基于支持向量机与图搜索的PET-CT图像中肺肿瘤分割算法研究
项目介绍
AI项目解读
基本信息
- 批准号:61401293
- 项目类别:青年科学基金项目
- 资助金额:24.0万
- 负责人:
- 依托单位:
- 学科分类:F0116.图像信息处理
- 结题年份:2017
- 批准年份:2014
- 项目状态:已结题
- 起止时间:2015-01-01 至2017-12-31
- 项目参与者:邓胜明; 朱伟芳; 石霏; 高向军; 金超; 鞠薇; 曹兆源; 蒋雪晴; 张莉;
- 关键词:
项目摘要
In recent years, cancer has been becoming more and more serious to public health, and lung tumors are the leading cause of cancer death. In worldwide, annual mortality from lung cancer is expected to exceed 13 million by 2030. Due to the integration of the advantages of the two imaging, PET-CT bimodality imaging has become one of the best choices of this stage lung cancer diagnosis, in order to non-invasively describe the physiological and morphological properties of lung tumors. However, lung tumors in PET images and CT images are often displayed differently, and the quality of PET-CT images are comparatively low; besides of individual differences, the anatomy, physiology and pathology of the human organs are extremely complex, which makes lung tumor segmentation in PET-CT become a major research focus and difficulty. In order to overcome the problems, this project will be concentrated on researches and development of lung tumor detection based on support vector machine (SVM), precise segmentation of lung tumor based on graph search,so as to accurately provide physiological metabolism and anatomical structure information of lung tumors and related structures. This project will provide basis and proofs for doctors to determine the objective staging of lung cancer, accurate diagnosis, rapid treatment plan and effectively evaluation of treatment, which will greatly reduce trial-and-error efforts and unreliability of lung cancer diagnosis and treatment.
近年来,癌症对人类健康的危害日益严重,肺癌则居于癌症死亡病例中的首位,到2030年,全球每年肺癌的死亡人数预计将超过1300万人。PET-CT双模态影像技术由于整合了两种成像技术的优势,为描述肺肿瘤的生理学与形态学特性提供了非侵入式手段,已经成为现阶段肺肿瘤诊断的最佳选择之一。然而,由于PET与CT图像中肺肿瘤显像通常有较大差异,PET-CT图像质量较低,人体器官的解剖、生理与病理的结构较复杂、个体差异较大等问题的存在,使得PET-CT图像中肺肿瘤的精确分割成为当前医学影像处理研究领域的一大研究热点与难点。为克服上述问题,本项目拟进行基于支持向量机的肺肿瘤检测、基于图搜索的肺肿瘤精确分割等算法研究,为客观地确定肺癌分期、准确地做出诊断、快速地制定治疗方案和有效地评估治疗后效果提供决策依据,这将大大降低肺癌诊断与治疗的盲目性和不可靠性,降低死亡率,提高生存率。
结项摘要
近年来,癌症对人类健康的危害日益严重,肺癌则居于癌症死亡病例中的首位,到2030年,全球每年肺癌的死亡人数预计将超过1300万人。PET-CT双模态影像技术由于整合了两种成像技术的优势,为描述肺肿瘤的生理学与形态学特性提供了非侵入式手段,已经成为现阶段肺肿瘤诊断的最佳选择之一。然而,由于PET与CT图像中肺肿瘤显像通常有较大差异,PET-CT图像质量较低,人体器官的解剖、生理与病理的结构较复杂、个体差异较大等问题的存在,使得PET-CT图像中肺肿瘤的精确分割成为当前医学影像处理研究领域的一大研究热点与难点。为克服上述问题,本项目研究PET-CT 多模态影像中的肺肿瘤精确分割算法,先后提出了基于随机游走和图割联合算法的PET-CT肺肿瘤分割、三维图割算法结合随机游走算法的PET-CT肺肿瘤分割方法、基于随机森林和BSS特征的PET-CT肺肿瘤分割、基于统计形状模型和可形变图割的CT图像脏器算法、基于统计形状模型与非均匀图搜索分割算法等五种方法,从而解决肺肿瘤个体差异、不同模态数据中形状有所差异等问题,从而降低肺肿瘤诊断的假阴性和假阳性率,并在国内外高水平学术期刊上发表SCI论文7 篇、EI检索论文4篇,获批国家发明专利授权3项,申请国家发明专利4 项。另外,研发计算机辅助肺癌诊断软件平台MPAV,对肺肿瘤区域的定量分析提供可靠的方法与工具,为医生客观地确定肺癌研究、准确地做出诊断、快速地制定治疗方案提供决策依据,有助于降低肺癌诊断与治疗的盲目性和不可靠性。
项目成果
期刊论文数量(6)
专著数量(0)
科研奖励数量(0)
会议论文数量(3)
专利数量(2)
3D Fast Automatic Segmentation of Kidney Based on Modified AAM and Random Forest
基于改进AAM和随机森林的肾脏3D快速自动分割
- DOI:10.1109/tmi.2015.2512606
- 发表时间:2016-01
- 期刊:IEEE Transactions on Medical Imaging
- 影响因子:10.6
- 作者:Chao Jin;Fei Shi;Dehui Xiang;Xueqing Jiang;Bin Zhang;Ximing Wang;Weifang Zhu;Enting Gao;Xinjian Chen
- 通讯作者:Xinjian Chen
Random Walk and Graph Cut for Co-Segmentation of Lung Tumor on PET-CT Images
PET-CT 图像上肺肿瘤联合分割的随机游走和图形切割。
- DOI:10.1109/tip.2015.2488902
- 发表时间:2015-12-01
- 期刊:IEEE TRANSACTIONS ON IMAGE PROCESSING
- 影响因子:10.6
- 作者:Ju, Wei;Xiang, Deihui;Chen, Xinjian
- 通讯作者:Chen, Xinjian
Automated 3-D Retinal Layer Segmentation of Macular Optical Coherence Tomography Images With Serous Pigment Epithelial Detachments
具有浆液性色素上皮脱离的黄斑光学相干断层扫描图像的自动 3D 视网膜层分割
- DOI:10.1109/tmi.2014.2359980
- 发表时间:2015-02-01
- 期刊:IEEE TRANSACTIONS ON MEDICAL IMAGING
- 影响因子:10.6
- 作者:Shi, Fei;Chen, Xinjian;Chen, Haoyu
- 通讯作者:Chen, Haoyu
An automated framework for 3D serous pigment epithelium detachment segmentation in SD-OCT images.
SD-OCT 图像中 3D 浆液性色素上皮脱离分割的自动化框架
- DOI:10.1038/srep21739
- 发表时间:2016-02-22
- 期刊:Scientific reports
- 影响因子:4.6
- 作者:Sun Z;Chen H;Shi F;Wang L;Zhu W;Xiang D;Yan C;Li L;Chen X
- 通讯作者:Chen X
Automatic Liver Segmentation Based on Shape Constraints and Deformable Graph Cut in CT Images
CT图像中基于形状约束和变形图切割的自动肝脏分割
- DOI:10.1109/tip.2015.2481326
- 发表时间:2015-12-01
- 期刊:IEEE TRANSACTIONS ON IMAGE PROCESSING
- 影响因子:10.6
- 作者:Li, Guodong;Chen, Xinjian;Xiang, Dehui
- 通讯作者:Xiang, Dehui
数据更新时间:{{ journalArticles.updateTime }}
{{
item.title }}
{{ item.translation_title }}
- DOI:{{ item.doi || "--"}}
- 发表时间:{{ item.publish_year || "--" }}
- 期刊:{{ item.journal_name }}
- 影响因子:{{ item.factor || "--"}}
- 作者:{{ item.authors }}
- 通讯作者:{{ item.author }}
数据更新时间:{{ journalArticles.updateTime }}
{{ item.title }}
- 作者:{{ item.authors }}
数据更新时间:{{ monograph.updateTime }}
{{ item.title }}
- 作者:{{ item.authors }}
数据更新时间:{{ sciAawards.updateTime }}
{{ item.title }}
- 作者:{{ item.authors }}
数据更新时间:{{ conferencePapers.updateTime }}
{{ item.title }}
- 作者:{{ item.authors }}
数据更新时间:{{ patent.updateTime }}
其他文献
其他文献
{{
item.title }}
{{ item.translation_title }}
- DOI:{{ item.doi || "--" }}
- 发表时间:{{ item.publish_year || "--"}}
- 期刊:{{ item.journal_name }}
- 影响因子:{{ item.factor || "--" }}
- 作者:{{ item.authors }}
- 通讯作者:{{ item.author }}
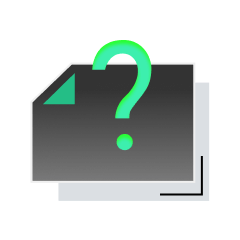
内容获取失败,请点击重试
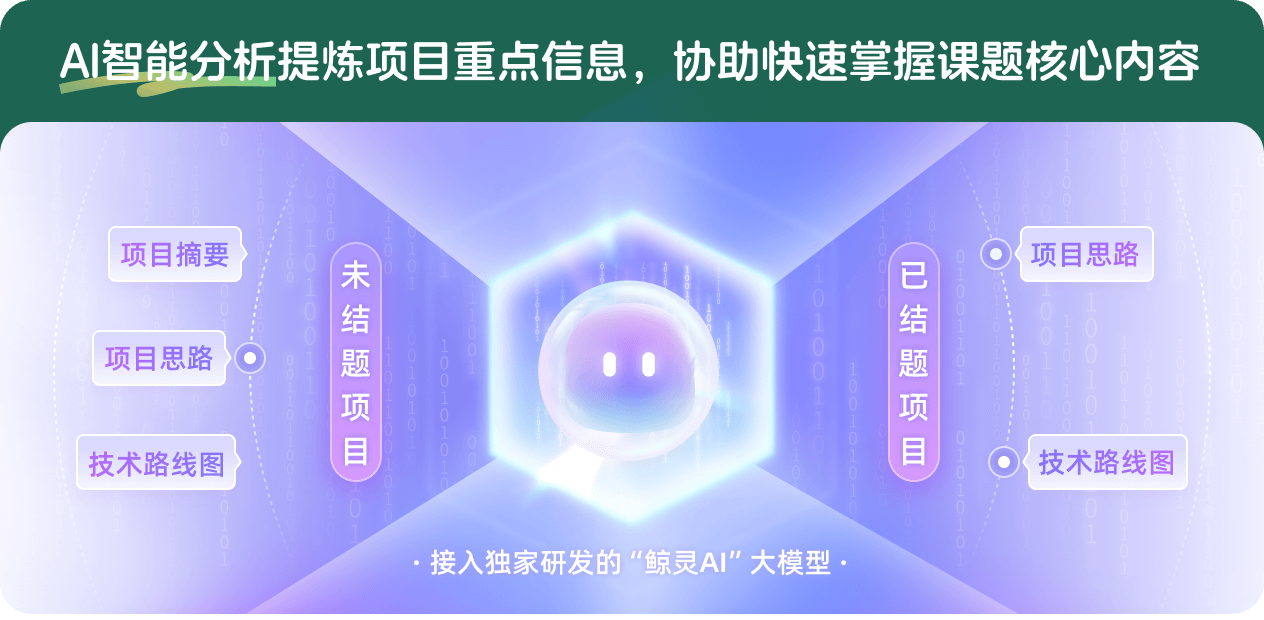
查看分析示例
此项目为已结题,我已根据课题信息分析并撰写以下内容,帮您拓宽课题思路:
AI项目摘要
AI项目思路
AI技术路线图
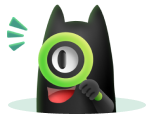
请为本次AI项目解读的内容对您的实用性打分
非常不实用
非常实用
1
2
3
4
5
6
7
8
9
10
您认为此功能如何分析更能满足您的需求,请填写您的反馈:
向德辉的其他基金
面向年龄相关性黄斑变性诊断的迁移学习算法研究
- 批准号:62371328
- 批准年份:2023
- 资助金额:53 万元
- 项目类别:面上项目
面向息肉样脉络膜血管病变多源异构数据的深度学习算法研究
- 批准号:61971298
- 批准年份:2019
- 资助金额:59 万元
- 项目类别:面上项目
相似国自然基金
{{ item.name }}
- 批准号:{{ item.ratify_no }}
- 批准年份:{{ item.approval_year }}
- 资助金额:{{ item.support_num }}
- 项目类别:{{ item.project_type }}
相似海外基金
{{
item.name }}
{{ item.translate_name }}
- 批准号:{{ item.ratify_no }}
- 财政年份:{{ item.approval_year }}
- 资助金额:{{ item.support_num }}
- 项目类别:{{ item.project_type }}