人体行为未来状态视觉信息生成关键技术研究
项目介绍
AI项目解读
基本信息
- 批准号:61906195
- 项目类别:青年科学基金项目
- 资助金额:24.0万
- 负责人:
- 依托单位:
- 学科分类:F0604.机器感知与机器视觉
- 结题年份:2022
- 批准年份:2019
- 项目状态:已结题
- 起止时间:2020-01-01 至2022-12-31
- 项目参与者:--
- 关键词:
项目摘要
The short-term prediction and visual imagination is a basic ability of human brains. It is a very important research topic in the brain-inspired artificial intelligence, and also a cutting-edge research task in the computer vision research filed. However, current studies are still in exploring basic methods, and there are many key problems need to be handled. This research is based on the cognitive process of human brains, and proposes a novel research program. 1) On the aspect of the activity feature extraction, this research proposes a hybrid neural network model based on image convolution and graph convolution, achieving synchronous feature extractions of image appearances and pose transformations. 2) On the aspect of uncertainty future state prediction, this research proposes a conditional variational autoencoder and pose map feature reasoning network, achieving uncertainty multi-modal prediction. 3) On the aspect of multi-target constraint, this research propose progressive tight coupling conditional image generation method, and achieves semantic consistency and visual fidelity in the image generation. This research framework unify the cognitive process of “observation-prediction-verification-optimization” into an integrated model, and achieves self-supervised model optimization. This research is expected to contribute to the key research project “The Basic Theory and Technology of Brain-inspired Intelligent Agent Autonomous Reasoning and Unknown Imagination”, and provides new ideas and approaches for this research field.
对人体行为未来状态的短时预测和视觉想象是人类大脑的基本能力,在类脑人工智能领域具有重要的研究意义,也是近年来计算机视觉领域的前沿研究方向。然而,目前的研究尚停留在探索阶段,关键问题亟待解决。本申请从模仿大脑认知过程的独特角度出发,提出了新颖的研究方案。1)针对行为特征提取难题,提出基于图像卷积和图卷积的异构神经网络模型,实现图像内容和行为趋势的同步特征提取;2)针对不确定性未来状态预测问题,提出变分自编码和位姿图特征解码推理网络,实现对不确定状态的多峰预测;3)针对生成信息的多目标约束问题,提出渐进式强耦合条件约束图像生成方法,实现语义连贯性和视觉真实性约束的多目标生成。该研究框架将认知过程的“观察-预测-验证-优化”统一为一个完整模型,实现了自监督模型优化。本项目预期为解决关键科学问题:“类脑智能体自主推理与未知想象基础理论与关键技术”做出贡献,为该领域的发展提供新思路和新方法。
结项摘要
该项目从类脑认知形成规律出发,对人体行为的未来状态预测这一科学问题进行研究。研究内容主要从四个方面展开:(1)感知:我们研究了异构特征的高精度姿态估计;(2)预测:我们研究了多峰预测算法和不确定性行为预测方法;(3)验证:我们研究了不确定性推理方法;(4)优化:我们研究了时序约束优化和模型的全局优化。此外,我们对技术落地中存在的问题进行了探索,对结构搜索和模型加速等问题进行了针对性研究。通过如上的研究工作,我们在多个层面上取得了重要成果。在感知层面,我们提出了便节点注意力图卷积网络(EVA-GCN),首次在人体头部姿态估计任务中引入图神经网络,使得姿态估计的精度达到了目前报告的最高水平。在预测方面,我们提出了基于生理学先验知识引导和时空约束的图变分自编码器模型(CST-VGAE),首次在人体头部姿态估计任务中提出了图谱模态上的长程多峰分布预测方法。在推理方面,我们提出了基于贝叶斯学习的人体行为不确定性量化方法和非对称性BSTG transformer模型。在目前已报告的学术研究中,首次使得长程人体行为未来状态的精确预测达到了六秒钟时长的密集预测。在优化方面,我们提出了基于时序验证约束的自监督优化方法。以上研究成果发表在CCF-A类会议和期刊论文中,获得研讨会最佳论文奖,技术应用成果获得第一届全国博士后创新创业大赛金奖。 通过本项目的研究,我们实现了模拟类脑认知的“观察-预测-验证-优化”模式和过程,这对于探索类脑智能与人机交互共融具有一定的科学意义,取得了丰富而有意义的研究成果,也为后续更深层次、更宽领域的研究打下了扎实的基础。
项目成果
期刊论文数量(2)
专著数量(0)
科研奖励数量(0)
会议论文数量(4)
专利数量(2)
Bayesian Automatic Model Compression
贝叶斯自动模型压缩
- DOI:10.1109/jstsp.2020.2977090
- 发表时间:2020-02
- 期刊:IEEE Journal of Selected Topics in Signal Processing
- 影响因子:7.5
- 作者:Jiaxing Wang;Haoli Bai;Jiaxiang Wu;Jian Cheng
- 通讯作者:Jian Cheng
Extremely Lightweight Skeleton-Based Action Recognition With ShiftGCN plus
使用 ShiftGCN 进行超轻量级基于骨架的动作识别
- DOI:10.1109/tip.2021.3104182
- 发表时间:2021-01-01
- 期刊:IEEE TRANSACTIONS ON IMAGE PROCESSING
- 影响因子:10.6
- 作者:Cheng, Ke;Zhang, Yifan;Lu, Hanqing
- 通讯作者:Lu, Hanqing
数据更新时间:{{ journalArticles.updateTime }}
{{
item.title }}
{{ item.translation_title }}
- DOI:{{ item.doi || "--"}}
- 发表时间:{{ item.publish_year || "--" }}
- 期刊:{{ item.journal_name }}
- 影响因子:{{ item.factor || "--"}}
- 作者:{{ item.authors }}
- 通讯作者:{{ item.author }}
数据更新时间:{{ journalArticles.updateTime }}
{{ item.title }}
- 作者:{{ item.authors }}
数据更新时间:{{ monograph.updateTime }}
{{ item.title }}
- 作者:{{ item.authors }}
数据更新时间:{{ sciAawards.updateTime }}
{{ item.title }}
- 作者:{{ item.authors }}
数据更新时间:{{ conferencePapers.updateTime }}
{{ item.title }}
- 作者:{{ item.authors }}
数据更新时间:{{ patent.updateTime }}
其他文献
其他文献
{{
item.title }}
{{ item.translation_title }}
- DOI:{{ item.doi || "--" }}
- 发表时间:{{ item.publish_year || "--"}}
- 期刊:{{ item.journal_name }}
- 影响因子:{{ item.factor || "--" }}
- 作者:{{ item.authors }}
- 通讯作者:{{ item.author }}
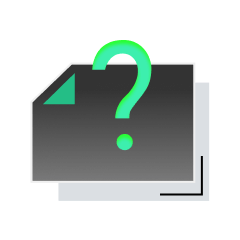
内容获取失败,请点击重试
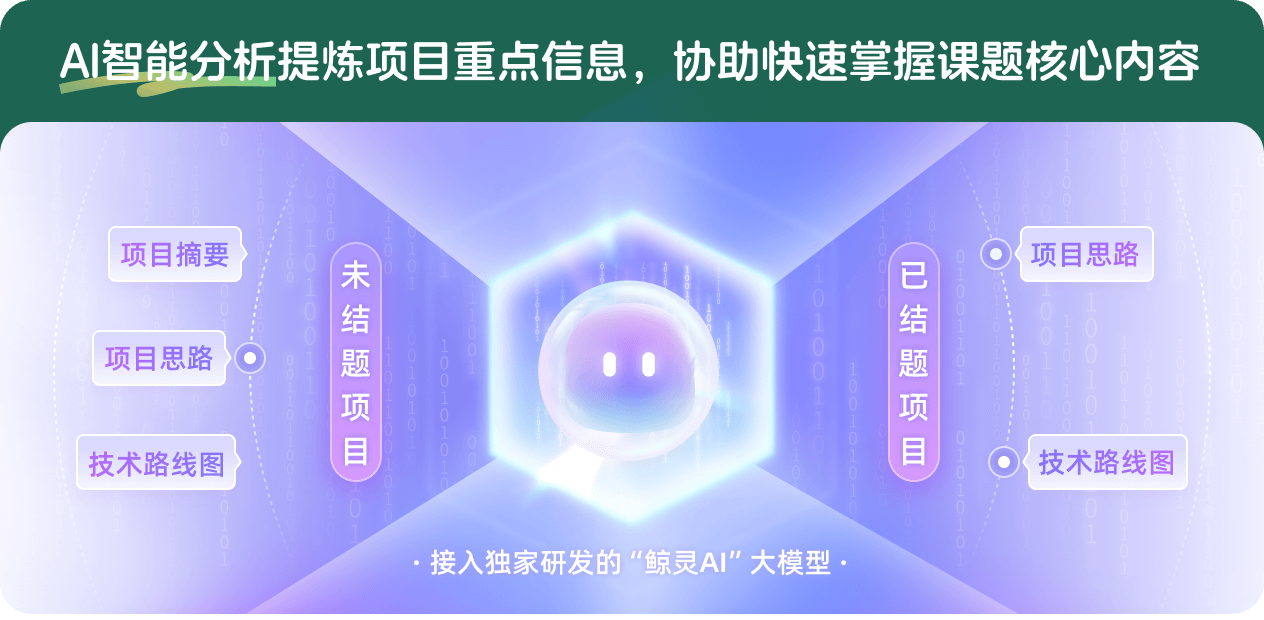
查看分析示例
此项目为已结题,我已根据课题信息分析并撰写以下内容,帮您拓宽课题思路:
AI项目摘要
AI项目思路
AI技术路线图
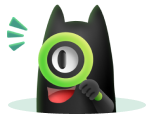
请为本次AI项目解读的内容对您的实用性打分
非常不实用
非常实用
1
2
3
4
5
6
7
8
9
10
您认为此功能如何分析更能满足您的需求,请填写您的反馈:
相似国自然基金
{{ item.name }}
- 批准号:{{ item.ratify_no }}
- 批准年份:{{ item.approval_year }}
- 资助金额:{{ item.support_num }}
- 项目类别:{{ item.project_type }}
相似海外基金
{{
item.name }}
{{ item.translate_name }}
- 批准号:{{ item.ratify_no }}
- 财政年份:{{ item.approval_year }}
- 资助金额:{{ item.support_num }}
- 项目类别:{{ item.project_type }}