不可靠无线传感器网络中自适应稀疏压缩采样关键技术研究
项目介绍
AI项目解读
基本信息
- 批准号:61402009
- 项目类别:青年科学基金项目
- 资助金额:24.0万
- 负责人:
- 依托单位:
- 学科分类:F0208.物联网及其他新型网络
- 结题年份:2017
- 批准年份:2014
- 项目状态:已结题
- 起止时间:2015-01-01 至2017-12-31
- 项目参与者:郑啸; 朱彤; 卫琳娜; 王倩; 高汉; 郑廷廷;
- 关键词:
项目摘要
Data gathering technology is one of the key techniques for the applications of large-scale wireless sensor networks (WSNs). To the best of our knowledge, most of existing theory and techniques have three challenges, poor robustness for data recovery, high sampling frequency and the lack of data prediction. The proposal aims at solving the measurement matrix not enough sparse, fixed sampling mode and lack of correlation of time and space utilization. Our research carries out the following three aspects. (1) Study on representation basis design based on the measurement matrix and the gathering data, using principal component analysis technique and the Gaussian probability model for extracting the structure of gathering data. It is used for solving that measurement value is not damaged by packet loss. (2) Study on adaptive compressive sampling for data gathering, which implements principal component selection using online learning way. It can make measurement matrix update adaptively and solve the problem of fixed sampling mode; (3) Study on spatial-temporal sampling and joint optimization of data gathering, which exploits the spatial-temporal joint optimization techniques and low rank completion theory to implement missing data completion and prediction under low sampling rate. It can solve the problem of separating spatial-temporal optimization. Through study on the project, we will implement robust compressive sampling data gathering in unreliable WSN. It also can expand the applications of CS theory in the distributed environment.
数据收集技术是大规模无线传感器网络(WSN)应用中的关键技术之一。针对压缩感知理论在该领域实际应用中面临的三个挑战:数据恢复鲁棒性差、采样频率过高和缺少网络数据预测功能。本课题拟解决测量矩阵不够稀疏、采样模式固定和缺少时空相关信息利用的三个关键问题。具体开展以下研究:(1)根据测量矩阵和收集数据进行稀疏表示基设计,利用主成份分析技术和高斯概率模型来对收集数据结构的提取,解决测量值易被网络丢包严重破坏的问题;(2)研究空间自适应稀疏采样数据收集,利用在线学习方式进行表示基的主分量选取,实现测量矩阵自适应更新,解决网络采样模式固定的问题;(3)研究时空联合的网络数据填充与预测算法,采用时空联合优化技术,结合低秩矩阵填充理论,实现低采样率下的有效优化问题求解,解决时空维度各自优化的问题。通过本项目研究,预期实现在大规模不可靠WSN下的有效稀疏压缩采样数据收集,同时扩展压缩感知在分布式环境中的应用。
结项摘要
为了提高不可靠网络环境下压缩数据收集质量,本项目针对其涉及到的关键技术展开一系列相关研究。在本项目执行过程中已发表学术论文16篇,其中SCI/EI检索期刊7篇,EI检索会议2篇,申报发明专利1项,软件著作权2项,培养研究生5名,已毕业3名。. 本项目在执行过程中较好的完成了预期研究目标,其主要研究内容与成果包括四个方面:(1)提出联合路由的稀疏投影无线传感器网络数据收集方案,降低网络丢包对测量值的破坏,实现不可靠网络环境下的鲁棒压缩感知数据收集;(2)提出限制条件下的稀疏表示基学习算法,实现压缩感知投影矩阵与网络路由的主动匹配,减少网络中传输的数据包数目,降低传感器节点资源消耗;(3)提出自适应状态感知的时空数据采样方案,实现低网络资源消耗下的事件源的有效监控;(4)提出基于压缩感知的时空大规模室外公共信号收集框架与方法,实现数据的稀疏采样与感知节点位置信息的有效保护。同时,本研究还扩展到感知数据的结构信息保护方面研究,实现感知数据在云端安全自适应存储计算外包。. 通过本项目的研究,在一定程度上解决不可靠无线传感器网络环境下,利用压缩感知进行数据收集中存在感知数据易受网络丢包干扰问题。本项目研究方案不仅可以减少网络能量消耗,同时还为网络数据在云端的存储提供一定的安全保证,为稀疏采样网络数据收集提供一定的理论依据与实际应用价值。
项目成果
期刊论文数量(12)
专著数量(0)
科研奖励数量(0)
会议论文数量(4)
专利数量(1)
基于路径上报的车联网轨迹隐私保护
- DOI:--
- 发表时间:2017
- 期刊:计算机研究与发展
- 影响因子:--
- 作者:吴宣够;王朋飞;郑啸;樊旭
- 通讯作者:樊旭
基于虚拟积分激励的内容部署方法
- DOI:10.15888/j.cnki.csa.006144
- 发表时间:2018
- 期刊:计算机应用系统
- 影响因子:--
- 作者:张云;郑啸;黄溯
- 通讯作者:黄溯
移动机会网络中接触时间感知的协作缓存策略
- DOI:--
- 发表时间:--
- 期刊:计算机研究与发展
- 影响因子:--
- 作者:郑啸;高汉;王修君;秦峰
- 通讯作者:秦峰
网络热点新闻焦点识别与演化跟踪
- DOI:--
- 发表时间:2017
- 期刊:小型微型计算机系统
- 影响因子:--
- 作者:刘玉文;吴宣够;郭强
- 通讯作者:郭强
基于高斯模型的随机采样网络数据重构的研究
- DOI:--
- 发表时间:2016
- 期刊:滁州学院学报
- 影响因子:--
- 作者:王倩;吴宣够
- 通讯作者:吴宣够
数据更新时间:{{ journalArticles.updateTime }}
{{
item.title }}
{{ item.translation_title }}
- DOI:{{ item.doi || "--"}}
- 发表时间:{{ item.publish_year || "--" }}
- 期刊:{{ item.journal_name }}
- 影响因子:{{ item.factor || "--"}}
- 作者:{{ item.authors }}
- 通讯作者:{{ item.author }}
数据更新时间:{{ journalArticles.updateTime }}
{{ item.title }}
- 作者:{{ item.authors }}
数据更新时间:{{ monograph.updateTime }}
{{ item.title }}
- 作者:{{ item.authors }}
数据更新时间:{{ sciAawards.updateTime }}
{{ item.title }}
- 作者:{{ item.authors }}
数据更新时间:{{ conferencePapers.updateTime }}
{{ item.title }}
- 作者:{{ item.authors }}
数据更新时间:{{ patent.updateTime }}
其他文献
基于TSSCM模型的新闻舆情演化识别
- DOI:--
- 发表时间:2017
- 期刊:情报杂志
- 影响因子:--
- 作者:刘玉文;郭强;吴宣够;张钰
- 通讯作者:张钰
链路不可靠下稀疏投影无线传感器网络数据收集研究
- DOI:--
- 发表时间:2019
- 期刊:计算机学报
- 影响因子:--
- 作者:吴宣够;储昭斌;郑啸;王修君;杨盘隆
- 通讯作者:杨盘隆
小波树结构在贝叶斯压缩感知图像重构中的应用研究
- DOI:--
- 发表时间:2014
- 期刊:计算机科学
- 影响因子:--
- 作者:袁琴;吴宣够;熊焰
- 通讯作者:熊焰
其他文献
{{
item.title }}
{{ item.translation_title }}
- DOI:{{ item.doi || "--" }}
- 发表时间:{{ item.publish_year || "--"}}
- 期刊:{{ item.journal_name }}
- 影响因子:{{ item.factor || "--" }}
- 作者:{{ item.authors }}
- 通讯作者:{{ item.author }}
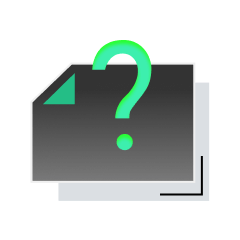
内容获取失败,请点击重试
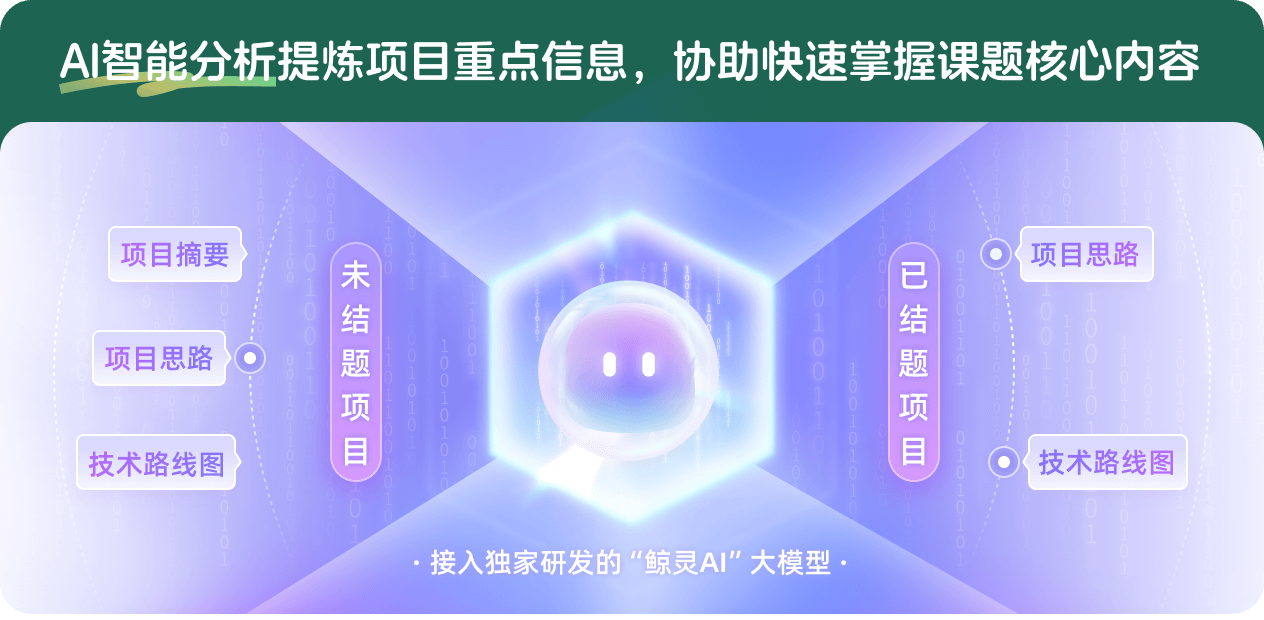
查看分析示例
此项目为已结题,我已根据课题信息分析并撰写以下内容,帮您拓宽课题思路:
AI项目摘要
AI项目思路
AI技术路线图
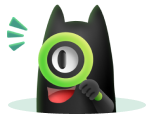
请为本次AI项目解读的内容对您的实用性打分
非常不实用
非常实用
1
2
3
4
5
6
7
8
9
10
您认为此功能如何分析更能满足您的需求,请填写您的反馈:
吴宣够的其他基金
面向联邦学习的异构智能物联网资源优化研究
- 批准号:62172003
- 批准年份:2021
- 资助金额:58 万元
- 项目类别:面上项目
面向路径隐私保护的移动群智感知数据收集研究
- 批准号:61672038
- 批准年份:2016
- 资助金额:62.0 万元
- 项目类别:面上项目
相似国自然基金
{{ item.name }}
- 批准号:{{ item.ratify_no }}
- 批准年份:{{ item.approval_year }}
- 资助金额:{{ item.support_num }}
- 项目类别:{{ item.project_type }}
相似海外基金
{{
item.name }}
{{ item.translate_name }}
- 批准号:{{ item.ratify_no }}
- 财政年份:{{ item.approval_year }}
- 资助金额:{{ item.support_num }}
- 项目类别:{{ item.project_type }}