数据驱动的混合逆问题cGANs回归及其在高光谱数据解混中的应用
项目介绍
AI项目解读
基本信息
- 批准号:61877066
- 项目类别:面上项目
- 资助金额:50.0万
- 负责人:
- 依托单位:
- 学科分类:F0610.交叉学科中的人工智能问题
- 结题年份:2022
- 批准年份:2018
- 项目状态:已结题
- 起止时间:2019-01-01 至2022-12-31
- 项目参与者:田小林; 朱虎明; 魏野; 郭贤鹏; 孟哲; 沈慧芳; 杨萌; 位冰可; 袁荣杰;
- 关键词:
项目摘要
Due to the rough spatial resolution, most of the pixels acquired by hyperspectral remote sensors are composed of several ground materials, which are well known as mixed pixels or mixtures. The widely presence of mixtures influences the performance of hyperspectral data classification and target recognition. Therefore, spectral unmixing, which extracts fractional abundance of pure materials (known as endmember) within a pixel, has been developed to solve such mixed-pixel problems. Regularization is a widely used technique throughout unmixing of hyperspectral data. From a computational perspective, regularization can lend stability to the optimization problem and can lead to algorithmic speed-ups. For decades, con¬structing regularization required careful engineering and considerable domain expertise to design a proper regularization term. In this project, the restricted isometry property (RIP) of the endmember matrix is studied in detail. The abundance vector reconstruction is treated as a regression problem mapping from spectral vector to abundance vector. The regression network is constructed via cGANs. Some abundance vectors and training samples can be obtained by a pre-unmixing. The basic requirement of the training samples is that the constructed mixed hyperspectral data should follow the same distribution with the real hyperspectral data. In addition, spatial correlation among pixels is considered in this project. It is assumed that spatial neighbors most likely possess a similar abundance vector as the testing pixel in the center of the neighborhood. However how to choose a proper size of the neighborhood is a key issue of the deep learning unmixing. Ensemble methods can thus reduce the risk of selecting the wrong model by aggregating all these candidate models. All the outputs of the deep learning unmixing network are put into a vector, and an ELM is trained to find a better result. Extensive experiment results, with both synthetic and real images, illustrate the generality and effectiveness of theses schemes.The research of this project will solve the nonlinear unmixing of hyperspectral data and promote the cross-integration between mathematics,machine learning and remote sensing data processing.
从空间分辨率低、地物谱信息动态变化的复杂高光谱数据中准确快速地提取地物目标的亚像元信息,是当前遥感领域的难题之一。本课题针对正则混合逆问题求解中的正则项构造难和正则优化问题求解速度慢等瓶颈问题,将混合逆问题转化为数据驱动的函数逼近问题。利用生成网络和判别网络的博弈对抗机制,建立高阶混合模型的cGANs回归架构,实现混合像元的模拟和隐函数逼近。面向高光谱数据解混,提出cGANs高光谱回归解混,突破端元提取、混合模式提取和表示、混合像元的生成、混合逆问题的网络回归等关键技术,最后通过合成数据、AVIRIS实测数据以及我国高分系列卫星的获取高光谱数据验证模型和方法有效性,打破了空间分辨率对高光谱数据解译的限制,推动数学、机器学习与遥感数据处理的交叉融合。
结项摘要
本课题针对正则逆问题求解中正则项构造难、混合优化问题求解速度慢以及样本手工标注费时费力等瓶颈问题,将解混转化为数据驱动的函数逼近问题,建立深度网络(AE,CNN、GAN和Transformer)逆问题回归模型,混合模式表示、混合像元的生成、混合逆问题的网络回归和小样本高光谱分类等关键技术方面取得突破性进展。.在高光谱图像解混方面,提出基于空间和光谱相似性的卷积神经网络(CNN)的高光谱解混、基于生成对抗网络(GAN)的高光谱解混、基于自编码网络的广义双线性模型解混、基于卷积神经网络的条件生成对抗网络空谱联合解混和基于Patch Transformer 的条件生成对抗网络空谱联合解混,利用生成网络和判别网络的博弈对抗机制以及先验知识,生成和实际高光谱数据同分布的训练样本,掘邻近高光谱混合像元丰度向量之间的相关性,最后借助深度网络强大的函数逼近能力逼近特征向量或谱向量与丰度向量之间的隐函数关系。在高光谱图像分类方面,提出基于生成对抗网络数据增强的高光谱图像分类算法和基于对抗表示学习的高光谱图像分类算法等方法,改进生成对抗网络进行,设计了基于辅助分类器的带梯度惩罚的生成对抗网络模型,通过条件熵引入无标签样本对模型进行半监督对抗训练,使编码器提取的特征更适合于分类任务。最后通过人工合成的高光谱数据和网上公开的AVIRIS 高光谱数据验证新模型和新方法的可行性和有效性。实验结果证明了所提算法相比于其他先进算法更有优势,尤其是在样本数目较少的情况下,这种优势更为明显。
项目成果
期刊论文数量(8)
专著数量(0)
科研奖励数量(0)
会议论文数量(1)
专利数量(5)
基于精英解和随机个体邻域信息的改进人工蜂群算法
- DOI:10.13195/j.kzyjc.2018.1797
- 发表时间:2020
- 期刊:控制与决策
- 影响因子:--
- 作者:孟红云;位冰可
- 通讯作者:位冰可
AC-WGAN-GP: Generating Labeled Samples for Improving Hyperspectral Image Classification with Small-Samples
AC-WGAN-GP:生成标记样本以改进小样本的高光谱图像分类
- DOI:10.3390/rs14194910
- 发表时间:2022-10
- 期刊:Remote Sensing
- 影响因子:5
- 作者:Caihao Sun;Xiaohua Zhang;Hongyun Meng;Xianghai Cao;Jinhua Zhang
- 通讯作者:Jinhua Zhang
Adversarial Representation Learning for Hyperspectral Image Classification with Small-Sized Labeled Set
小尺寸标记集高光谱图像分类的对抗性表示学习
- DOI:10.3390/rs14112612
- 发表时间:2022
- 期刊:Remote Sensing
- 影响因子:5
- 作者:Shuhan Zhang;Xiaohua Zhang;Tianrui Li;Hongyun Meng;Xianghai Cao;Li Wang
- 通讯作者:Li Wang
Hyperspectral Imagery Classification Based on Contrastive Learning
基于对比学习的高光谱图像分类
- DOI:10.1109/tgrs.2021.3139099
- 发表时间:2022
- 期刊:IEEE Transactions on Geoscience and Remote Sensing
- 影响因子:8.2
- 作者:Sikang Hou;Hongye Shi;Xianghai Cao;Xiaohua Zhang;Licheng Jiao
- 通讯作者:Licheng Jiao
数据更新时间:{{ journalArticles.updateTime }}
{{
item.title }}
{{ item.translation_title }}
- DOI:{{ item.doi || "--"}}
- 发表时间:{{ item.publish_year || "--" }}
- 期刊:{{ item.journal_name }}
- 影响因子:{{ item.factor || "--"}}
- 作者:{{ item.authors }}
- 通讯作者:{{ item.author }}
数据更新时间:{{ journalArticles.updateTime }}
{{ item.title }}
- 作者:{{ item.authors }}
数据更新时间:{{ monograph.updateTime }}
{{ item.title }}
- 作者:{{ item.authors }}
数据更新时间:{{ sciAawards.updateTime }}
{{ item.title }}
- 作者:{{ item.authors }}
数据更新时间:{{ conferencePapers.updateTime }}
{{ item.title }}
- 作者:{{ item.authors }}
数据更新时间:{{ patent.updateTime }}
其他文献
基于分子模拟技术的豨莶通栓制剂抗血小板聚集作用机制研究
- DOI:10.19540/j.cnki.cjcmm.20190111.005
- 发表时间:2019
- 期刊:中国中药杂志
- 影响因子:--
- 作者:赵博文;张小华;谷宇;赵帅;陈茜;雒银珍;张燕玲
- 通讯作者:张燕玲
酿酒酵母CHS3基因缺失对热耐受性的影响
- DOI:--
- 发表时间:--
- 期刊:中国酿造
- 影响因子:--
- 作者:刘向勇;王跃嗣;张小华
- 通讯作者:张小华
雄激素联合5型磷酸二酯酶抑制剂治疗大鼠糖尿病性勃起功能障碍的研究
- DOI:--
- 发表时间:2015
- 期刊:现代泌尿外科杂志
- 影响因子:--
- 作者:张小华;钟山;张敏光;沈周俊
- 通讯作者:沈周俊
Pt-CeO2/聚苯乙烯磺酸盐功能化碳纳米管复合物的制备及对甲醇的电催化氧化性能
- DOI:--
- 发表时间:2018
- 期刊:高等学校化学学报
- 影响因子:--
- 作者:陈晨;李丽;陈金华;张小华;许杰;李益波;韦杰
- 通讯作者:韦杰
掺锶聚磷酸钙对内皮细胞来源促血管化因子基质金属蛋白酶2表达的影响
- DOI:--
- 发表时间:--
- 期刊:中国组织工程研究与临床康复
- 影响因子:--
- 作者:邓晓薇;任大伟;余喜讯;万昌秀;张小华;刘菲
- 通讯作者:刘菲
其他文献
{{
item.title }}
{{ item.translation_title }}
- DOI:{{ item.doi || "--" }}
- 发表时间:{{ item.publish_year || "--"}}
- 期刊:{{ item.journal_name }}
- 影响因子:{{ item.factor || "--" }}
- 作者:{{ item.authors }}
- 通讯作者:{{ item.author }}
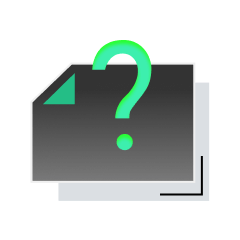
内容获取失败,请点击重试
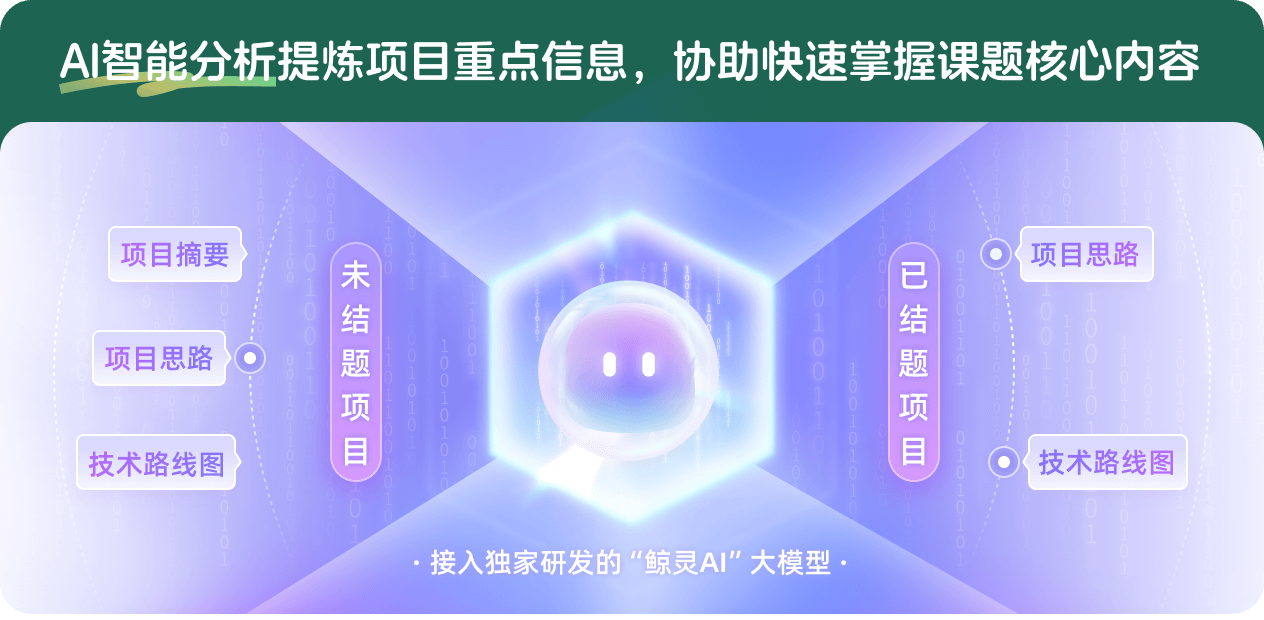
查看分析示例
此项目为已结题,我已根据课题信息分析并撰写以下内容,帮您拓宽课题思路:
AI项目摘要
AI项目思路
AI技术路线图
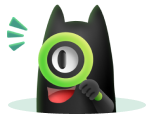
请为本次AI项目解读的内容对您的实用性打分
非常不实用
非常实用
1
2
3
4
5
6
7
8
9
10
您认为此功能如何分析更能满足您的需求,请填写您的反馈:
张小华的其他基金
基于Shearlet变换的图像分割和融合
- 批准号:60703109
- 批准年份:2007
- 资助金额:20.0 万元
- 项目类别:青年科学基金项目
相似国自然基金
{{ item.name }}
- 批准号:{{ item.ratify_no }}
- 批准年份:{{ item.approval_year }}
- 资助金额:{{ item.support_num }}
- 项目类别:{{ item.project_type }}
相似海外基金
{{
item.name }}
{{ item.translate_name }}
- 批准号:{{ item.ratify_no }}
- 财政年份:{{ item.approval_year }}
- 资助金额:{{ item.support_num }}
- 项目类别:{{ item.project_type }}