择方法和降低参数维数的信息压缩方法,以提高辨识算法的精度和计算效率;(4)基于递阶辨识原理将大规模耦合系统分解为众多子系统,结合启发式快速搜索方法,探讨大规模网络的高效在线辨识方法,以减小辨识算法的复杂性和计算量。研究成果在流程工业过程、多智能体系统等领域有广泛的应用前景。
结项摘要
研究了无线传感器网络的鲁棒状态估计方法,复杂模块化系统的系列辨识建模和参数估计方法,以及多智能体的新型最大奖励概率算法。主要内容为:(1)对于状态方程表示的无线传感器网络系统,为了节约传感器能耗,减少网络流量拥塞,分别提出了基于数据驱动传输策略和基于局部事件触发传输策略的鲁棒融合网络状态估计方法。(2)针对双率采样数据/缺失数据模块化非线性系统,基于极大似然原理,研究参数辨识的极大似然算法和最大期望EM算法,解决了存在多种形式参数的缺失数据模块化模型的辨识问题。(3)针对模块化系统的阶次和参数同时辨识问题,基于稀疏原理,提出正交匹配追踪算法,同时求得系统阶次和待估参数;通过采集仅仅大于系统未知参数维数的少量数据,而不是传统系统辨识的上千组数据,进行系统辨识,压缩了测量矩阵维数,提高了辨识算法的精度和计算效率。(4)针对模块化多变量MISO/MIMO系统,利用矩阵变换技术进行模型重塑,进一步利用递阶辨识原理,实现系统的最小二乘/随机梯度辨识方法。克服了传统过参数化辨识模型存在冗余参数,辨识算法计算量大的问题。(5)分别提出解决多智能体协同任务的基于最大回报频率的Q学习方法、基于无穷小学习率梯度上升方法和一种策略梯度潜力方法的最大奖励概率算法,以达到重复游戏中的最大总奖励。并对无穷小学习率梯度上升算法和策略梯度潜力算法的收敛性进行理论分析。(6)提出一种基于改进遗传算法的多AGV路径规划方法和一种新型混合区域控制模式的AGV导引路径配置及其路径调度方法。
项目成果
期刊论文数量(21)
专著数量(0)
科研奖励数量(5)
会议论文数量(13)
专利数量(4)
Multi-AGV path planning with double-path constraints by using an improved genetic algorithm.
基于改进遗传算法的双路径约束多AGV路径规划
- DOI:10.1371/journal.pone.0181747
- 发表时间:2017
- 期刊:PloS one
- 影响因子:3.7
- 作者:Han Z;Wang D;Liu F;Zhao Z
- 通讯作者:Zhao Z
Model recovery for Hammerstein systems using the auxiliary model based orthogonal matching pursuit method
使用基于辅助模型的正交匹配追踪方法进行 Hammerstein 系统的模型恢复
- DOI:10.1016/j.apm.2017.10.005
- 发表时间:2018-02-01
- 期刊:APPLIED MATHEMATICAL MODELLING
- 影响因子:5
- 作者:Wang, Dongqing;Li, Liwei;Yan, Yaru
- 通讯作者:Yan, Yaru
Recursive least squares identification method for a Wiener nonlinear system
维纳非线性系统的递归最小二乘辨识方法
- DOI:--
- 发表时间:2016
- 期刊:ICIC Express Letters
- 影响因子:--
- 作者:Wang XD;Song DL;Wang DQ(王冬青)
- 通讯作者:Wang DQ(王冬青)
FMRQ-A Multiagent Reinforcement Learning Algorithm for Fully Cooperative Tasks
FMRQ-一种用于完全协作任务的多智能体强化学习算法
- DOI:10.1109/tcyb.2016.2544866
- 发表时间:2017
- 期刊:Ieee Transactions ON Cybernetics
- 影响因子:11.8
- 作者:Zhang Zhen;Zhao Dongbin;Gao Junwei;Wang Dongqing;Dai Yujie
- 通讯作者:Dai Yujie
Robust state estimation for wireless sensor networks with data-driven communication
具有数据驱动通信的无线传感器网络的鲁棒状态估计
- DOI:10.1002/rnc.3819
- 发表时间:2017-12-01
- 期刊:INTERNATIONAL JOURNAL OF ROBUST AND NONLINEAR CONTROL
- 影响因子:3.9
- 作者:Liu, Huabo;Wang, Dongqing
- 通讯作者:Wang, Dongqing
数据更新时间:{{ journalArticles.updateTime }}
{{
item.title }}
{{ item.translation_title }}
- DOI:{{ item.doi || "--"}}
- 发表时间:{{ item.publish_year || "--" }}
- 期刊:{{ item.journal_name }}
- 影响因子:{{ item.factor || "--"}}
- 作者:{{ item.authors }}
- 通讯作者:{{ item.author }}
数据更新时间:{{ journalArticles.updateTime }}
{{ item.title }}
- 作者:{{ item.authors }}
数据更新时间:{{ monograph.updateTime }}
{{ item.title }}
- 作者:{{ item.authors }}
数据更新时间:{{ sciAawards.updateTime }}
{{ item.title }}
- 作者:{{ item.authors }}
数据更新时间:{{ conferencePapers.updateTime }}
{{ item.title }}
- 作者:{{ item.authors }}
数据更新时间:{{ patent.updateTime }}
其他文献
2D和3D MRI结合技术在胰腺癌诊断
- DOI:--
- 发表时间:--
- 期刊:中华肿瘤杂志,29(3):216-220,2007/03
- 影响因子:--
- 作者:王冬青;曾蒙苏*;靳大勇;楼文
- 通讯作者:楼文
电力信息物理系统的攻击行为与安全防护
- DOI:--
- 发表时间:2017
- 期刊:电力系统自动化
- 影响因子:--
- 作者:李田;苏盛;杨洪明;文福拴;王冬青;朱林
- 通讯作者:朱林
具有期望时变容错域的联想记忆模型的新设计方法
- DOI:--
- 发表时间:--
- 期刊:计算机学报
- 影响因子:--
- 作者:杨国为;王冬青;庄晓东;曹文谊
- 通讯作者:曹文谊
全厂断电事故下AP1000非能动余热排出系统瞬态特性数值分析
- DOI:--
- 发表时间:2013
- 期刊:原子能科学技术
- 影响因子:--
- 作者:王冬青;董华平;姜晶;张建民
- 通讯作者:张建民
胰腺囊性肿瘤92例临床病理分析
- DOI:--
- 发表时间:--
- 期刊:中华病理学杂志,36(3):160-165.2007/03
- 影响因子:--
- 作者:纪元;朱雄增;楼文辉;王冬青
- 通讯作者:王冬青
其他文献
{{
item.title }}
{{ item.translation_title }}
- DOI:{{ item.doi || "--" }}
- 发表时间:{{ item.publish_year || "--"}}
- 期刊:{{ item.journal_name }}
- 影响因子:{{ item.factor || "--" }}
- 作者:{{ item.authors }}
- 通讯作者:{{ item.author }}
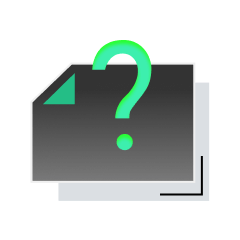
内容获取失败,请点击重试
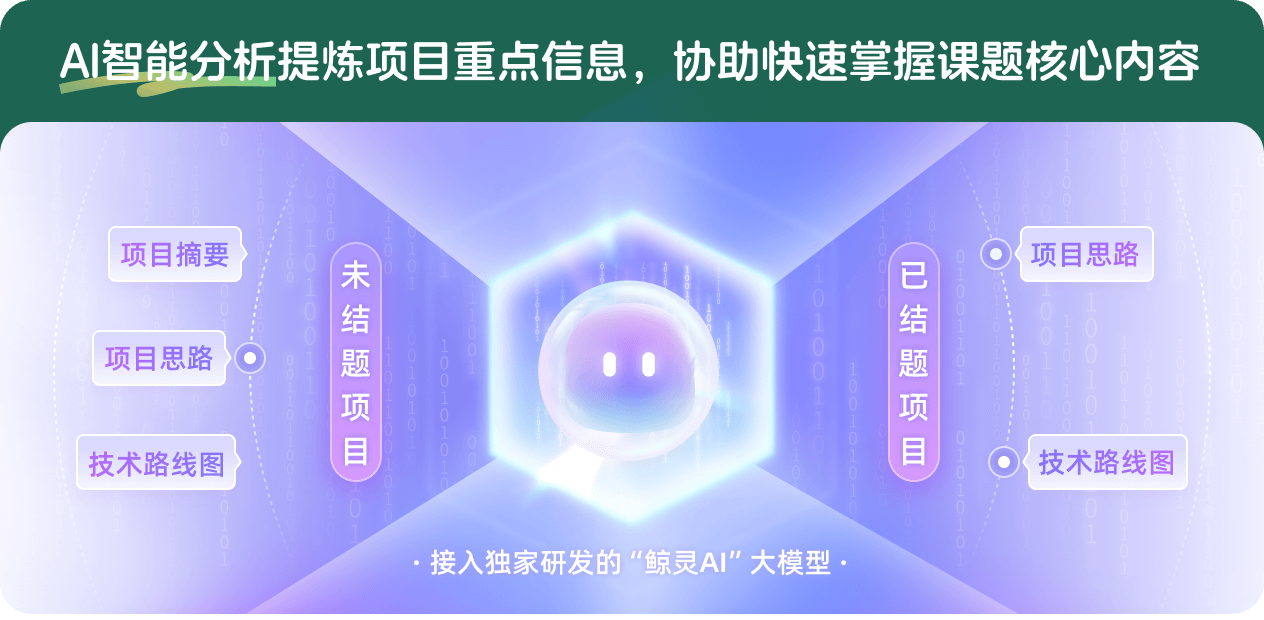
查看分析示例
此项目为已结题,我已根据课题信息分析并撰写以下内容,帮您拓宽课题思路:
AI项目摘要
AI项目思路
AI技术路线图
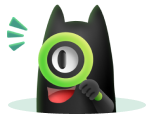
请为本次AI项目解读的内容对您的实用性打分
非常不实用
非常实用
1
2
3
4
5
6
7
8
9
10
您认为此功能如何分析更能满足您的需求,请填写您的反馈:
王冬青的其他基金
基于数据驱动的动力电池多模型融合建模与状态估计
- 批准号:
- 批准年份:2022
- 资助金额:54 万元
- 项目类别:面上项目
基于数据驱动的动力电池多模型融合建模与状态估计
- 批准号:62273190
- 批准年份:2022
- 资助金额:54.00 万元
- 项目类别:面上项目
基于数据特征的多模态过程辨识建模方法
- 批准号:61873138
- 批准年份:2018
- 资助金额:63.0 万元
- 项目类别:面上项目
相似国自然基金
{{ item.name }}
- 批准号:{{ item.ratify_no }}
- 批准年份:{{ item.approval_year }}
- 资助金额:{{ item.support_num }}
- 项目类别:{{ item.project_type }}
相似海外基金
{{
item.name }}
{{ item.translate_name }}
- 批准号:{{ item.ratify_no }}
- 财政年份:{{ item.approval_year }}
- 资助金额:{{ item.support_num }}
- 项目类别:{{ item.project_type }}