自然刺激下的多维度与多尺度脑电信号处理方法研究
项目介绍
AI项目解读
基本信息
- 批准号:81471742
- 项目类别:面上项目
- 资助金额:62.0万
- 负责人:
- 依托单位:
- 学科分类:H2707.生物电磁成像
- 结题年份:2018
- 批准年份:2014
- 项目状态:已结题
- 起止时间:2015-01-01 至2018-12-31
- 项目参与者:常翼; 胡增春; JARMO HAMALAINEN; PETRI TOIVIAINEN; 马征; 牟真; 王程远; 郝亚娜;
- 关键词:
项目摘要
In order to decode human brain activity during real-world experiences, ongoing EEG can be measured as one of the important approaches, instead of spontaneous EEG and event-related potentials (ERPs). Nevertheless, how to process and analyze the ongoing EEG is still an open question. In this project, when healthy adults and patients listen to pieces of naturalistic music, ongoing EEG will be collected, and the long-term and short-term musical features of the naturalistic music stimuli will be extracted. Subsequently, the time-frequency representation of ongoing EEG in different time scales will be used to construct the high-order tensor (multi-way array) including the modes of time, frequency, space and subject. Then, nonnegative tensor factorization will be applied to decompose the high-order tensor. Finally, the extracted temporal components (which are significantly correlated with the musical features) will be selected, and the corresponding multi-domain features of ongoing EEG will be analyzed for drawing conclusions. Particularly, the new tensor clustering algorithm in the tensor space will be developed to evaluate the stability of tensor decomposition results; the new coupled tensor decomposition algorithm (based on maximizing correlation of latent variables between two modalities) will be developed for more effectively extracting the evoked potentials (elicited by the naturalistic music stimuli); and the developed ongoing EEG signal processing method will assist to exploit the underlying mechanism why the musical therapy can function. The proposed research in the project is significant for the brain science research during real-world experiences.
与事件相关电位(由短时且重复刺激诱发)和自发脑电图(EEG)不同,自然刺激(比如音乐和电影等)下的EEG成像是研究人在真实经验中大脑加工的重要手段。如何处理和分析这种EEG是该研究的难点。本课题将采集健康受试者和患者在听音乐时的EEG数据,并提取音乐的长时和短时特征;然后分别在秒与分钟的时间尺度下,基于EEG的时频表述数据构建高阶张量(亦即多维数组,包含时间、频率、空间和被试维度);进而用非负张量分解算法对高阶张量进行分解;最后选择与音乐特征显著相关的时域分量,分析该分量所对应的 EEG多域特征,从而得出结论。特别地,发展张量空间的聚类方法来判定张量分解结果的稳定性;发展基于双模态数据(音乐和EEG)潜变量间相关性最大化的联合张量分解算法,从而更有效地提取出音乐所诱发的电位;为探讨音乐治疗发挥治疗作用的脑电生理机制提供技术支持。这些工作对通过EEG进行自然刺激下的脑科学研究有学术意义。
结项摘要
与事件相关电位(由短时且重复刺激诱发)和自发脑电图(EEG)不同,自然刺激(比如音乐和电影等)下的EEG成像是研究人在真实经验中大脑加工的重要手段。如何处理和分析这种EEG是该研究的难点。本课题采集健康受试者和患者在听音乐时的EEG数据,并提取音乐的长时和短时特征;然后分别在分钟的时间尺度下,基于EEG的时频表述数据构建高阶张量(亦即多维数组,包含时间、频率、空间和被试维度);进而采用非负张量分解算法对高阶张量进行分解,为了能够保证张量分解的收敛性和稳定性,我们采用一种最新的alternating proximal gradient (APG) 数值优化计算方法进行求解,得到了理想的结果;最后选择与音乐特征显著相关的时域分量,分析该分量所对应的 EEG多域特征,从而找到了与自然刺激诱发的大脑活动。特别地,发展张量空间(基于系数矩阵和成分矩阵形成的秩一张量)的聚类方法来进一步判定张量和矩阵分解结果的稳定性。为探讨音乐治疗发挥治疗作用的脑电生理机制提供技术支持。这些工作对通过EEG进行自然刺激下的脑科学研究有学术意义。
项目成果
期刊论文数量(11)
专著数量(2)
科研奖励数量(0)
会议论文数量(0)
专利数量(0)
Adaptive independent vector analysis for multi-subject complex-valued fMRI data
多对象复值功能磁共振成像数据的自适应独立向量分析
- DOI:10.1016/j.jneumeth.2017.01.017
- 发表时间:2017
- 期刊:Journal of Neuroscience Methods
- 影响因子:3
- 作者:Kuang Lidan;Lin Qiuhua;Gong Xiaofeng;Cong Fengyu;Calhoun Vince D.
- 通讯作者:Calhoun Vince D.
Extracting multi-mode ERP features using fifth-order nonnegative tensor decomposition
使用五阶非负张量分解提取多模式 ERP 特征
- DOI:10.1016/j.jneumeth.2018.07.020
- 发表时间:2018-10-01
- 期刊:JOURNAL OF NEUROSCIENCE METHODS
- 影响因子:3
- 作者:Wang, Deqing;Zhu, Yongjie;Cong, Fengyu
- 通讯作者:Cong, Fengyu
Examining stability of independent component analysis based on coefficient and component matrices for voxel-based morphometry of structural magnetic resonance imaging
检查基于系数和分量矩阵的独立分量分析的稳定性,用于基于体素的结构磁共振成像形态测量
- DOI:10.1007/s11571-018-9484-2
- 发表时间:2018-03
- 期刊:COGNITIVE NEURODYNAMICS
- 影响因子:3.7
- 作者:Zhang Qing;Hu Guoqiang;Tian Lili;Ristaniemi Tapani;Wang Huili;Chen Hongjun;Wu Jianlin;Cong Fengyu
- 通讯作者:Cong Fengyu
Tensor decomposition of EEG signals: A brief review
脑电图信号的张量分解:简要回顾
- DOI:10.1016/j.jneumeth.2015.03.018
- 发表时间:2015-06-15
- 期刊:JOURNAL OF NEUROSCIENCE METHODS
- 影响因子:3
- 作者:Cong, Fengyu;Lin, Qiu-Hua;Ristaniemi, Tapani
- 通讯作者:Ristaniemi, Tapani
Multi-subject fMRI analysis via combined independent component analysis and shift-invariant canonical polyadic decomposition
通过独立成分分析和平移不变正则多元分解相结合的多受试者功能磁共振成像分析
- DOI:10.1016/j.jneumeth.2015.08.023
- 发表时间:2015
- 期刊:Journal of Neuroscience Methods
- 影响因子:3
- 作者:Li-Dan Kuang;Qiu-Hua Lin;Xiao-Feng Gong;Fengyu Cong;Jing Sui;Vince D. Calhoun
- 通讯作者:Vince D. Calhoun
数据更新时间:{{ journalArticles.updateTime }}
{{
item.title }}
{{ item.translation_title }}
- DOI:{{ item.doi || "--"}}
- 发表时间:{{ item.publish_year || "--" }}
- 期刊:{{ item.journal_name }}
- 影响因子:{{ item.factor || "--"}}
- 作者:{{ item.authors }}
- 通讯作者:{{ item.author }}
数据更新时间:{{ journalArticles.updateTime }}
{{ item.title }}
- 作者:{{ item.authors }}
数据更新时间:{{ monograph.updateTime }}
{{ item.title }}
- 作者:{{ item.authors }}
数据更新时间:{{ sciAawards.updateTime }}
{{ item.title }}
- 作者:{{ item.authors }}
数据更新时间:{{ conferencePapers.updateTime }}
{{ item.title }}
- 作者:{{ item.authors }}
数据更新时间:{{ patent.updateTime }}
其他文献
改进的卷积神经网络实现端到端的水下目标自动识别
- DOI:10.16798/j.issn.1003-0530.2020.06.018
- 发表时间:2020
- 期刊:信号处理
- 影响因子:--
- 作者:王小宇;李凡;曹琳;李军;张驰;彭圆;丛丰裕
- 通讯作者:丛丰裕
其他文献
{{
item.title }}
{{ item.translation_title }}
- DOI:{{ item.doi || "--" }}
- 发表时间:{{ item.publish_year || "--"}}
- 期刊:{{ item.journal_name }}
- 影响因子:{{ item.factor || "--" }}
- 作者:{{ item.authors }}
- 通讯作者:{{ item.author }}
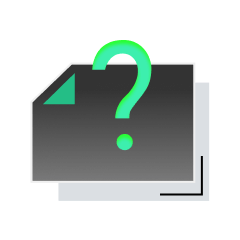
内容获取失败,请点击重试
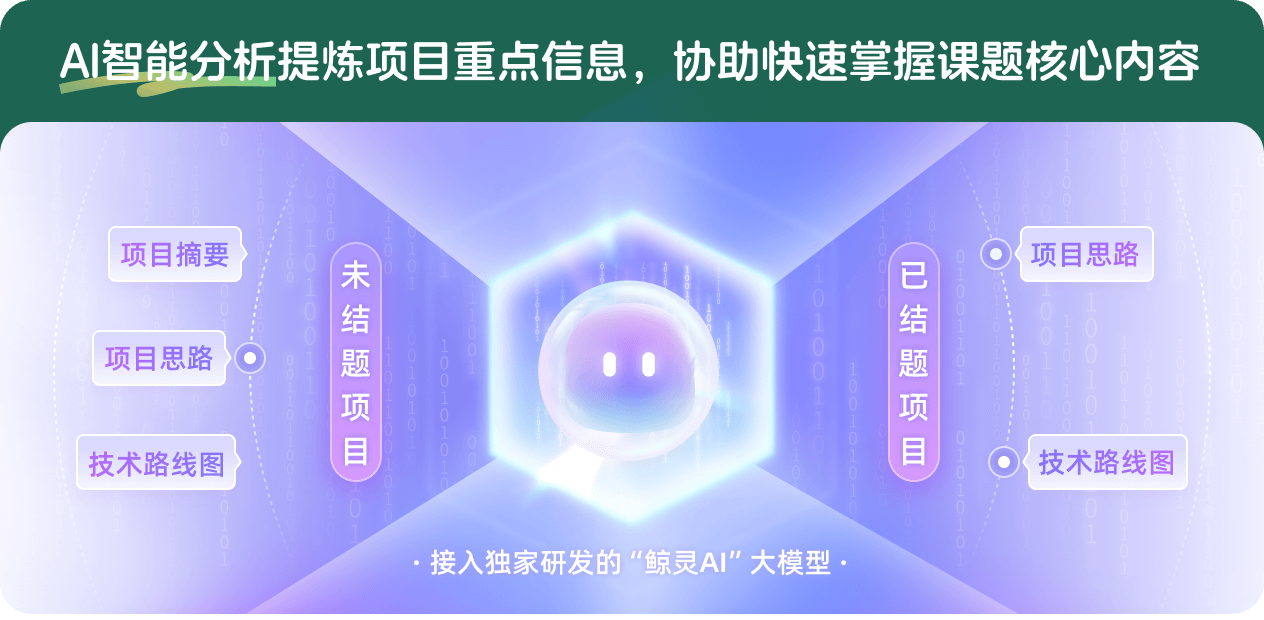
查看分析示例
此项目为已结题,我已根据课题信息分析并撰写以下内容,帮您拓宽课题思路:
AI项目摘要
AI项目思路
AI技术路线图
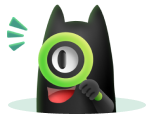
请为本次AI项目解读的内容对您的实用性打分
非常不实用
非常实用
1
2
3
4
5
6
7
8
9
10
您认为此功能如何分析更能满足您的需求,请填写您的反馈:
丛丰裕的其他基金
基于声呐操作员大脑识别声信号神经机制特性的水下目标特征提取与自动识别研究
- 批准号:91748105
- 批准年份:2017
- 资助金额:63.0 万元
- 项目类别:重大研究计划
相似国自然基金
{{ item.name }}
- 批准号:{{ item.ratify_no }}
- 批准年份:{{ item.approval_year }}
- 资助金额:{{ item.support_num }}
- 项目类别:{{ item.project_type }}
相似海外基金
{{
item.name }}
{{ item.translate_name }}
- 批准号:{{ item.ratify_no }}
- 财政年份:{{ item.approval_year }}
- 资助金额:{{ item.support_num }}
- 项目类别:{{ item.project_type }}