跨模态的多语种新闻热点人物标识方法研究
项目介绍
AI项目解读
基本信息
- 批准号:61902301
- 项目类别:青年科学基金项目
- 资助金额:28.0万
- 负责人:
- 依托单位:
- 学科分类:F0210.计算机图像视频处理与多媒体技术
- 结题年份:2022
- 批准年份:2019
- 项目状态:已结题
- 起止时间:2020-01-01 至2022-12-31
- 项目参与者:--
- 关键词:
项目摘要
News character identification plays an important role in news comprehension analysis and retrieval. Multiple name-multiple face correlation analysis is a key and difficult problem for large-scale online automatic identification news character. The name and face are the main aspects of personal identity, which carrying the text and image information of the news respectively. This project intends to use the co-occurrence information between the image and the text to carry out similarity measure and semantic analysis of cross-modality, and research on the automatic identification of hot people in multi-lingual news. The main innovative research of the project includes: 1) For the problem of name annotation in multi-lingual network news, the project researches on using the context information of news person name to establish a high recognition rate model of name annotation, and construct a high quality model of name translation based on the trait of multi-lingual news characters. 2) Aiming at solving the problem of face representation in the large-scale network news, the project proposes an optimization method of face feature representation by jointing recurrent convolutional neural network and local Gabor binary pattern histogram sequence, for reducing the false accept rate and false reject rate. 3) For the problem of multiple name-multiple face correlation modeling, this project studies the complementarity of multi-source information in multi-lingual news to construct a name theme network based on cross-modality, and enhances the accuracy. The research results not only improve the ability of computers to analyze and understand cross-media news, but also pave good ways for news retrieval, news person retrieval and personal news retrieval.
新闻人物标识在新闻理解分析和检索中起着重要作用,多人名-多人脸关联分析是大规模网络新闻人物标识的关键和难点问题。人名和人脸是人物鉴别的主要方面,分别承载着新闻的文本和图像信息,本项目拟利用图像与文本信息,进行跨模态的数据相似性计算和语义分析,开展多语种新闻热点人物标识方法研究。主要研究包括:1)针对多语种网络新闻的人名标识问题,研究利用新闻人名上下文建立高识别率的人名标识模型,根据多语种新闻人名特点构建高质量的人名翻译模型。2)针对大规模网络新闻人物的人脸表示问题,提出联合递归卷积神经网络与局部Gabor二值模式直方图序列的人脸特征表示方法,从而降低误识率和拒识率。3)针对多人名-多人脸关系建模问题,研究利用多语种新闻多源信息的互补性,构建跨模态的新闻人名主题网络,提升人物标识的准确率。研究成果可提高计算机对跨模态新闻的理解能力,且为网络新闻检索、新闻人物检索、人物新闻检索等研究奠定基础。
结项摘要
按项目申报书要求,本项目围绕跨模态的多语种新闻热点人物标识方法开展研究,主要研究网络新闻的人名标识与多语种新闻人名翻译方法、网络新闻人物的人脸表示方法、多语种网络新闻的多人名-多人脸关系建模方法。拟在国际高水平期刊与会议发表论文4-5篇,申请发明专利1-2项。培养硕士研究生2-3名。三年来,项目组成员围绕既定目标进行深入研究并取得较大科研进展。具体而言,在新闻人名标识方面,提出融合ALBert+Bi-LSTM+CRF的命名实体识别算法,有效提高新闻人名标识的准确率、召回率和F1值;在多语种新闻人名翻译方面,提出基于最优传输理论进行信息对齐的多语种神经机器翻译算法,有效提升翻译质量。在新闻人脸表示方面,提出融合迁移学习与注意力增强网络的佩戴口罩人脸识别算法,有效提高佩戴口罩人脸识别的准确率;在多人名-多人脸关系建模方面,提出基于知识图谱的新闻多人名-多人脸关系建模算法,有效提升跨模态数据融合效果。基于上述研究内容,项目共发表论文6篇,其中包含Applied Sciences、Multimedia tools and application、Discrete Dynamics in Nature and Society等SCI论文4篇,申请发明专利4项,授权发明专利1项,指导硕士研究生3人,已完成项目计划书制定的所有目标。项目负责人目前正积极与企业开展合作,有望将项目研究成果进行试点与推广。
项目成果
期刊论文数量(5)
专著数量(0)
科研奖励数量(0)
会议论文数量(1)
专利数量(5)
Face Shape Classification Based on Bilinear Network with Attention Mechanism
基于带有注意力机制的双线性网络的人脸形状分类
- DOI:10.1088/1742-6596/2278/1/012041
- 发表时间:2022-05
- 期刊:IOP Publishing
- 影响因子:--
- 作者:Jiawei Duan;Xueping Su;Jie Ren;Longsheng Xie
- 通讯作者:Longsheng Xie
Personalized Chinese Tourism Recommendation Algorithm Based on Knowledge Graph
基于知识图谱的个性化中国旅游推荐算法
- DOI:10.3390/app122010226
- 发表时间:2022-10
- 期刊:Applied Sciences
- 影响因子:--
- 作者:Xueping Su;Jiao He;Jie Ren;Jinye Peng
- 通讯作者:Jinye Peng
Automatic identification of focus personage in multi-lingual news images
多语言新闻图像中焦点人物自动识别
- DOI:10.1007/s11042-020-10254-4
- 发表时间:2021-01
- 期刊:Multimedia Tools and Applications
- 影响因子:3.6
- 作者:Xueping Su;Danyao Zhu;Jie Ren;Matthias Rätsch
- 通讯作者:Matthias Rätsch
Personalized Clothing Recommendation Based on User Emotional Analysis
基于用户情感分析的个性化服装推荐
- DOI:10.1155/2020/7954393
- 发表时间:2020-03
- 期刊:Discrete Dynamics in Nature and Society
- 影响因子:1.4
- 作者:Xueping Su;Meng Gao;Jie Ren;Yunhong Li;Matthias Rätsch
- 通讯作者:Matthias Rätsch
Face mask detection and classification via deep transfer learning.
通过深度迁移学习进行口罩检测和分类
- DOI:10.1007/s11042-021-11772-5
- 发表时间:2022
- 期刊:Multimedia tools and applications
- 影响因子:3.6
- 作者:Su X;Gao M;Ren J;Li Y;Dong M;Liu X
- 通讯作者:Liu X
数据更新时间:{{ journalArticles.updateTime }}
{{
item.title }}
{{ item.translation_title }}
- DOI:{{ item.doi || "--"}}
- 发表时间:{{ item.publish_year || "--" }}
- 期刊:{{ item.journal_name }}
- 影响因子:{{ item.factor || "--"}}
- 作者:{{ item.authors }}
- 通讯作者:{{ item.author }}
数据更新时间:{{ journalArticles.updateTime }}
{{ item.title }}
- 作者:{{ item.authors }}
数据更新时间:{{ monograph.updateTime }}
{{ item.title }}
- 作者:{{ item.authors }}
数据更新时间:{{ sciAawards.updateTime }}
{{ item.title }}
- 作者:{{ item.authors }}
数据更新时间:{{ conferencePapers.updateTime }}
{{ item.title }}
- 作者:{{ item.authors }}
数据更新时间:{{ patent.updateTime }}
其他文献
新闻图像中重要人物的自动标识
- DOI:--
- 发表时间:2013
- 期刊:计算机辅助设计与图形学学报
- 影响因子:--
- 作者:苏雪平;彭进业;冯晓毅;吴俊
- 通讯作者:吴俊
新闻图像中重要人物的自动标志
- DOI:--
- 发表时间:2013
- 期刊:计算机辅助设计与图形学学报
- 影响因子:--
- 作者:苏雪平;彭进业;冯晓毅;吴俊
- 通讯作者:吴俊
其他文献
{{
item.title }}
{{ item.translation_title }}
- DOI:{{ item.doi || "--" }}
- 发表时间:{{ item.publish_year || "--"}}
- 期刊:{{ item.journal_name }}
- 影响因子:{{ item.factor || "--" }}
- 作者:{{ item.authors }}
- 通讯作者:{{ item.author }}
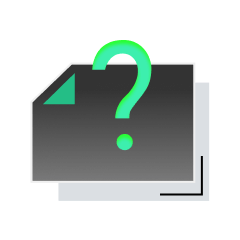
内容获取失败,请点击重试
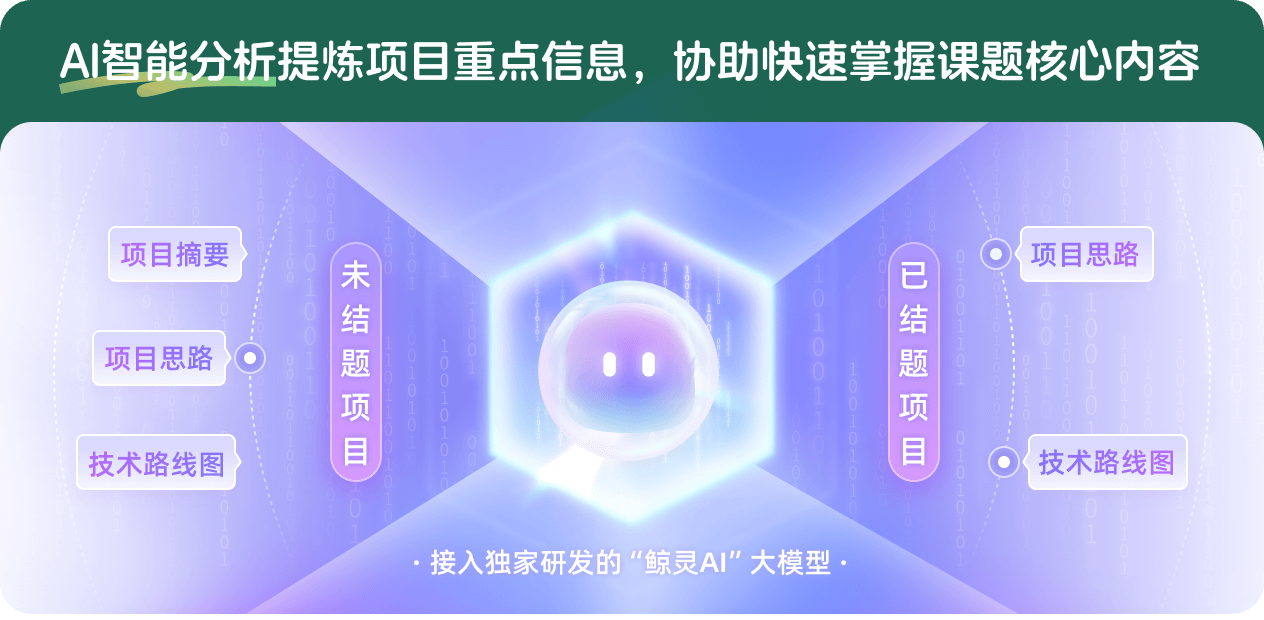
查看分析示例
此项目为已结题,我已根据课题信息分析并撰写以下内容,帮您拓宽课题思路:
AI项目摘要
AI项目思路
AI技术路线图
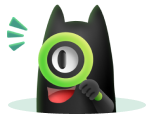
请为本次AI项目解读的内容对您的实用性打分
非常不实用
非常实用
1
2
3
4
5
6
7
8
9
10
您认为此功能如何分析更能满足您的需求,请填写您的反馈:
相似国自然基金
{{ item.name }}
- 批准号:{{ item.ratify_no }}
- 批准年份:{{ item.approval_year }}
- 资助金额:{{ item.support_num }}
- 项目类别:{{ item.project_type }}
相似海外基金
{{
item.name }}
{{ item.translate_name }}
- 批准号:{{ item.ratify_no }}
- 财政年份:{{ item.approval_year }}
- 资助金额:{{ item.support_num }}
- 项目类别:{{ item.project_type }}