面向异构系统的可扩展的迭代方法并行优化模型及其应用研究
项目介绍
AI项目解读
基本信息
- 批准号:61872422
- 项目类别:面上项目
- 资助金额:63.0万
- 负责人:
- 依托单位:
- 学科分类:F0204.计算机系统结构与硬件技术
- 结题年份:2022
- 批准年份:2018
- 项目状态:已结题
- 起止时间:2019-01-01 至2022-12-31
- 项目参与者:王雨顺; 蔡文君; 周星星; 杨艳红; 蒋朝龙; 穆振国; 张甜; 江琪; 殷人杰;
- 关键词:
项目摘要
With the rapid improvement of the computation capacity of computers and the increasing depth and scale of the problems,researchers focus on the GPU computing to improve the performance of iterative methods for solving the large-scale sparse linear system Ax = b over the recent years. Given that there is not any GPU-accelerated iterative method that is always highly efficient for all sparse linear systems, and constructing it is expensive and time-consuming, based on the heterogeneous multi-GPU platform, we take several popular preconditioned iterative methods for example in this project, analyze the main ingredients influencing the performance of iterative methods, and thus construct the parallel optimization models of the vector operation, inner product, sparse matrix-vector multiplication, and preconditioner. My target is to present an adaptive parallel optimization model and the corresponding theoretical system for iterative methods on any multi-GPU platform. Using the proposed optimization model of iterative methods, we can automatically and rapidly construct the optimal parallel iterative method on multi-GPUs for any given sparse linear system. We validate our models by applying them to the electromagnetic field. This project presents a new idea of how to automatically and rapidly construct the optimal multi-GPU parallel iterative method, which is meaningful for the development of promoting the parallel computing.
随着计算机计算能力的提高,人们探讨问题的深度和规模也随着增大,为适应求解大规模稀疏线性系统Ax=b的需要,近些年通过GPU计算提高迭代求解算法性能的研究已得到广泛的关注。考虑不存在普遍适用的基于GPU的异构并行迭代方法,而且构造它是繁重且耗时的,为此,本研究面向CPU+多GPU异构系统,基于CUDA编程模型,针对流行的预条件迭代方法,分析影响其性能的关键成分,深入研究构建与具体问题无关的矢量运算、内积和稀疏矩阵矢量乘的并行优化模型、并行预条件子算法及迭代方法并行优化框架,建立一整套可扩展的迭代方法并行优化模型和理论体系,以达到任意给定稀疏线性系统,能够自动快速地构建求解此稀疏线性系统的最优化多GPU并行迭代方法。通过在电磁计算中的应用,验证模型有效性和实用性。本项目为如何自动快速地建立最优性能的多GPU并行迭代方法提供了一个新的思路,对推动并行计算的发展有着重要的理论意义和实际应用价值。
结项摘要
在计算流体力学、电磁计算等诸多领域实际应用中,将描述自然现象的偏微分方程离散后,通常会得到一个稀疏的线性系统Ax=b,求解稀疏线性系统对整个应用问题的解决至关重要。求解的算法主要有两种:直接法和迭代法。相对于直接法,迭代法因运算量和存储量小而备受研究者们的青睐。随着计算机计算能力的提高,人们探讨问题的深度和广度也逐渐增大,要求必须提高线性系统的迭代求解效率,并行计算自然成为提高迭代法性能的有效手段首选。. 项目围绕建立高效的CPU/GPU异构并行迭代算法,面向CPU/GPU异构平台,基于CUDA编程模型,展开建立一系列高效并行求解算法的研究。主要的研究内容包括并行稀疏矩阵矢量乘、并行预条件子、并行迭代算法及性能优化等方面,取得了一系列研究成果。首先针对有散点和长零段的稀疏对角矩阵,提出了一种新的存储格式,克服了流行对角存储格式大量零填充的缺陷;进一步,对对角矩阵分类,针对每一类矩阵提出一种高效的存储格式和核函数,并通过设计的搜索引擎,使得任给一个对角稀疏矩阵,能自动识别其类别,从而选择最优的存储格式和核函数;其次,针对基于F范数的稀疏近似逆,提出了一种自适应线程分配的高并行静态稀疏近似逆预条件算法;一种排序的多GPU并行静态稀疏近似逆预条件算法;进一步,针对现有基于F范数的动态稀疏近似逆预条件子算法面对大规模问题出现内存溢出异常错误这一共性问题,以一种最流行的其算法为例,分析其错误原因,并给出了解决方法,且以此为基础,提出了一种面向GPU高并行动态稀疏近似逆预条件子算法;最后,在CPU/GPU异构平台上,构建了高性能迭代算法库,并针对对称正定的稀疏矩阵,研究构建了一个自动生成预条件共轭梯度算法系统。应用本研究提出的算法去解实际的应用问题,取得不错的效果。总结起来,本研究不仅为建立最优性能的GPU并行迭代方法提供了一个新的思路,而且对推动并行计算的发展也有着重要的实际应用价值。
项目成果
期刊论文数量(19)
专著数量(0)
科研奖励数量(1)
会议论文数量(6)
专利数量(0)
A parallel sparse approximate inverse preconditioning algorithm based on MPI and CUDA
基于MPI和CUDA的并行稀疏近似逆预处理算法
- DOI:10.1016/j.tbench.2021.100007
- 发表时间:2021-11
- 期刊:BenchCouncil Transactions on Benchmarks, Standards and Evaluations
- 影响因子:--
- 作者:Wang Yizhou;Li Wenhao;Gao Jiaquan
- 通讯作者:Gao Jiaquan
A linearly implicit structure-preserving scheme for the fractional sine-Gordon equation based on the IEQ approach
基于 IEQ 方法的分数正弦-Gordon 方程的线性隐式保结构方案
- DOI:10.1016/j.apnum.2020.10.009
- 发表时间:2019-11
- 期刊:Applied Numerical Mathematics
- 影响因子:2.8
- 作者:Fu Yayun;Cai Wenjun;Wang Yushun
- 通讯作者:Wang Yushun
Structure-preserving algorithms for the two-dimensional sine-Gordon equation with Neumann boundary conditions
具有诺依曼边界条件的二维正弦-戈登方程的结构保持算法
- DOI:10.1016/j.jcp.2019.05.048
- 发表时间:2018-09
- 期刊:Journal of Computational Physics
- 影响因子:4.1
- 作者:Cai Wenjun;Jiang Chaolong;Wang Yushun;Song Yongzhong
- 通讯作者:Song Yongzhong
面向印花图案检索的特征融合方法研究
- DOI:--
- 发表时间:2022
- 期刊:南京师范大学学报
- 影响因子:--
- 作者:殷业瑜;高家全;李莹
- 通讯作者:李莹
An efficient sparse approximate inverse preconditioning algorithm on GPU
GPU上高效稀疏近似逆预处理算法
- DOI:10.1002/cpe.5598
- 发表时间:2019-12
- 期刊:Concurrency and Computation- Practice & Experience
- 影响因子:--
- 作者:He Guixia;Yin Renjie;Gao Jiaquan
- 通讯作者:Gao Jiaquan
数据更新时间:{{ journalArticles.updateTime }}
{{
item.title }}
{{ item.translation_title }}
- DOI:{{ item.doi || "--"}}
- 发表时间:{{ item.publish_year || "--" }}
- 期刊:{{ item.journal_name }}
- 影响因子:{{ item.factor || "--"}}
- 作者:{{ item.authors }}
- 通讯作者:{{ item.author }}
数据更新时间:{{ journalArticles.updateTime }}
{{ item.title }}
- 作者:{{ item.authors }}
数据更新时间:{{ monograph.updateTime }}
{{ item.title }}
- 作者:{{ item.authors }}
数据更新时间:{{ sciAawards.updateTime }}
{{ item.title }}
- 作者:{{ item.authors }}
数据更新时间:{{ conferencePapers.updateTime }}
{{ item.title }}
- 作者:{{ item.authors }}
数据更新时间:{{ patent.updateTime }}
其他文献
出租车GPS大数据的道路行车可视分析
- DOI:--
- 发表时间:2014
- 期刊:计算机辅助设计与图形学学报
- 影响因子:--
- 作者:何贤国;孙国道;高家全;郑春益;梁荣华
- 通讯作者:梁荣华
基于GPU的SSOR稀疏近似逆预条件研究
- DOI:--
- 发表时间:2016
- 期刊:浙江工业大学学报
- 影响因子:--
- 作者:高家全;王志超
- 通讯作者:王志超
基于GPU的高效并行l1最小化算法
- DOI:--
- 发表时间:2016
- 期刊:浙江工业大学学报
- 影响因子:--
- 作者:高家全;李泽界
- 通讯作者:李泽界
其他文献
{{
item.title }}
{{ item.translation_title }}
- DOI:{{ item.doi || "--" }}
- 发表时间:{{ item.publish_year || "--"}}
- 期刊:{{ item.journal_name }}
- 影响因子:{{ item.factor || "--" }}
- 作者:{{ item.authors }}
- 通讯作者:{{ item.author }}
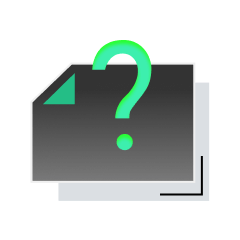
内容获取失败,请点击重试
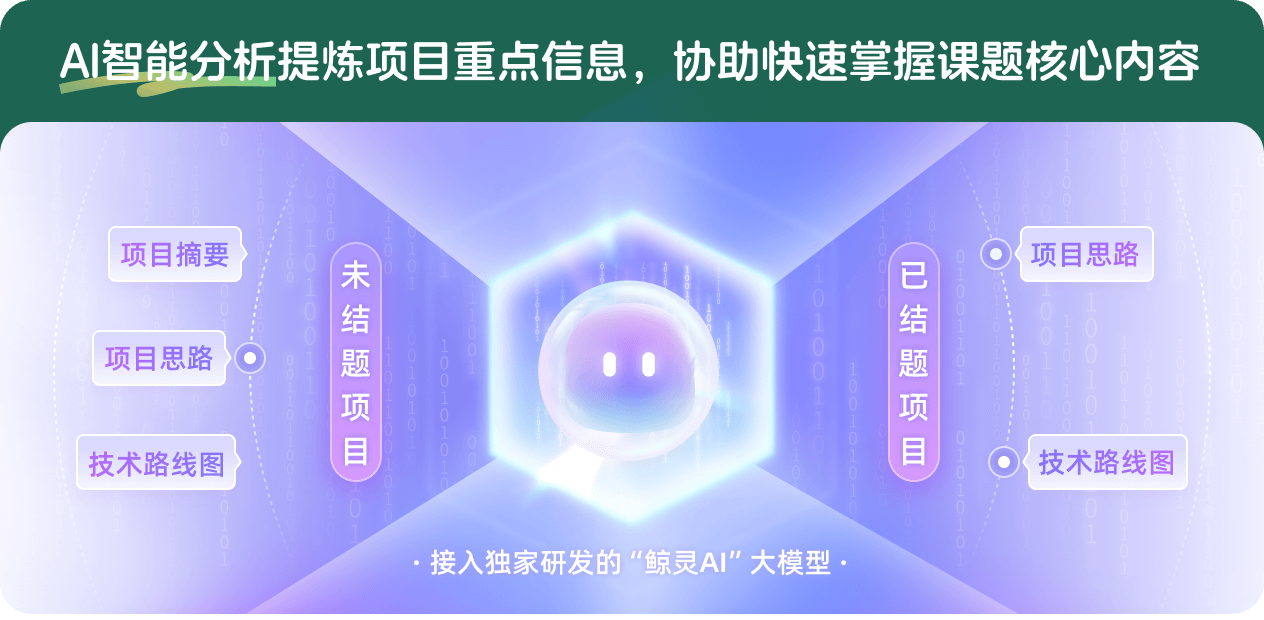
查看分析示例
此项目为已结题,我已根据课题信息分析并撰写以下内容,帮您拓宽课题思路:
AI项目摘要
AI项目思路
AI技术路线图
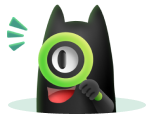
请为本次AI项目解读的内容对您的实用性打分
非常不实用
非常实用
1
2
3
4
5
6
7
8
9
10
您认为此功能如何分析更能满足您的需求,请填写您的反馈:
相似国自然基金
{{ item.name }}
- 批准号:{{ item.ratify_no }}
- 批准年份:{{ item.approval_year }}
- 资助金额:{{ item.support_num }}
- 项目类别:{{ item.project_type }}
相似海外基金
{{
item.name }}
{{ item.translate_name }}
- 批准号:{{ item.ratify_no }}
- 财政年份:{{ item.approval_year }}
- 资助金额:{{ item.support_num }}
- 项目类别:{{ item.project_type }}