大规模动态模态分解中的张量方法
项目介绍
AI项目解读
基本信息
- 批准号:11801479
- 项目类别:青年科学基金项目
- 资助金额:22.0万
- 负责人:
- 依托单位:
- 学科分类:A0405.连续优化
- 结题年份:2021
- 批准年份:2018
- 项目状态:已结题
- 起止时间:2019-01-01 至2021-12-31
- 项目参与者:林学磊; 刘金杰;
- 关键词:
项目摘要
This project aims at investigating the tensor methods in the large-scale dynamic mode decomposition (DMD) systematically. DMD is an important data-driven method in data science, which models and controls the dynamical systems hidden behind the sample data by spatiotemporal coherent decompositions. DMD is widely applied to fluid dynamics, neuroscience, computer vision, financial market, epidemiology, etc. Nevertheless, many current problems cannot be solved by DMD and its existing modifications, since the dimension and the complexity of data increase tremendously. Therefore, we introduce the tensor methods in the large-scale DMD. We focus on the following three models in this project: (1) We consider the DMD of the data embedded into a tensor with Hankel structures, which is modelled by a bi-convex tensor optimization problem with linear constraints; (2) We assume that the evolution function in DMD can be represented by a tensor with a hierarchical Tucker decomposition, which is modelled by a tensor least squares problem with tensor rank and hierarchical Tucker constraints and closely related to deep learning; (3) We assume that the evolution in DMD can be represented by a sparse higher-order Markov chain, which is modelled by a sparse tensor optimization problem with linear constraints. For all these three models, we would like to make sufficient use of the structures in the involved tensors to design efficient and effective algorithms for the large-scale problems.
本项目系统研究大规模动态模态分解(DMD)中的张量方法。在数据科学中,DMD是重要的“数据驱动”方法,通过时空相干的分解,对数据背后动力系统进行建模和控制,被广泛应用于流体动力系统、神经科学、计算机视觉、金融市场、流行病学等领域。随着数据规模和复杂度的增加,许多问题无法用现有的DMD及其变型来解决。因此,我们将张量方法引入大规模数据的DMD。内容包括:(1) 将数据嵌入具有Hankel结构的张量再进行动态模态分解,考虑非均匀采样和数据丢失,可写成带线性约束的双凸张量优化问题;(2) 假设DMD中演化函数可由分层Tucker分解的张量表示,则可写成带张量秩约束与分层结构等非线性约束的张量最小二乘问题并与深度学习紧密相连;(3) 假设DMD中演化过程可以表示为稀疏高阶Markov链,则可写成带线性约束的稀疏张量优化问题。我们要充分利用所涉及张量的结构,针对大规模问题,设计快速、有效的算法。
结项摘要
本项目系统研究大规模动态模态分解中张量方法的算法、理论和应用。在数据科学中,动态模态分解是重要的“数据驱动”方法,通过时空相干的分解,对数据背后动力系统进行建模和控制,被广泛应用于流体动力系统、神经科学、计算机视觉、金融市场、流行病学等领域。随着数据规模和复杂度的增加,许多问题无法用现有分解算法来解决。因此,我们将张量方法引入大规模数据的动态模态分解,并对其中的优化问题、张量理论等进行了深入的研究。主要成果包括:(1) 大规模优化算法设计和张量优化问题的理论研究:这部分研究内容是本课题的基础,我们利用数值代数中适合求解鞍点问题的厄米特-反厄米特分裂方法的启发,设计新的求解凸优化问题的算子分裂算法,称为单调-反对称分裂方法,在较弱的条件下可以保证对具有任意多块的凸优化问题的收敛性。还有对还有弹性M-结构等的结构张量的理论做了系统的阐述和研究。(2) 高阶广义动态模态分解:我们提出的基于Hankel结构化整体最小二乘的高阶广义动态模态分解,可以处理谱复杂度高于空间复杂度的系统、在多条轨道上采样的情形,将噪声影响分配到模型中的所有部分提高了算法鲁棒性。进一步,我们将基于结构化整体最小二乘的高阶广义动态模态分解算法应用到了高阶Markov链、脑科学fMRI信号的分析中。(3) 动态模态分解中的四元数计算:四元数矩阵可以看成是特殊的张量。基于四元数的动态模态分解,主要是运用在RGB图像和视频以及向量场数据的分析中。其中涉及到如何从计算结果中提取视频,即对四元数矩阵作纯虚的低秩逼近,我们提出了交替投影方法,利用流形优化的技术和知识,证明了该交替投影算法的概率意义下的收敛性,并提出了利用算子分裂算法的初值点选取策略。(4) 相关分解在深度学习中的应用:我们利用本项目中的低秩分解的思想,通过引入张量skeleton分解,解决了基于注意力机制的Transformer算法的存储和计算消耗的问题。
项目成果
期刊论文数量(4)
专著数量(0)
科研奖励数量(0)
会议论文数量(0)
专利数量(0)
Low rank pure quaternion approximation for pure quaternion matrices
纯四元数矩阵的低秩纯四元数近似
- DOI:doi.10.1137/19m1307329
- 发表时间:2021
- 期刊:SIAM Journal on Matrix Analysis and Applications
- 影响因子:1.5
- 作者:Guangjing Song;Weiyang Ding;Michael K. Ng
- 通讯作者:Michael K. Ng
Acceptable Solutions and Backward Errors for Tensor Complementarity Problems
张量互补问题的可接受解和后向误差
- DOI:10.1007/s10957-020-01774-y
- 发表时间:2021
- 期刊:Journal of Optimization Theory and Applications
- 影响因子:1.9
- 作者:Shouqiang Du;Weiyang Ding;Yimin Wei
- 通讯作者:Yimin Wei
Elasticity M-tensors and the strong ellipticity condition
弹性 M 张量和强椭圆条件
- DOI:10.1016/j.amc.2019.124982
- 发表时间:2020
- 期刊:Applied Mathematics and Computation
- 影响因子:4
- 作者:Weiyang Ding;Jinjie Liu;Liqun Qi;Hong Yan
- 通讯作者:Hong Yan
A Peaceman-Rachford Splitting Method with Monotone Plus Skew-Symmetric Splitting for Nonlinear Saddle Point Problems
非线性鞍点问题的单调加斜对称分裂的Peaceman-Rachford分裂法
- DOI:--
- 发表时间:2019
- 期刊:J. Sci. Comput.
- 影响因子:--
- 作者:Weiyang Ding;Michael K. Ng;Wenxing Zhang
- 通讯作者:Wenxing Zhang
数据更新时间:{{ journalArticles.updateTime }}
{{
item.title }}
{{ item.translation_title }}
- DOI:{{ item.doi || "--"}}
- 发表时间:{{ item.publish_year || "--" }}
- 期刊:{{ item.journal_name }}
- 影响因子:{{ item.factor || "--"}}
- 作者:{{ item.authors }}
- 通讯作者:{{ item.author }}
数据更新时间:{{ journalArticles.updateTime }}
{{ item.title }}
- 作者:{{ item.authors }}
数据更新时间:{{ monograph.updateTime }}
{{ item.title }}
- 作者:{{ item.authors }}
数据更新时间:{{ sciAawards.updateTime }}
{{ item.title }}
- 作者:{{ item.authors }}
数据更新时间:{{ conferencePapers.updateTime }}
{{ item.title }}
- 作者:{{ item.authors }}
数据更新时间:{{ patent.updateTime }}
其他文献
Acceptable solutions and backward errors for tensor complementarity problems
张量互补问题的可接受解和后向误差
- DOI:10.1007/s10957-020-01774-y
- 发表时间:2020
- 期刊:Journal of Optimization Theory and Applications
- 影响因子:1.9
- 作者:杜守强;丁维洋;魏益民
- 通讯作者:魏益民
Computing the p-Spectral Radii of Uniform Hypergraphs with Applications
计算均匀超图的 p 谱半径及其应用
- DOI:10.1007/s10915-017-0520-x
- 发表时间:2018
- 期刊:Journal of Scientific Computing
- 影响因子:2.5
- 作者:常静雅;丁维洋;祁力群;Yan Hong
- 通讯作者:Yan Hong
其他文献
{{
item.title }}
{{ item.translation_title }}
- DOI:{{ item.doi || "--" }}
- 发表时间:{{ item.publish_year || "--"}}
- 期刊:{{ item.journal_name }}
- 影响因子:{{ item.factor || "--" }}
- 作者:{{ item.authors }}
- 通讯作者:{{ item.author }}
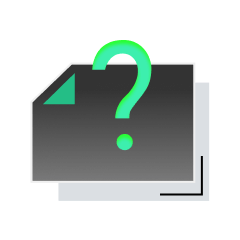
内容获取失败,请点击重试
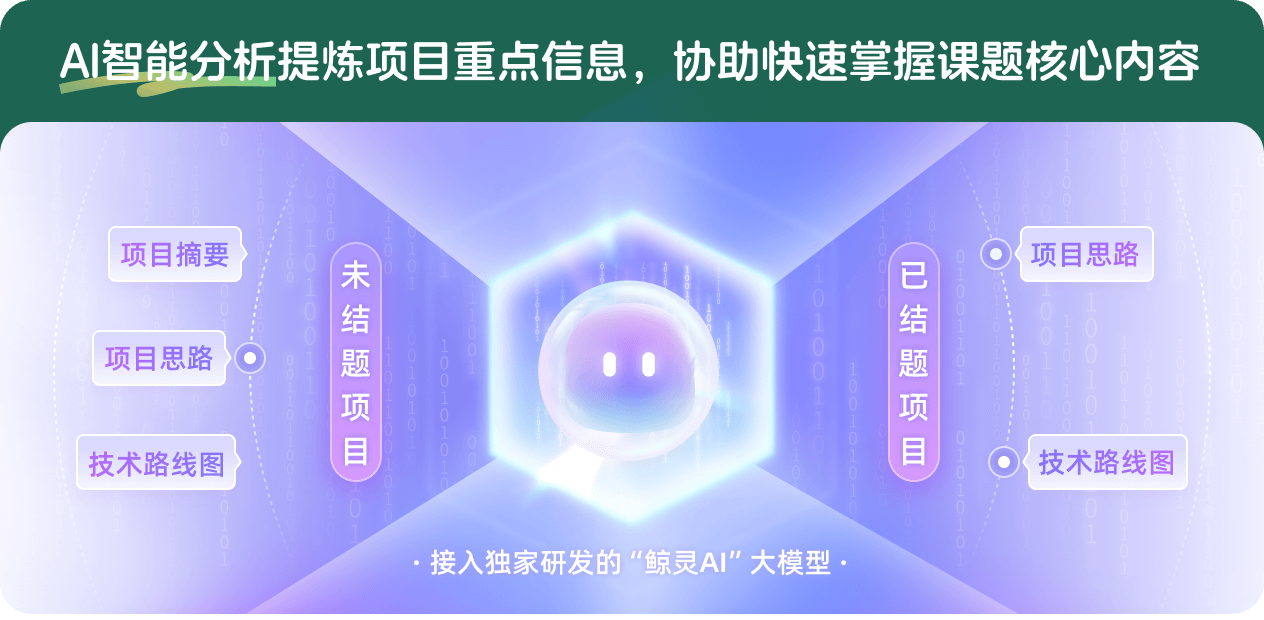
查看分析示例
此项目为已结题,我已根据课题信息分析并撰写以下内容,帮您拓宽课题思路:
AI项目摘要
AI项目思路
AI技术路线图
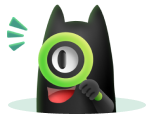
请为本次AI项目解读的内容对您的实用性打分
非常不实用
非常实用
1
2
3
4
5
6
7
8
9
10
您认为此功能如何分析更能满足您的需求,请填写您的反馈:
相似国自然基金
{{ item.name }}
- 批准号:{{ item.ratify_no }}
- 批准年份:{{ item.approval_year }}
- 资助金额:{{ item.support_num }}
- 项目类别:{{ item.project_type }}
相似海外基金
{{
item.name }}
{{ item.translate_name }}
- 批准号:{{ item.ratify_no }}
- 财政年份:{{ item.approval_year }}
- 资助金额:{{ item.support_num }}
- 项目类别:{{ item.project_type }}