通用可扩展神经形态芯片及其关键技术研究
项目介绍
AI项目解读
基本信息
- 批准号:61771097
- 项目类别:面上项目
- 资助金额:62.0万
- 负责人:
- 依托单位:
- 学科分类:F0124.生物电子学与生物信息处理
- 结题年份:2021
- 批准年份:2017
- 项目状态:已结题
- 起止时间:2018-01-01 至2021-12-31
- 项目参与者:于奇; 孙明远; 王俊杰; 伍元聪; 詹希童; 王弘喆; 刘广玉; 黄歆羡;
- 关键词:
项目摘要
Currently, brain-inspired neuromorphic chip has attracted much attention. However, most neuromorphic chips are application specific integrated circuits (ASICs), which are of poor versatility, and difficult to expand to large scale, and difficult to realize off- and on-line learning simultaneously. In our previous work, learning from the information process mechanism of the brain, we developed a general-purpose and scalable neuromorphic core in the scale of 256*256 based on the discrete digital integrated circuit technology. In this project, we will further develop the core to the scale of 1024*1024, improve the computing function of the neuron module, and improve the versatility and scalability of the core. And then, learning from the hierarchical and organizational structure of the brain, we will use the hardware multiplexing and network on chip (NOC) technologies to extend the chip scale, and then design and tape out multi-core general-purpose neuromorphic chip, which will lay the foundation for large-scale many-core neuromorphic chip. Finally, we will integrate the shallow / deep artificial neural network, spike neural network with the multi-core neuromorphic chip to realize the brain-like recognition and classification functions through off-line learning and on-line learning. This project has important scientific and practical value for large-scale general-purpose neuromorphic or brain-like computing, as well as large-scale brain functional integration and brain-like intelligence.
受大脑神经系统工作机制启发产生的新型神经形态芯片是国内外研究热点。当前神经形态芯片多为专用芯片,通用性差、难扩展,难与众多神经网络算法有效整合,难以同时实现离线和在线学习。前期,申请人借鉴大脑信息处理机制,基于数字CMOS集成电路技术研发了规模为256*256的高通用可扩展神经形态芯片内核。基于此,本课题将进一步扩展神经形态芯片内核规模至1024*1024,完善其神经元模块计算功能,优化其通用性和可扩展性;进而,借鉴大脑分层分块协同工作机制,基于硬件复用和片上网络技术扩展芯片内核数量,研发多核通用神经形态芯片,为大规模众核神经形态芯片研究打下基础;之后,探索神经形态芯片实际应用,将多核神经形态芯片与浅层/深度/脉冲神经网络整合,通过片外离线学习和片上在线学习,实现识别分类、联想记忆等人脑认知功能。本课题对大规模通用神经形态计算和类脑计算、大规模脑功能整合以及类脑智能有重要科学和应用价值。
结项摘要
针对脑启发神经形态芯片面临的扩展难、集成度低、通用性差、算法模型部署难等问题,本课题开展了高通用可扩展神经形态芯片技术研究:(1)建立了内核紧凑化设计技术方案,使得内核硬件开销与其集成的神经元数量弱相关,为大规模神经形态芯片研发提供了理论和技术支撑;(2)基于紧凑化技术研发了全连接神经形态内核,其结构紧凑、可重构、可扩展、且可部署多种模型;(3)基于紧凑性内核研发了三款神经形态芯片,其中103规模神经形态系统在MNIST数据集下获得96.2%的识别准确率、104规模神经形态芯片将神经元集成度提高约5倍、106规模神经形态芯片中单核集成当前最大规模的神经元且神经元集成密度提升两个以上数量级,为构建大规模神经形态芯片提供了实践方案;(4)面向自主神经形态架构,建立了直接训练和间接获取高精度量化/非量化脉冲神经网络模型(SNN)的方法,所构建的STF-SNN模型在 CIFAR10 和 DVS-CIFAR10 上实现95.77%准确率,分别比基于帧和基于事件的信息的非融合 SNN 高 5.01% 和 19.27%,为神经形态芯片的应用提供了算法支撑;(5)建立了SNN模型和算术运算模型高效部署方案,并围绕自主神经形态芯片研发了融合识别-运算-书写功能的智能系统,为研究神经形态芯片迭代关键问题提供了参考平台。本课题形成的关键理论和技术方案对构建大规模、高密度、低功耗、高通用神经形态系统的有重要参考价值,研发的神经形态芯片在边缘智能领域有广阔的应用前景。
项目成果
期刊论文数量(17)
专著数量(0)
科研奖励数量(0)
会议论文数量(1)
专利数量(24)
Application of Deep Compression Technique in Spiking Neural Network Chip
深度压缩技术在尖峰神经网络芯片中的应用
- DOI:10.1109/tbcas.2019.2952714
- 发表时间:2019-11
- 期刊:IEEE Transactions on Biomedical Circuits and Systems
- 影响因子:--
- 作者:Yanchen Liu;Kun Qian;Shaogang Hu;Kun An;Sheng Xu;Xitong Zhan;J. J. Wang;Rui Guo;Yuancong Wu;Tu-Pei Chen;Qi Yu;Yang Liu
- 通讯作者:Yang Liu
Direct training of hardware-friendly weight binarized spiking neural network with surrogate gradient learning towards spatio-temporal event-based dynamic data recognition
利用代理梯度学习直接训练硬件友好的权重二值化尖峰神经网络,实现基于时空事件的动态数据识别
- DOI:10.1016/j.neucom.2021.06.070
- 发表时间:2021
- 期刊:Neurocomputing
- 影响因子:6
- 作者:Qiao GC;Ning N;Zuo Y;Hu SG;Yu Q;Liu Y
- 通讯作者:Liu Y
Implementation of a Low Noise Amplifier With Self-Recovery Capability
具有自恢复能力的低噪声放大器的实现
- DOI:10.1109/access.2019.2907524
- 发表时间:2019
- 期刊:IEEE Access
- 影响因子:3.9
- 作者:Liu Yanchen;Zhang Caizhi;Chen Tupei;Kong Deyu;Guo Rui;Wang J J;Wu Yuancong;Hu S G;Rong L M;Yu Qi;Liu Yang
- 通讯作者:Liu Yang
Winner-takes-all mechanism realized by memristive neural network
忆阻神经网络实现赢者通吃机制
- DOI:10.1063/1.5120973
- 发表时间:2019-12-09
- 期刊:APPLIED PHYSICS LETTERS
- 影响因子:4
- 作者:Wang, J. J.;Yu, Q.;Liu, Y.
- 通讯作者:Liu, Y.
A versatile neuromorphic system based on simple neuron model
基于简单神经元模型的多功能神经形态系统
- DOI:10.1063/1.5052609
- 发表时间:2019-01
- 期刊:AIP Advances
- 影响因子:1.6
- 作者:C. M. Zhang;G. C. Qiao;S. G. Hu;J. J. Wang;Z. W. Liu;Y. A. Liu;Q. Yu;Y. Liu
- 通讯作者:Y. Liu
数据更新时间:{{ journalArticles.updateTime }}
{{
item.title }}
{{ item.translation_title }}
- DOI:{{ item.doi || "--"}}
- 发表时间:{{ item.publish_year || "--" }}
- 期刊:{{ item.journal_name }}
- 影响因子:{{ item.factor || "--"}}
- 作者:{{ item.authors }}
- 通讯作者:{{ item.author }}
数据更新时间:{{ journalArticles.updateTime }}
{{ item.title }}
- 作者:{{ item.authors }}
数据更新时间:{{ monograph.updateTime }}
{{ item.title }}
- 作者:{{ item.authors }}
数据更新时间:{{ sciAawards.updateTime }}
{{ item.title }}
- 作者:{{ item.authors }}
数据更新时间:{{ conferencePapers.updateTime }}
{{ item.title }}
- 作者:{{ item.authors }}
数据更新时间:{{ patent.updateTime }}
其他文献
其他文献
{{
item.title }}
{{ item.translation_title }}
- DOI:{{ item.doi || "--" }}
- 发表时间:{{ item.publish_year || "--"}}
- 期刊:{{ item.journal_name }}
- 影响因子:{{ item.factor || "--" }}
- 作者:{{ item.authors }}
- 通讯作者:{{ item.author }}
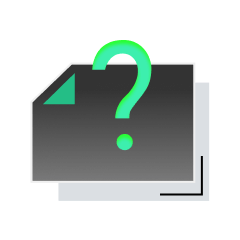
内容获取失败,请点击重试
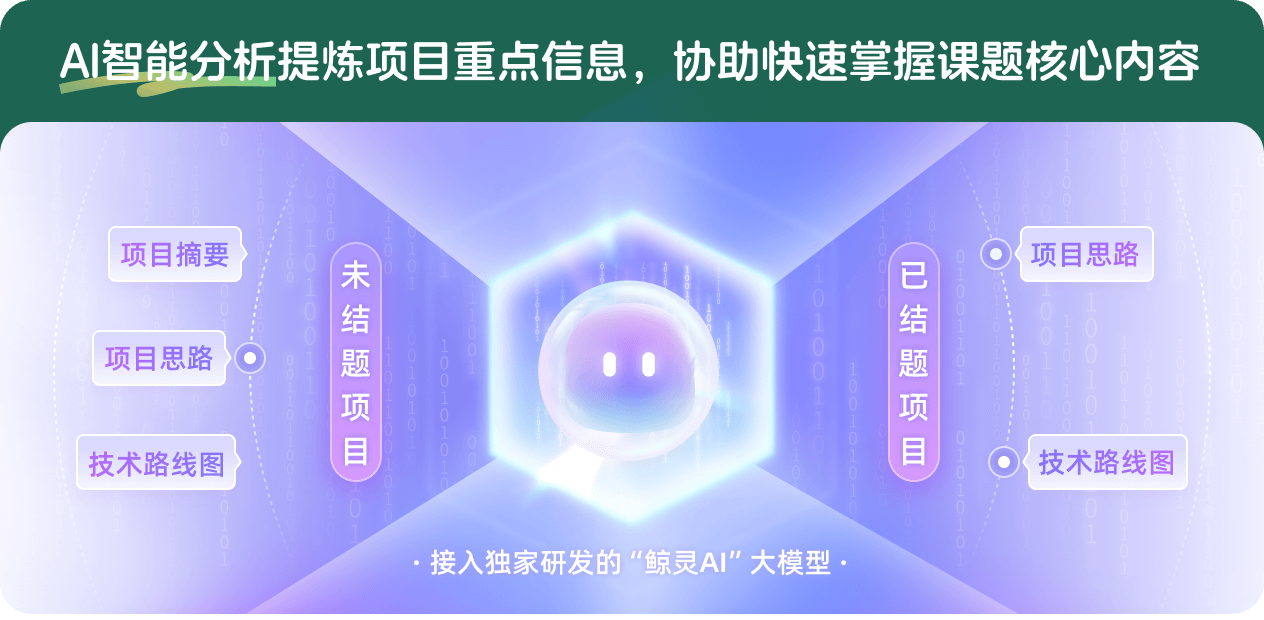
查看分析示例
此项目为已结题,我已根据课题信息分析并撰写以下内容,帮您拓宽课题思路:
AI项目摘要
AI项目思路
AI技术路线图
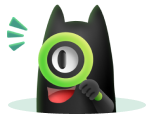
请为本次AI项目解读的内容对您的实用性打分
非常不实用
非常实用
1
2
3
4
5
6
7
8
9
10
您认为此功能如何分析更能满足您的需求,请填写您的反馈:
相似国自然基金
{{ item.name }}
- 批准号:{{ item.ratify_no }}
- 批准年份:{{ item.approval_year }}
- 资助金额:{{ item.support_num }}
- 项目类别:{{ item.project_type }}
相似海外基金
{{
item.name }}
{{ item.translate_name }}
- 批准号:{{ item.ratify_no }}
- 财政年份:{{ item.approval_year }}
- 资助金额:{{ item.support_num }}
- 项目类别:{{ item.project_type }}