根据COSMIN标准研制大骨节病患者生命质量量表及其实证研究
项目介绍
AI项目解读
基本信息
- 批准号:81302393
- 项目类别:青年科学基金项目
- 资助金额:23.0万
- 负责人:
- 依托单位:
- 学科分类:H3013.地方病学
- 结题年份:2016
- 批准年份:2013
- 项目状态:已结题
- 起止时间:2014-01-01 至2016-12-31
- 项目参与者:李小妹; 王小琴; 王娣妙; 王森; 夏传涛; 张迎; 鱼星峰;
- 关键词:
项目摘要
Kashin-Beck disease (KBD) is an endemic osteoarthropathy with high prevalence, high deformity and disability rate,which is the most serious condition in the resouce-limited western part of China. The disease influences patients' quality of life and labor productivity, and most severiously restrictes the local social economic development. The current evaluation instruments can not measure the patients' quality of life and treatment effect comprehensively and scientifically, not to mention the reflection of the disease's characteristics and the patients' psychosocial condition. Therefore, the purpose of this study is to develop a scientific, effective and practical KBD quality of life instrument under the guidance of the latest international "the consensus-based standards for the selection of health measurement instruments (COSMIN)", adopting the model of "the special scale combined with the common scale", and with the considering the actual situation of KBD in China. The research innovatively aims at three contents: ① developing a KBD quality of life instrument that reflects patients' characteristic, and adapts to Chinese culture, ② measuring the reliability, validity and responsiveness of the instrument, ③ evaluating the quality of life of KBD patients and its influencing factors. The results will provide theoretical and practical bases for developing a evaluation and treatment system for KBD, a theoretical evidence for the benefit analysis of health resources allocation, which will ultimately promote the prevention and control of KBD.
大骨节病是一种患病率和致残率很高的地方性、畸形性骨关节病,目前以经济相对落后的中国西部地区病情最为严重,不但严重影响了患者生命质量和劳动生产力,且制约了当地经济发展。现有的测评工具不能全面反映大骨节病的特征和患者社会心理特点,更无法评价大骨节病患者治疗效果和生命质量。因此,本研究以国际最新"发展生命质量量表标准(COSMIN) "为依据,采用"特异量表与共性量表相结合"的模式,根据我国社会经济特点及患者实际情况,研制科学、有效、实用的大骨节病患者生命质量量表,主要内容为:① 研制能反映大骨节病特征及患者社会心理特点大骨节病患者生命质量量表,②确定该量表的信度、效度和反应度,③测评大骨节病患者生命质量及影响因素。为大骨节病患者干预措施形成评价体系、筛选治疗方案及分析卫生资源的投入效益提供理论依据和测评工具,提高我国大骨节病的防治水平。
结项摘要
大骨节病是一种患病率和致残率很高的地方性、畸形性骨关节病,目前以经济相对落后的中国西部地区病情最为严重,不但严重影响了患者生命质量和劳动生产力,且制约了当地经济发展。现有的测评工具不能全面反映大骨节病的特征和患者社会心理特点,更无法评价大骨节病患者治疗效果和生命质量。因此,本研究以国际最新“发展生命质量量表标准(COSMIN) ”为依据,采用“特异量表与共性量表相结合”的模式,根据我国社会经济特点及患者实际情况,研制科学、有效、实用的大骨节病患者生命质量量表,主要内容为:① 研制能反映大骨节病特征及患者社会心理特点大骨节病患者生命质量量表,②确定该量表的信度、效度和反应度,③测评大骨节病患者生命质量及影响因素。.大骨节病患者生命质量量表(KBDQOL)-特异量表,已经依据COSMIN标准,世界卫生组织生命质量结构框架并结合大骨节病患者实际情况研制出来。研制该工具的第一步是组织公共卫生专家和大骨节病患者讨论生命质量的概念框架,接着通过深度访谈20名大骨节病患者和8名大骨节病专家、4个焦点访谈小组及文献回顾产生条目池KBDQOL1.1,第二步是用KBDQOL1.1 调查368名大骨节病患者,10-14天后重测95名大骨节病患者。结果通过因子分析得到含有6个维度共28个条目的KBDQOL1.2。6个维度是:躯体功能(7个条目)、活动限制(5个条目)、社会支持(4个条目)、经济(3个条目)、精神健康(5个条目)、总的健康(4个条目)。各维度的条目内部一致性系数(Cronbach’s alpha)范围为0.77-0.89,重测信度(ICC)范围为0.73-0.90,各维度最小可探测改变范围在个体水平为13.2-30.2分,在群体水平范围为1.4-3.1分。结构效度较好。因此KBDQOL1.2是一个能评价大骨节病治疗效果的科学、有效、实用的新工具。.本项目研究者用欧洲五维健康量表(EQ-5D)中文版测量大骨节病患者得到的重测信度(Kappa values)范围为0.324-0.554,EQ-VAS的重测信度(ICC)为0.497。结构效度已得到验证。并且EQ-5D只有5个条目,使用方便,容易被大骨节病患者理解。因此可作为一般工具(共性量表)用于患者的生命质量测量。.研究结果为大骨节病患者干预措施形成评价体系、筛选治疗方案及分析卫生资源的投入效益提供了理论依据和测评工具.
项目成果
期刊论文数量(4)
专著数量(0)
科研奖励数量(0)
会议论文数量(0)
专利数量(0)
Reliability and validity of the EQ?5D?3L for Kashin–Beck disease in China
中国大骨节病EQ、5D、3L的信度和效度
- DOI:--
- 发表时间:2016
- 期刊:SpringerPlus
- 影响因子:--
- 作者:Wang Di-miao;Yu Fang-fang;Younus MI;Guo Xiong
- 通讯作者:Guo Xiong
生命质量及肢体残疾量表在评估大骨节病患者中的应用进展
- DOI:--
- 发表时间:2014
- 期刊:中华地方病学杂志
- 影响因子:--
- 作者:余方方;方华;郭雄
- 通讯作者:郭雄
大骨节病患者生命质量量表的介绍 及使用指南
- DOI:--
- 发表时间:2014
- 期刊:中华地方病学杂志
- 影响因子:--
- 作者:方华;郭雄;夏传涛;王娣妙
- 通讯作者:王娣妙
Evaluation of the therapeutic effect of treatment with intra-articular hyaluronic acid in knees for Kashin-Beck disease: A meta-analysis
膝关节腔内注射透明质酸治疗大骨节病的疗效评价:荟萃分析
- DOI:--
- 发表时间:2014
- 期刊:Osteoarthritis and Cartilage
- 影响因子:7
- 作者:Fang; H.;Han; J.;Younus; M. I.;Guo; X.
- 通讯作者:X.
数据更新时间:{{ journalArticles.updateTime }}
{{
item.title }}
{{ item.translation_title }}
- DOI:{{ item.doi || "--"}}
- 发表时间:{{ item.publish_year || "--" }}
- 期刊:{{ item.journal_name }}
- 影响因子:{{ item.factor || "--"}}
- 作者:{{ item.authors }}
- 通讯作者:{{ item.author }}
数据更新时间:{{ journalArticles.updateTime }}
{{ item.title }}
- 作者:{{ item.authors }}
数据更新时间:{{ monograph.updateTime }}
{{ item.title }}
- 作者:{{ item.authors }}
数据更新时间:{{ sciAawards.updateTime }}
{{ item.title }}
- 作者:{{ item.authors }}
数据更新时间:{{ conferencePapers.updateTime }}
{{ item.title }}
- 作者:{{ item.authors }}
数据更新时间:{{ patent.updateTime }}
其他文献
乳化炸药中空功能微囊的制备方法及性能表征
- DOI:10.1039/9781782620280-00032
- 发表时间:2019
- 期刊:含能材料
- 影响因子:--
- 作者:程扬帆;方华;刘文近;韩体飞;龚悦;陶臣;姚雨乐;沈兆武
- 通讯作者:沈兆武
镍表面修饰活性炭的电容性能
- DOI:10.3390/universe8030147
- 发表时间:2018
- 期刊:电池
- 影响因子:--
- 作者:方华;孟浩阳;丰小华;王力臻
- 通讯作者:王力臻
毒死蜱降解菌株Bacillus lateros
- DOI:10.1017/cbo9781107587977.006
- 发表时间:--
- 期刊:土壤学报,2006. 43: 648-654.
- 影响因子:--
- 作者:王 晓;楚小强;虞云龙;方华
- 通讯作者:方华
Co3O4/碳纳米管复合膜的超级电容器性能
- DOI:10.3969/j.issn.1001⁃3849.2020.02.001
- 发表时间:2020
- 期刊:电镀与精饰
- 影响因子:--
- 作者:张永霞;王玫;方华;程顺要;王嘉辉
- 通讯作者:王嘉辉
腐殖酸对水中多壁碳纳米管凝聚特性的影响
- DOI:--
- 发表时间:2015
- 期刊:中国环境科学
- 影响因子:--
- 作者:方华;孙宇心;于江华;方若雨;荆洁
- 通讯作者:荆洁
其他文献
{{
item.title }}
{{ item.translation_title }}
- DOI:{{ item.doi || "--" }}
- 发表时间:{{ item.publish_year || "--"}}
- 期刊:{{ item.journal_name }}
- 影响因子:{{ item.factor || "--" }}
- 作者:{{ item.authors }}
- 通讯作者:{{ item.author }}
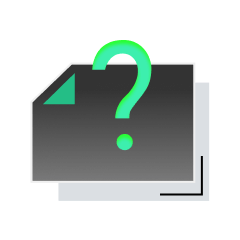
内容获取失败,请点击重试
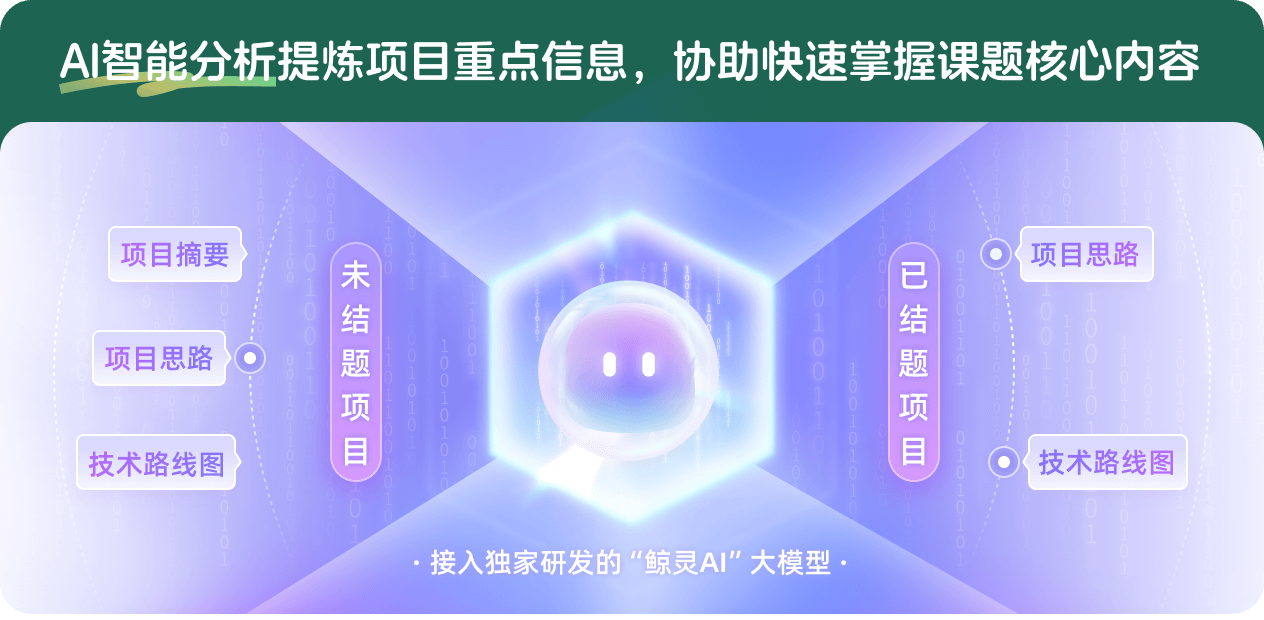
查看分析示例
此项目为已结题,我已根据课题信息分析并撰写以下内容,帮您拓宽课题思路:
AI项目摘要
AI项目思路
AI技术路线图
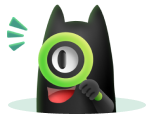
请为本次AI项目解读的内容对您的实用性打分
非常不实用
非常实用
1
2
3
4
5
6
7
8
9
10
您认为此功能如何分析更能满足您的需求,请填写您的反馈:
相似国自然基金
{{ item.name }}
- 批准号:{{ item.ratify_no }}
- 批准年份:{{ item.approval_year }}
- 资助金额:{{ item.support_num }}
- 项目类别:{{ item.project_type }}
相似海外基金
{{
item.name }}
{{ item.translate_name }}
- 批准号:{{ item.ratify_no }}
- 财政年份:{{ item.approval_year }}
- 资助金额:{{ item.support_num }}
- 项目类别:{{ item.project_type }}