面部伪造视频的鲁棒性检测方法研究
项目介绍
AI项目解读
基本信息
- 批准号:61906052
- 项目类别:青年科学基金项目
- 资助金额:27.0万
- 负责人:
- 依托单位:
- 学科分类:F0604.机器感知与机器视觉
- 结题年份:2022
- 批准年份:2019
- 项目状态:已结题
- 起止时间:2020-01-01 至2022-12-31
- 项目参与者:--
- 关键词:
项目摘要
Recently face swapping and manipulation receives more and more attentions with the development of various, polytropic, and adversarial techniques. Detecting the tampered face video plays an important role in real application and can bring great intellectual merit. In this project, we explore the robust methods for tampered face video detection in perspective of feature learning, multi-scale and temporal-spatial modeling. First, we propose to combine the mid-level vision learning and the low-level statistics for feature fusion to cope with the representation limits of low-level vision and the discriminative limits of high-level vision. Second, we propose a progressive convolutional neural network to utilize the training results under each image scale. This method can capture the huge characteristic differences of each scale, which is difficult to be modelled consistently. Finally, we try to exploit the structural feature from the high-dimensional spatial- temporal domain, and propose a graph convolutional neural network to study the essential structure hidden in spatial-temporal pattern. We expect to provide some new solutions for robust detection of tampered face image and video.
面部伪造图像或视频技术有着多样性、多变性和对抗性的特点,针对伪造视频实现鲁棒性检测具有很大的学术意义和实用价值,本课题拟针对特征表示鲁棒、尺度变化鲁棒和空时变化鲁棒问题.(1)在充分分析面部伪造的特点基础上,提出中级视觉特征和低级统计特性融合学习的方法,解决低级视觉特征表示不足和高层语义特征区分难的问题;.(2)针对不同尺度下的伪造特征差异大,获取一致性模型难的问题,提出一种渐进式多尺度卷积神经网络模型,充分利用各分辨率下的训练结果;.(3)针对高维视频空时域中获取结构性特征的难题,提出一种基于图卷积神经网络建模方法,充分挖掘空时模式中内在的结构性关联。.通过上述方面的研究,可为解决鲁棒性面部伪造检测问题提供新的思路。
结项摘要
面部伪造图像或视频技术有着多样性、多变性和对抗性的特点,针对伪造视频实现鲁棒性检测具有很大的学术意义和实用价值,本课题拟针对特征表示鲁棒、尺度变化鲁棒和空时变化鲁棒问题.(1)提出了一种多尺度纹理感知的计算机生成图像鉴别模型。该模型首先基于图像特征之间的相关性进行纹理增强,再通过GRAM矩阵对视觉上的相关性进行建模,进一步提取纹理特征,然后将多个纹理提取模块应用于特征提取网络的不同层以提取多尺度纹理特征。最后基于多尺度纹理特征进行计算机生成图像的判别;.(2)提出基于样本独立的动态多尺度融合目标检测算法(DSIC)。通过模拟大脑神经的兴奋与抑制状态,针对不同输入样本,根据激活状态动态地打开与关闭输入与连接的路径,以此实现更为灵活且动态的多尺度目标检测。算法的主要特点在于,DSIC 可动态的选择输入和连接,避免通过固定的设计,自动调节连接和特征交互过程以适应不同的样本;.(3)提出基于Cross-Transformer的压缩域少样本视频分类方法。首先对视频进行部分解码得到压缩域信息,利用压缩域信息计算视频信息量分布,根据这一分布选取最具鉴别力、对分类帮助最大的视频帧,将其输入分类网络。分类网络由短时模块和长时模块组成,短时模块在视频压缩域子序列内进行短时特征融合,长时模块则在整个视频序列内提炼有效的视频特征。最后通过Cross-Transformer将未知类别的查询视频与已知类别的支撑视频的特征进行对齐匹配,按照最近邻原则进行分类。.算法在已有的数据库达到96.49%的平均准确率,超过了现有的鉴别方法,充分证明了算法的有效性。.
项目成果
期刊论文数量(1)
专著数量(0)
科研奖励数量(0)
会议论文数量(2)
专利数量(5)
Robust Texture-Aware Computer-Generated Image Forensic: Benchmark and Algorithm
强大的纹理感知计算机生成图像取证:基准和算法
- DOI:10.1109/tip.2021.3114989
- 发表时间:2021-10
- 期刊:IEEE TRANSACTIONS ON IMAGE PROCESSING
- 影响因子:10.6
- 作者:Bai Weiming;Zhang Zhipeng;Li Bing;Wang Pei;Li Yangxi;Zhang Congxuan;Hu Weiming
- 通讯作者:Hu Weiming
数据更新时间:{{ journalArticles.updateTime }}
{{
item.title }}
{{ item.translation_title }}
- DOI:{{ item.doi || "--"}}
- 发表时间:{{ item.publish_year || "--" }}
- 期刊:{{ item.journal_name }}
- 影响因子:{{ item.factor || "--"}}
- 作者:{{ item.authors }}
- 通讯作者:{{ item.author }}
数据更新时间:{{ journalArticles.updateTime }}
{{ item.title }}
- 作者:{{ item.authors }}
数据更新时间:{{ monograph.updateTime }}
{{ item.title }}
- 作者:{{ item.authors }}
数据更新时间:{{ sciAawards.updateTime }}
{{ item.title }}
- 作者:{{ item.authors }}
数据更新时间:{{ conferencePapers.updateTime }}
{{ item.title }}
- 作者:{{ item.authors }}
数据更新时间:{{ patent.updateTime }}
其他文献
其他文献
{{
item.title }}
{{ item.translation_title }}
- DOI:{{ item.doi || "--" }}
- 发表时间:{{ item.publish_year || "--"}}
- 期刊:{{ item.journal_name }}
- 影响因子:{{ item.factor || "--" }}
- 作者:{{ item.authors }}
- 通讯作者:{{ item.author }}
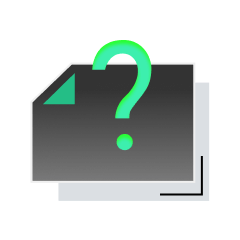
内容获取失败,请点击重试
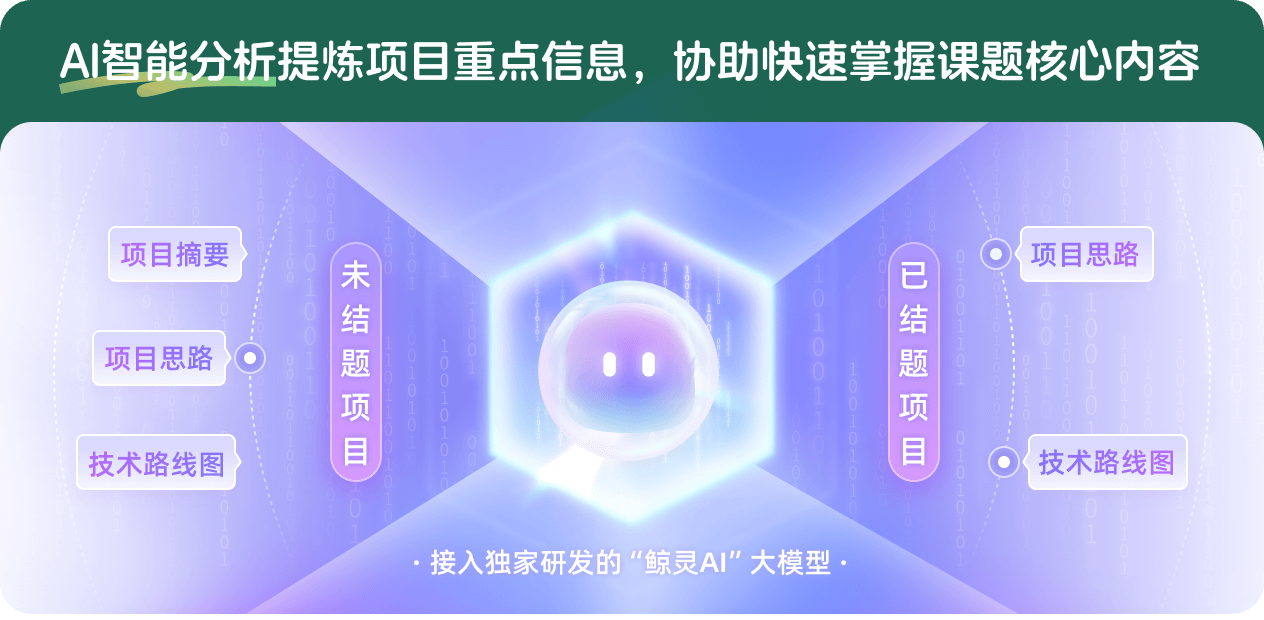
查看分析示例
此项目为已结题,我已根据课题信息分析并撰写以下内容,帮您拓宽课题思路:
AI项目摘要
AI项目思路
AI技术路线图
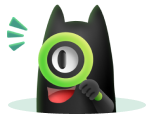
请为本次AI项目解读的内容对您的实用性打分
非常不实用
非常实用
1
2
3
4
5
6
7
8
9
10
您认为此功能如何分析更能满足您的需求,请填写您的反馈:
相似国自然基金
{{ item.name }}
- 批准号:{{ item.ratify_no }}
- 批准年份:{{ item.approval_year }}
- 资助金额:{{ item.support_num }}
- 项目类别:{{ item.project_type }}
相似海外基金
{{
item.name }}
{{ item.translate_name }}
- 批准号:{{ item.ratify_no }}
- 财政年份:{{ item.approval_year }}
- 资助金额:{{ item.support_num }}
- 项目类别:{{ item.project_type }}