基于任务双能力匹配的高复杂度场景驾驶分心险态辨识机理及模型构建
项目介绍
AI项目解读
基本信息
- 批准号:51905224
- 项目类别:青年科学基金项目
- 资助金额:24.0万
- 负责人:
- 依托单位:
- 学科分类:E0507.机械仿生学与生物制造
- 结题年份:2022
- 批准年份:2019
- 项目状态:已结题
- 起止时间:2020-01-01 至2022-12-31
- 项目参与者:--
- 关键词:
项目摘要
The existing theory of driving distraction does not consider the complexity of the change of driving distraction environment, it is difficult to apply the driving distraction discrimination of high complexity scene, and it is very important to study the identification mechanism of driving distraction risk in high complexity scene from the angle of improving the accuracy and efficiency of driving distraction discriminating model. Therefore, based on virtual driving simulation test and simulation data, this research analyzes the characteristic root and eigenvectors of driving distraction characterization factor, studies the correlation between the influence strength and contribution rate of index factor, explores the matching relationship between focus ability and demand ability, constructs the task double ability matching model, and extracts the confidence interval of driving distraction in high complexity scene. The dynamic threshold model is established to define the dynamic variation range of driving distraction threshold. To explore the mechanism of driving distraction risk identification under high complexity scene, and analyze the correlation between support vector machine and iterative algorithm, a high complexity scene driving distraction risk identification model based on support vector machine and iterative algorithm is constructed. The research will change the existing single , static index selection and threshold determination method based on low complexity. It is of positive scientific significance and value to further improve the theory of driving distraction and to further explore the mechanism of dangerous driving distraction in high-complexity scenarios.
现有驾驶分心理论未充分考虑驾驶分心环境变化的复杂性,难以适用高复杂度场景的驾驶分心判别,从提升驾驶分心判别模型精确性和高效性的角度,研究高复杂度场景下驾驶分心险态辨识机理。为此,基于驾驶仿真实验及数据,分析驾驶分心表征因子特征根及特征向量,研究指标因子的影响强度和贡献率大小的关联性,探究专注能力和需求能力的匹配关系,构建任务双能力匹配模型;提取高复杂度场景驾驶分心判别置信区间,建立动态阈值模型,界定驾驶分心阈值动态变化范围;探索高复杂度场景下驾驶分心险态辨识机理,分析支持向量机与迭代算法的关联性,构建基于支持向量机和迭代算法的高复杂度场景驾驶分心险态辨别模型。研究将改变现有的从低复杂度场景出发的单一、静止的指标选取和阈值确定方法,对进一步完善驾驶分心理论和深入挖掘高复杂度场景的驾驶分心险态辨识机理具有积极的科学意义和价值。
结项摘要
由于驾驶人分心而产生的注意偏离是引发道路交通事故最常见的原因。因此,针对驾驶分心表现及驾驶分心判别模型展开研究刻不容缓。本研究根据驾驶分心类别的划分,结合驾驶分心的定义设置分心次任务,基于Prescan与simulink耦合仿真程序构建了高复杂度驾驶分心场景,并对数据进行标准化处理,获取了三类场景(直行路段、停止交叉口、转向路段)下不同难度的视觉分心和认知分心数据。以高复杂度道路为实验场景,设置了4组变量(性别分组-组间变量、年龄分组-组间变量、视觉/认知任务-组内变量、难度分组-组内变量)进行驾驶模拟,分析了驾驶人(性别、年龄)在不同路段(直行、停止、转向)执行不同难度的视觉和认知次任务对驾驶分心特性表现的影响,总结了各分心特征的指标显著性。对驾驶专注能力及驾驶需求能力进行了抽象函数定义,选取了表征驾驶双能力的特征因子,对驾驶双能力匹配模态及险态定义进行了驾驶分心险态辨识机理分析。基于主成分分析提取表征驾驶分心的关键特征,包括注视时间比、注视点坐标、车速、加速度、方向盘转角、制动力,油门踏板开度7个特征指标,集成两种机器学习算法的集成构建了基于SVM-Adaboost的驾驶分心判别模型,并使用驾驶分心判别模型结合驾驶分心危险状态判别方法,对驾驶分心危险状态预警进行评估。研究结果表明,基于改进SVM-Adaboost驾驶分心判别模型精确度显著提高,对模拟实验中的六类分心状况判别效果显著,分心预警的使用能够显著提高交通安全水平,减少分心驾驶行为对道路交通安全的影响。
项目成果
期刊论文数量(7)
专著数量(0)
科研奖励数量(0)
会议论文数量(0)
专利数量(2)
Research on Calculation of Warning Zone Length of Freeway Based on Micro-Simulation Model
基于微观仿真模型的高速公路警戒区长度计算研究
- DOI:10.1109/access.2020.2989471
- 发表时间:2020
- 期刊:IEEE Access
- 影响因子:3.9
- 作者:Ge Huimin;Yang Yousen
- 通讯作者:Yang Yousen
驾驶分心综述
- DOI:--
- 发表时间:2021
- 期刊:交通运输工程学报
- 影响因子:--
- 作者:葛慧敏;郑明强;吕能超;陆颖;孙辉
- 通讯作者:孙辉
Study on Traffic Conflict Prediction Model of Closed Lanes on the Outside of Expressway
高速公路外侧封闭车道交通冲突预测模型研究
- DOI:10.3390/sym12060926
- 发表时间:2020-06
- 期刊:Symmetry
- 影响因子:--
- 作者:Ge Huimin;Huang Mingyue;Lu Ying;Yang Yousen
- 通讯作者:Yang Yousen
Adaptive Kernel Density Estimation for Traffic Accidents Based on Improved Bandwidth Research on Black Spot Identification Model
基于改进带宽的交通事故自适应核密度估计黑点识别模型研究
- DOI:10.3390/electronics11213604
- 发表时间:2022-11
- 期刊:Electronics
- 影响因子:2.9
- 作者:Ge Huimin;Lei Dong;Mingyue Huang;Wenkai Zang;Lijun Zhou
- 通讯作者:Lijun Zhou
Research on Characteristics and Trends of Traffic Flow Based on Mixed Velocity Method and Background Difference Method
基于混合速度法和背景差法的交通流特征和趋势研究
- DOI:10.1155/2020/8546479
- 发表时间:2020-08
- 期刊:Mathematical Problems in Engineering
- 影响因子:--
- 作者:Ge Huimin;Sun Hui;Lu Ying
- 通讯作者:Lu Ying
数据更新时间:{{ journalArticles.updateTime }}
{{
item.title }}
{{ item.translation_title }}
- DOI:{{ item.doi || "--"}}
- 发表时间:{{ item.publish_year || "--" }}
- 期刊:{{ item.journal_name }}
- 影响因子:{{ item.factor || "--"}}
- 作者:{{ item.authors }}
- 通讯作者:{{ item.author }}
数据更新时间:{{ journalArticles.updateTime }}
{{ item.title }}
- 作者:{{ item.authors }}
数据更新时间:{{ monograph.updateTime }}
{{ item.title }}
- 作者:{{ item.authors }}
数据更新时间:{{ sciAawards.updateTime }}
{{ item.title }}
- 作者:{{ item.authors }}
数据更新时间:{{ conferencePapers.updateTime }}
{{ item.title }}
- 作者:{{ item.authors }}
数据更新时间:{{ patent.updateTime }}
其他文献
新能源汽车产业专利池的形成机制
- DOI:10.15918/j.jbitss1009-3370.2017.0192
- 发表时间:2017
- 期刊:北京理工大学学报(社会科学版)
- 影响因子:--
- 作者:孙华平;胡凌翔;葛慧敏;刘桂锋
- 通讯作者:刘桂锋
其他文献
{{
item.title }}
{{ item.translation_title }}
- DOI:{{ item.doi || "--" }}
- 发表时间:{{ item.publish_year || "--"}}
- 期刊:{{ item.journal_name }}
- 影响因子:{{ item.factor || "--" }}
- 作者:{{ item.authors }}
- 通讯作者:{{ item.author }}
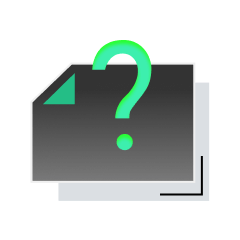
内容获取失败,请点击重试
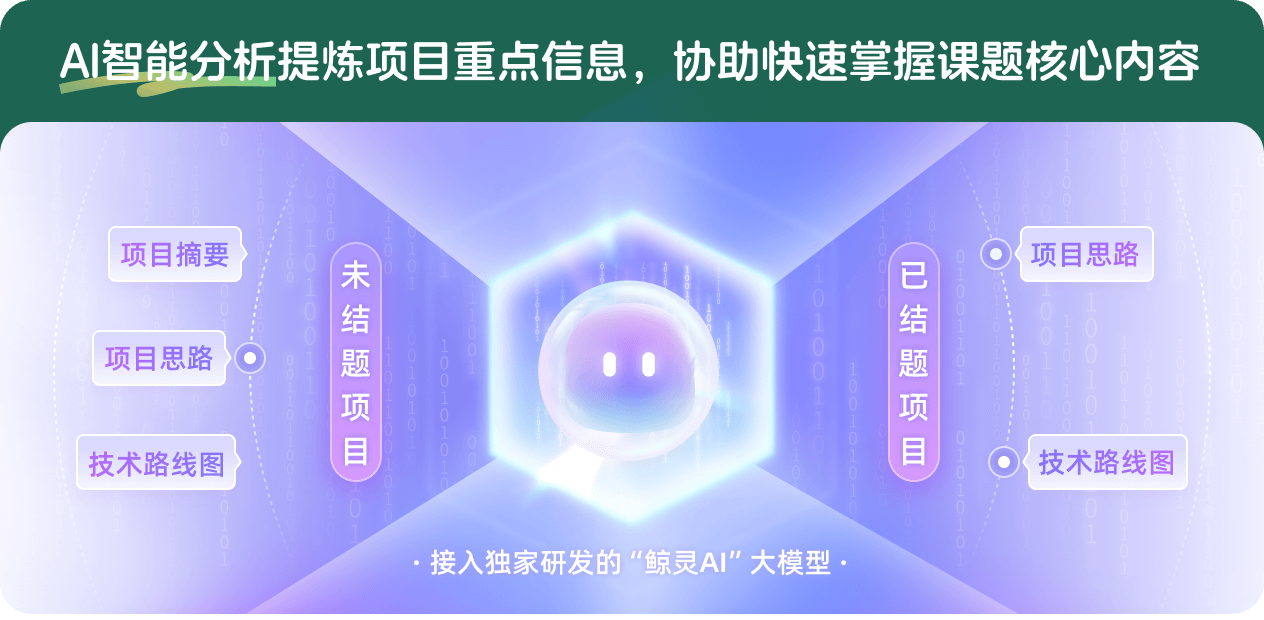
查看分析示例
此项目为已结题,我已根据课题信息分析并撰写以下内容,帮您拓宽课题思路:
AI项目摘要
AI项目思路
AI技术路线图
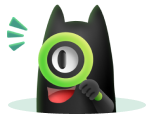
请为本次AI项目解读的内容对您的实用性打分
非常不实用
非常实用
1
2
3
4
5
6
7
8
9
10
您认为此功能如何分析更能满足您的需求,请填写您的反馈:
相似国自然基金
{{ item.name }}
- 批准号:{{ item.ratify_no }}
- 批准年份:{{ item.approval_year }}
- 资助金额:{{ item.support_num }}
- 项目类别:{{ item.project_type }}
相似海外基金
{{
item.name }}
{{ item.translate_name }}
- 批准号:{{ item.ratify_no }}
- 财政年份:{{ item.approval_year }}
- 资助金额:{{ item.support_num }}
- 项目类别:{{ item.project_type }}