面向特征提取的深度鉴别稀疏表示学习方法研究
项目介绍
AI项目解读
基本信息
- 批准号:61806098
- 项目类别:青年科学基金项目
- 资助金额:22.0万
- 负责人:
- 依托单位:
- 学科分类:F0605.模式识别与数据挖掘
- 结题年份:2021
- 批准年份:2018
- 项目状态:已结题
- 起止时间:2019-01-01 至2021-12-31
- 项目参与者:郑豪; 吴海勇; 王燕清; 王寅同; 朱杰;
- 关键词:
项目摘要
Feature extraction is an important research topic in the field of pattern recognition and computer vision. As a popular feature extraction method, sparse representation learning cannot obtain the high-level features effectively because it is a shallow model. This project would investigate the deep sparse representation learning for feature extraction by integrating dictionary learning and deep learning, to establish a deep sparse representation learning framework and to enrich and develop the theoretical system of feature extraction. Particularly, the points of our research mainly include: (1) Referencing the framework of deep learning model, constructing deep discriminative analysis-synthesis dictionary learning method, making the feature more representative and discriminative with faster extraction efficiency. (2) Studying the structure information characterization methods, which has superior performance, enhancing the identification ability in convolutional sparse coding, establishing multi-layer discriminative convolutional sparse representation learning method. (3) Exploring the mechanism of the effective combination of dictionary learning and deep learning, establishing dictionary-driven deep learning method, designing a similar end-to-end solution algorithm..The project will develop the theory and method of deep sparse representation learning with strong representation and discrimination ability, form a systematic learning framework about deep discriminative sparse representation learning to improve the understanding and perception of computer.
特征提取是模式识别和计算机视觉领域中极其重要的研究课题。作为一种主流的特征提取方法,属于浅层模型的稀疏表示学习无法有效地获取数据的高层表示。针对这一问题,本项目拟结合字典学习和深度学习等模型和理论,研究面向特征提取的深度鉴别稀疏表示学习方法,建立深度鉴别稀疏表示学习框架,丰富和发展特征提取理论体系。具体的研究内容包括:(1)借鉴深度学习框架,设计深度鉴别解析-综合字典学习方法,使得特征的表示和鉴别能力更好同时提取效率更快;(2)研究性能优越的结构信息刻画方法,增强卷积稀疏编码的鉴别能力,建立多层鉴别卷积稀疏表示学习方法;(3)探讨字典学习和深度学习有效结合的机制,建立字典驱动的深度表示学习方法并设计类似端到端的快速求解算法。.本项目将大力发展具有强表示能力和鉴别能力的深度稀疏表示学习理论与方法,形成系统的深度鉴别稀疏表示学习框架,进一步提高计算机对图像的理解和感知能力。
结项摘要
提取数据的高层特征表示对于人脸识别、图像分类等应用至关重要。稀疏表示学习是特征提取的主流方法之一,但是它只能提取数据的浅层特征,针对复杂问题的泛化能力较弱;深度学习模型可以获得数据的高层表示,但是在处理小样本数据问题时容易出现过拟合。本项目拟结合稀疏表示学习和深度学习等模型和理论,研究面向特征提取的深度鉴别稀疏表示学习方法,有效地提取数据的高层特征表示。(1)构造多种结构信息的刻画方法,提出深度鉴别解析-综合字典学习方法。(2)提出多层鉴别卷积稀疏表示学习方法,提高卷积稀疏编码方法的表示能力和鉴别能力。(3)探讨了字典学习和深度学习有效结合的机制,提出了字典驱动的深度表示学习方法。.本项目推动了稀疏表示学习理论和方法研究的发展,在提取小样本数据的高层特征表示方面有较大改善,为小样本数据处理提供了理论和方法支持。.
项目成果
期刊论文数量(10)
专著数量(0)
科研奖励数量(0)
会议论文数量(2)
专利数量(1)
Graph-Regularized Discriminative Analysis-Synthesis Dictionary Pair Learning for Image Classification
用于图像分类的图正则判别分析综合字典对学习
- DOI:10.1109/access.2019.2912932
- 发表时间:2019
- 期刊:IEEE Access
- 影响因子:3.9
- 作者:Chang Heyou;Tang Hui;Zhang Fanlong;Chen Yang;Zheng Hao
- 通讯作者:Zheng Hao
基于信息扩散与聚集的脱机手写字符重心定位方法
- DOI:--
- 发表时间:2020
- 期刊:山西大学学报(自然科学版)
- 影响因子:--
- 作者:王寅同;王燕清;肖文洁
- 通讯作者:肖文洁
Denoising Diffusion MRI via Graph Total Variance in Spatioangular Domain
通过空间角域图总方差对扩散 MRI 进行去噪
- DOI:10.1155/2021/4645544
- 发表时间:2021
- 期刊:Computational and Mathematical Methods in Medicine
- 影响因子:--
- 作者:Wu H;Yan S
- 通讯作者:Yan S
Cross-resolution face recognition with pose variations via multilayer locality-constrained structural orthogonal procrustes regression
通过多层局部约束结构正交 Procrustes 回归进行姿态变化的跨分辨率人脸识别
- DOI:10.1016/j.ins.2019.08.004
- 发表时间:2020
- 期刊:Information Sciences
- 影响因子:8.1
- 作者:Gao Guangwei;Yu Yi;Yang Meng;Chang Heyou;Huang Pu;Yue Dong
- 通讯作者:Yue Dong
Locality-constrained feature space learning for cross-resolution sketch-photo face recognition
用于跨分辨率草图照片人脸识别的局部约束特征空间学习
- DOI:10.1007/s11042-019-08488-y
- 发表时间:2020
- 期刊:Multimedia Tools and Applications
- 影响因子:3.6
- 作者:Gao Guangwei;Wang Yannan;Huang Pu;Chang Heyou;Lu Huimin;Yue Dong
- 通讯作者:Yue Dong
数据更新时间:{{ journalArticles.updateTime }}
{{
item.title }}
{{ item.translation_title }}
- DOI:{{ item.doi || "--"}}
- 发表时间:{{ item.publish_year || "--" }}
- 期刊:{{ item.journal_name }}
- 影响因子:{{ item.factor || "--"}}
- 作者:{{ item.authors }}
- 通讯作者:{{ item.author }}
数据更新时间:{{ journalArticles.updateTime }}
{{ item.title }}
- 作者:{{ item.authors }}
数据更新时间:{{ monograph.updateTime }}
{{ item.title }}
- 作者:{{ item.authors }}
数据更新时间:{{ sciAawards.updateTime }}
{{ item.title }}
- 作者:{{ item.authors }}
数据更新时间:{{ conferencePapers.updateTime }}
{{ item.title }}
- 作者:{{ item.authors }}
数据更新时间:{{ patent.updateTime }}
其他文献
其他文献
{{
item.title }}
{{ item.translation_title }}
- DOI:{{ item.doi || "--" }}
- 发表时间:{{ item.publish_year || "--"}}
- 期刊:{{ item.journal_name }}
- 影响因子:{{ item.factor || "--" }}
- 作者:{{ item.authors }}
- 通讯作者:{{ item.author }}
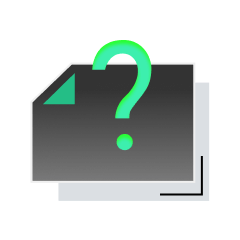
内容获取失败,请点击重试
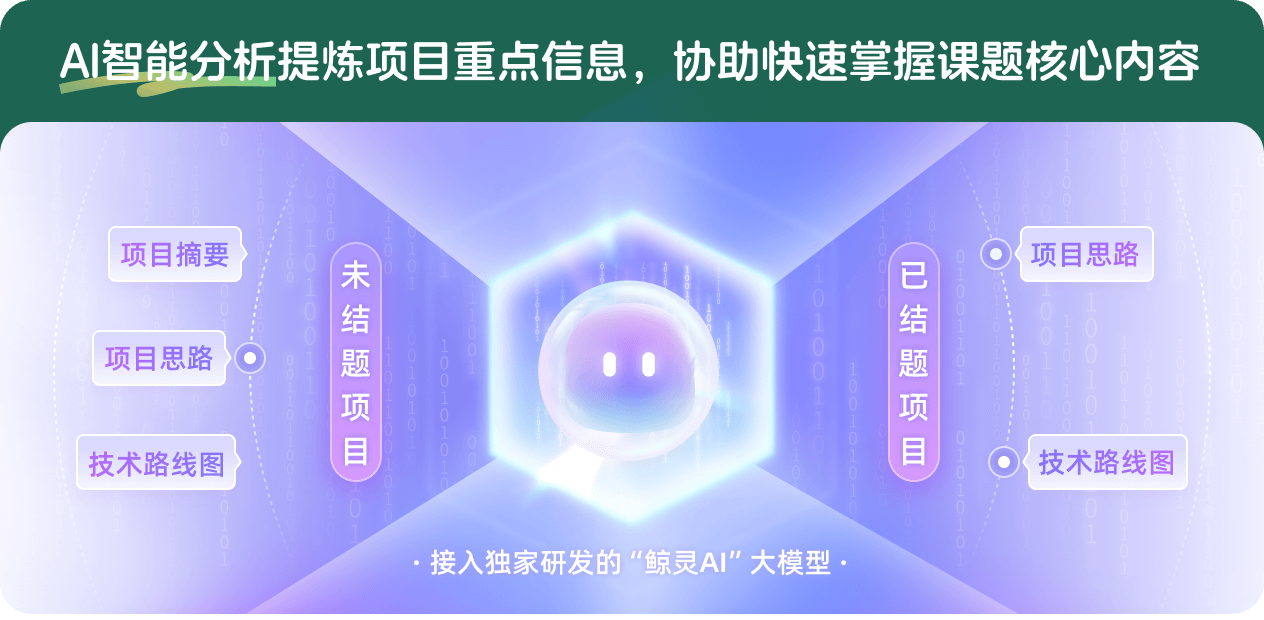
查看分析示例
此项目为已结题,我已根据课题信息分析并撰写以下内容,帮您拓宽课题思路:
AI项目摘要
AI项目思路
AI技术路线图
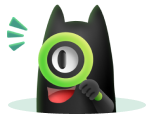
请为本次AI项目解读的内容对您的实用性打分
非常不实用
非常实用
1
2
3
4
5
6
7
8
9
10
您认为此功能如何分析更能满足您的需求,请填写您的反馈:
相似国自然基金
{{ item.name }}
- 批准号:{{ item.ratify_no }}
- 批准年份:{{ item.approval_year }}
- 资助金额:{{ item.support_num }}
- 项目类别:{{ item.project_type }}
相似海外基金
{{
item.name }}
{{ item.translate_name }}
- 批准号:{{ item.ratify_no }}
- 财政年份:{{ item.approval_year }}
- 资助金额:{{ item.support_num }}
- 项目类别:{{ item.project_type }}