基于深度学习与声影校正的全乳房超声解剖层分割新方法研究
项目介绍
AI项目解读
基本信息
- 批准号:61501305
- 项目类别:青年科学基金项目
- 资助金额:19.0万
- 负责人:
- 依托单位:
- 学科分类:F0125.医学信息检测与处理
- 结题年份:2018
- 批准年份:2015
- 项目状态:已结题
- 起止时间:2016-01-01 至2018-12-31
- 项目参与者:李征毅; 林穆清; 张灵; 孟德明; 和晓念; 韩笑; 季兴;
- 关键词:
项目摘要
Whole breast ultrasound image can provide complete breast anatomic information and then potentially increase the detection rate of breast lesions. However, the reading and understanding of the whole breast image data is far more difficult than traditional B-mode ultrasound and may also lower down the efficiency of diagnostic procedure. To improve the efficiency of the clinical image reading and diagnosis, this study aims to develop an automatic segmentation method to partition the whole breast ultrasound into four layers of "fat", "mammary gland tissue", "muscle", and "rib/chest wall". The clinical applications of the segmentation of four layers can be at least twofold. First, the segmentation can provide quantitative volume measurements of the specific layers for the calculation of breast density. Second, the segmentation result can be served as the prior knowledge to exclude clinically implausible false-positive findings in the computerized breast lesion detection scheme for the 3D whole breast ultrasound. In such cases, the segmentation of the four breast anatomic layers is quite valuable in the clinical practice. The segmentation scheme is proposed to be realized in three steps: (1) construction of breast anatomic recognition models with deep learning techniques to identify each specific layer in the image as the pre-segmentation; (2) detection of the locations of acoustic shadows in the image; (3) accurate segmentation and shadow correction with the region competition framework that can be initialized with the results of steps (1) and (2). The first step employs the deep learning techniques to address the high intra-class variation problem in the pattern of each anatomic layer. The result of the first step can be served as a reliable pre-segmentation for the third step. The second step will provide the prior location knowledge of acoustic shadows to prevent over-correction in the normal regions in the process of shadow correction. The third step then adopts the iterative segmentation and shadow correction fashion to seek the satisfactory segmentation result with high accuracy. The segmentation of four anatomic layers in the whole breast ultrasound image is a brand new problem with the advance of this cutting-edge imaging technique. The corresponding research methods in this proposal are original and innovative. The research outcomes can potentially be shaped into a computerized tool for the analysis of the whole breast ultrasound images. Meanwhile, the research experience exploited in this proposal can be accumulated and shared as the referential knowledge for the future studies on image segmentation and pattern recognition in the 3D ultrasound image.
全乳房超声图像由于可提供完整的乳房解剖信息,而有潜力能大大提高病灶检出率。但是全乳房数据的图像判读诊断更加的困难,也使得诊断效率低下。为了提升全乳房超声图像的分析,本项目拟研发全自动乳房解剖层分割算法,将全乳超声分割为脂肪、乳腺组织、肌肉、肋骨与胸腔壁等四层。分割结果可用于计算乳房密度和减少计算机辅助乳腺病灶检测的假阳性,具有极大的临床价值。本项目拟采用以下步骤进行分割:1)使用深度学习技术进行四个解剖层的预分割,来对付解剖层样式高类内差异的问题;2)声影区域检测,提供声影位置先验知识,避免正常区域过度校正;3)基於步骤1-2的结果以区域竞争算法为框架,借由分割与声影校正迭代的手段,求得精确的分割结果。全乳超声解剖层分割为全新的课题,本项目提出的一系列研究方案具有原始初创性,可作为临床全乳超声分析工具,也可为三维超声图像分割与模式识别等科学问题提供知识积累。
结项摘要
全乳房超声图像由于可提供完整的乳房解剖信息,而有潜力能大大提高病灶检出率。但是全乳房数据的图像判读诊断更加的困难,也使得诊断效率低下。为了提升全乳房超声图像的分析,本项目拟研发全自动乳房解剖层分割算法,将全乳超声分割为脂肪、乳腺组织、肌肉、肋骨与胸腔壁等四层。分割结果可用于计算乳房密度和减少计算机辅助乳腺病灶检测的假阳性,具有极大的临床价值。本项目拟采用以下步骤进行分割:1)使用深度学习技术进行四个解剖层的预分割,来对付解剖层样式高类内差异的问题;2)声影区域检测,提供声影位置先验知识,避免正常区域过度校正;3)基於步骤1-2的结果以区域竞争算法为框架,借由分割与声影校正迭代的手段,求得精确的分割结果。全乳超声解剖层分割为全新的课题,本项目提出的一系列研究方案具有原始初创性,可作为临床全乳超声分析工具,也可为三维超声图像分割与模式识别等科学问题提供知识积累。
项目成果
期刊论文数量(20)
专著数量(0)
科研奖励数量(0)
会议论文数量(9)
专利数量(4)
Longitudinal Analysis for Disease Progression via Simultaneous Multi-Relational Temporal-Fused Learning.
通过同时多关系时间融合学习对疾病进展进行纵向分析
- DOI:10.3389/fnagi.2017.00006
- 发表时间:2017
- 期刊:Frontiers in aging neuroscience
- 影响因子:4.8
- 作者:Lei B;Jiang F;Chen S;Ni D;Wang T
- 通讯作者:Wang T
Automatic Categorization and Scoring of Solid, Part-Solid and Non-Solid Pulmonary Nodules in CT Images with Convolutional Neural Network.
利用卷积神经网络对 CT 图像中实性、部分实性和非实性肺部结节进行自动分类和评分
- DOI:10.1038/s41598-017-08040-8
- 发表时间:2017-09-01
- 期刊:Scientific reports
- 影响因子:4.6
- 作者:Tu X;Xie M;Gao J;Ma Z;Chen D;Wang Q;Finlayson SG;Ou Y;Cheng JZ
- 通讯作者:Cheng JZ
Automatic Fetal Head Circumference Measurement in Ultrasound Using Random Forest and Fast Ellipse Fitting
使用随机森林和快速椭圆拟合的超声自动胎头周长测量
- DOI:10.1109/jbhi.2017.2703890
- 发表时间:2018-01-01
- 期刊:IEEE JOURNAL OF BIOMEDICAL AND HEALTH INFORMATICS
- 影响因子:7.7
- 作者:Li, Jing;Wang, Yi;Ni, Dong
- 通讯作者:Ni, Dong
Computer-Aided Diagnosis with Deep Learning Architecture: Applications to Breast Lesions in US Images and Pulmonary Nodules in CT Scans.
采用深度学习架构的计算机辅助诊断:在 US 图像中的乳腺病变和 CT 扫描中的肺部结节中的应用
- DOI:10.1038/srep24454
- 发表时间:2016-04-15
- 期刊:Scientific reports
- 影响因子:4.6
- 作者:Cheng JZ;Ni D;Chou YH;Qin J;Tiu CM;Chang YC;Huang CS;Shen D;Chen CM
- 通讯作者:Chen CM
Multi-modal and multi-layout discriminative learning for placental maturity staging
胎盘成熟度分期的多模式和多布局判别学习
- DOI:10.1016/j.patcog.2016.09.037
- 发表时间:2017-03
- 期刊:Pattern Recognition
- 影响因子:8
- 作者:Baiying Lei;Wanjun Li;Yuan Yao;Xudong Jiang;Ee-Leng Tan;Jing Qin;Siping Chen
- 通讯作者:Siping Chen
数据更新时间:{{ journalArticles.updateTime }}
{{
item.title }}
{{ item.translation_title }}
- DOI:{{ item.doi || "--"}}
- 发表时间:{{ item.publish_year || "--" }}
- 期刊:{{ item.journal_name }}
- 影响因子:{{ item.factor || "--"}}
- 作者:{{ item.authors }}
- 通讯作者:{{ item.author }}
数据更新时间:{{ journalArticles.updateTime }}
{{ item.title }}
- 作者:{{ item.authors }}
数据更新时间:{{ monograph.updateTime }}
{{ item.title }}
- 作者:{{ item.authors }}
数据更新时间:{{ sciAawards.updateTime }}
{{ item.title }}
- 作者:{{ item.authors }}
数据更新时间:{{ conferencePapers.updateTime }}
{{ item.title }}
- 作者:{{ item.authors }}
数据更新时间:{{ patent.updateTime }}
其他文献
其他文献
{{
item.title }}
{{ item.translation_title }}
- DOI:{{ item.doi || "--" }}
- 发表时间:{{ item.publish_year || "--"}}
- 期刊:{{ item.journal_name }}
- 影响因子:{{ item.factor || "--" }}
- 作者:{{ item.authors }}
- 通讯作者:{{ item.author }}
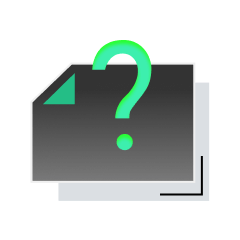
内容获取失败,请点击重试
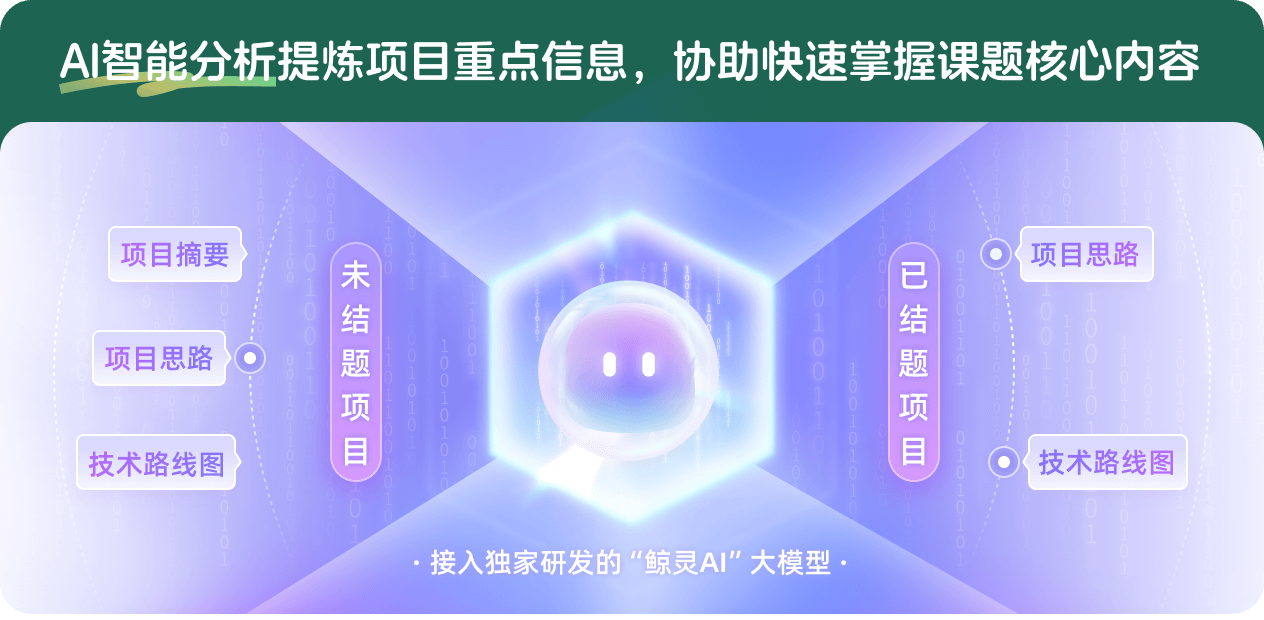
查看分析示例
此项目为已结题,我已根据课题信息分析并撰写以下内容,帮您拓宽课题思路:
AI项目摘要
AI项目思路
AI技术路线图
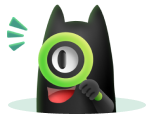
请为本次AI项目解读的内容对您的实用性打分
非常不实用
非常实用
1
2
3
4
5
6
7
8
9
10
您认为此功能如何分析更能满足您的需求,请填写您的反馈:
相似国自然基金
{{ item.name }}
- 批准号:{{ item.ratify_no }}
- 批准年份:{{ item.approval_year }}
- 资助金额:{{ item.support_num }}
- 项目类别:{{ item.project_type }}
相似海外基金
{{
item.name }}
{{ item.translate_name }}
- 批准号:{{ item.ratify_no }}
- 财政年份:{{ item.approval_year }}
- 资助金额:{{ item.support_num }}
- 项目类别:{{ item.project_type }}