数据驱动的高速高精度定位操作的广义点对点迭代学习控制及应用
项目介绍
AI项目解读
基本信息
- 批准号:61374102
- 项目类别:面上项目
- 资助金额:79.0万
- 负责人:
- 依托单位:
- 学科分类:F0301.控制理论与技术
- 结题年份:2017
- 批准年份:2013
- 项目状态:已结题
- 起止时间:2014-01-01 至2017-12-31
- 项目参与者:Chiang-Ju Chien; 刘喜梅; 赵彤; 秦浩华; Chia-Liang Tai; 张德霞; 苏涛; 韩吉志;
- 关键词:
项目摘要
It is one of the most important scientific and technological problems in advanced electronic industries to achieve high-precision positioning control for the high-speed motion systems in the chip packaging processes. However, it is most difficult to achieve an exact mathematical model for the high-speed motion system. It is generally known that iterative learning control (ILC) is a data-driven control approach in nature and can learn from repetitions to achieve perfect tracking. This project will present a series of data-driven generalized point-to-point iterative learning control for high-precision positioning operation of the high-speed motion systems by utilizing the repeatability of the chip packaging process. First, the data-driven terminal iterative learning control (TILC) will be proposed to attain the optimal acceleration switching point. And then two data-driven generalized point-to-point ILC will be provided for the high-precision positioning operation, where the standard ILC is to achieve the perfect tracking of the desired motion trajectory which passes the optimal acceleration switching point and the desired positioning point, and the multiple point-to-point ILC (MPTP-ILC) is to track both the optimal acceleration switching point and the desired positioning point directly, instead of the target trajectory. The energy-efficient property and control performance of the above proposed ILC and MPTP-ILC will be compared and accessed in order to provide a higher performance and lower energy-consumption control scheme finally in the positioning operation of the high-speed motion system. The robust design and analysis data-driven generalized point-to-point ILC will be extensively explored for more general time-iteration-varying systems under non-strictly repeatable conditions to achieve better tracking performance in the presence of the uncertainties of system structure and parameters, initial errors, iteration-varying tracking tasks and random disturbances. And the anti-windup generalized point-to-point ILC methods with input saturation will be developed to deal with actuator and transducer constraints. Finally, the efficiency and applicability of all the schemes to be proposed will be tested on the linear motor driven X-Y positioning table.
芯片封装平台高速高精度定位是先进电子制造业的重要科学技术问题,而建立高速运动系统精确模型非常困难。迭代学习控制具有重复中学习的能力,属于数据驱动控制方法,可实现高精度跟踪性能。本项目拟利用封装过程的重复性,在数据驱动的框架下,首先提出TILC方法确定高速运动的最优加速度切换点,然后提出高精度定位操作的两种学习控制方法,其中标准ILC目标于跟踪通过最优加速度切换点和精确定位点的期望运动轨线,而MPTP-ILC则直接跟踪最优加速度切换点和精确定位点,而非期望轨线,并对这两种方法的能耗情况和控制性能进行评价,给出高性能低能耗控制策略;讨论初始误差、跟踪目标变化、系统结构参数不确定性、随机扰动等因素的鲁棒性设计及执行器传感器饱和等问题,最终提出一套数据驱动的高速运动系统精确定位操作的广义点对点ILC方法及其收敛性和鲁棒性分析。基于直线电机X-Y平台设计半实物仿真实验,验证所提出方法的有效性。
结项摘要
高速运动系统精确模型的建立非常困难。迭代学习控制(ILC)具有重复中学习的能力,属于数据驱动控制方法。本项目主要目标是提出一套数据驱动的高速运动系统精确定位操作的广义点对点ILC 方法及其收敛性和鲁棒性分析。本项目按照计划已全面完成,取得了一些进展。(1)提出了数据驱动最优迭代学习控制方法设计和分析的统一框架,讨论了跟踪完整参考轨迹、跟踪参考轨迹上某些数据点和仅跟踪参考轨迹最终点的三类控制任务,分别给出了跟踪整个轨迹的数据驱动最优ILC、跟踪关键点的数据驱动最优点到点ILC及跟踪运行终点期望值的数据驱动最优TILC方法。(2)讨论了数据驱动广义最优ILC方法设计和实用中遇到的随机初始条件、随机扰动、迭代变化参考轨迹等不确定性问题,分别给出了基于初值补偿的,基于神经网络学习的,基于高阶内模的等一系列的数据驱动自适应ILC和自适应TILC方法。(3)研究了被控系统的受限问题,包括状态受限、输入受限、输出受限、输入输出变化率受限以及延时等问题,分别提出了受限的自适应ILC和数据驱动最优ILC等方法。(4)探讨了利用更多控制知识提高控制性能的可能性,提出了系列的高阶控制律、基于分块区间滚动优化的点到点ILC、基于在线输入信号的增强型终端ILC,基于动态预测模型的数据驱动最优TILC以及基于遗忘因子的TILC等方法。(5)对数据驱动的周期自适应控制方法、有效降低系统存储和计算量的采样ILC方法、以及状态扰动的迭代学习估计和无模型自适应切换控制等问题进行了研究。(6)应用方面,具体讨论了以直线电机为例的高速运行过程、复杂城市交通系统、列车运行控制、工业间歇过程、网络传输过程以及电机伺服系统等实际过程和问题的数据驱动控制和学习控制策略,提出了具有严谨的数学分析保障的、不依赖系统精确数学模型的、可实际应用的数据驱动与学习控制理论和方法,为现代工业自动化提供了理论支持和方法保障。
项目成果
期刊论文数量(28)
专著数量(0)
科研奖励数量(8)
会议论文数量(25)
专利数量(3)
A data-driven adaptive ILC for a class of nonlinear discrete-time systems with random initial states and iteration-varying target trajectory
一种用于具有随机初始状态和迭代变化目标轨迹的一类非线性离散时间系统的数据驱动自适应 ILC
- DOI:10.1016/j.jfranklin.2015.03.014
- 发表时间:2015
- 期刊:Journal of the Franklin Institute-Engineering and Applied Mathematics
- 影响因子:4.1
- 作者:Chi Ronghu;Hou Zhongsheng;Jin Shangtai
- 通讯作者:Jin Shangtai
Data-driven terminal iterative learning control with high-order learning law for a class of non-linear discrete-time multiple-input-multiple output systems
一类非线性离散时间多输入多输出系统的具有高阶学习律的数据驱动终端迭代学习控制
- DOI:10.1049/iet-cta.2014.0754
- 发表时间:2015
- 期刊:Iet Control Theory and Applications
- 影响因子:--
- 作者:Chi Ronghu;Liu Yu;Hou Zhongsheng;Jin Shangtai
- 通讯作者:Jin Shangtai
Adaptive iterative learning control for nonlinearly parameterised systems with unknown time-varying delays and input saturations
具有未知时变延迟和输入饱和度的非线性参数化系统的自适应迭代学习控制
- DOI:10.1080/00207179.2014.994103
- 发表时间:2015
- 期刊:International Journal of Control
- 影响因子:2.1
- 作者:Zhang Ruikun;Hou Zhongsheng;Chi Ronghu;Ji Honghai
- 通讯作者:Ji Honghai
High-order data-driven optimal TILC approach for fed-batch processes
用于流加过程的高阶数据驱动的最佳 TILC 方法
- DOI:10.1002/cjce.22232
- 发表时间:2015
- 期刊:Canadian Journal of Chemical Engineering
- 影响因子:2.1
- 作者:Chi Ronghu;Liu Yu;Hou Zhongsheng;Jin Shangtai
- 通讯作者:Jin Shangtai
On the current error based sampled-data iterative learning control with reduced memory capacity
基于电流误差的减少存储容量的采样数据迭代学习控制
- DOI:10.1007/s11633-015-0890-1
- 发表时间:2015
- 期刊:Int. Journal of Automation and Computing
- 影响因子:--
- 作者:Chiang-Ju Chien;Yu-Chung Hung;Ronghu Chi
- 通讯作者:Ronghu Chi
数据更新时间:{{ journalArticles.updateTime }}
{{
item.title }}
{{ item.translation_title }}
- DOI:{{ item.doi || "--"}}
- 发表时间:{{ item.publish_year || "--" }}
- 期刊:{{ item.journal_name }}
- 影响因子:{{ item.factor || "--"}}
- 作者:{{ item.authors }}
- 通讯作者:{{ item.author }}
数据更新时间:{{ journalArticles.updateTime }}
{{ item.title }}
- 作者:{{ item.authors }}
数据更新时间:{{ monograph.updateTime }}
{{ item.title }}
- 作者:{{ item.authors }}
数据更新时间:{{ sciAawards.updateTime }}
{{ item.title }}
- 作者:{{ item.authors }}
数据更新时间:{{ conferencePapers.updateTime }}
{{ item.title }}
- 作者:{{ item.authors }}
数据更新时间:{{ patent.updateTime }}
其他文献
基于学习自适应估计环的迭代学习
- DOI:--
- 发表时间:--
- 期刊:仪器仪表学报,Vol.26(8), pp800-803, 2005.
- 影响因子:--
- 作者:侯忠生*;池荣虎
- 通讯作者:池荣虎
船舶吊舱SSP推进电机的无模型自适应矢量控制方法
- DOI:--
- 发表时间:2014
- 期刊:交通运输工程学报
- 影响因子:--
- 作者:姚文龙;张均东;池荣虎;张桂臣
- 通讯作者:张桂臣
非线性离散时间系统的最优终端迭代学习控制(英文)
- DOI:--
- 发表时间:--
- 期刊:Kongzhi Lilun Yu Yinyong/Control Theory and Applications
- 影响因子:--
- 作者:池荣虎;侯忠生;王郸维;金尚泰
- 通讯作者:金尚泰
间歇过程最优迭代学习控制的发展:从基于模型到数据驱动
- DOI:--
- 发表时间:2017
- 期刊:自动化学报
- 影响因子:--
- 作者:池荣虎;侯忠生;黄彪
- 通讯作者:黄彪
基于数据驱动高阶学习律的轮式移动机器人轨迹跟踪控制
- DOI:10.1128/mbio.02590-19
- 发表时间:2021
- 期刊:南京信息工程大学学报(自然科学版)
- 影响因子:--
- 作者:李佳伟;林娜;池荣虎
- 通讯作者:池荣虎
其他文献
{{
item.title }}
{{ item.translation_title }}
- DOI:{{ item.doi || "--" }}
- 发表时间:{{ item.publish_year || "--"}}
- 期刊:{{ item.journal_name }}
- 影响因子:{{ item.factor || "--" }}
- 作者:{{ item.authors }}
- 通讯作者:{{ item.author }}
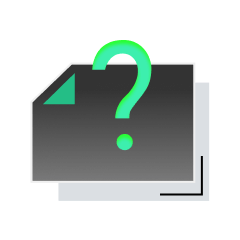
内容获取失败,请点击重试
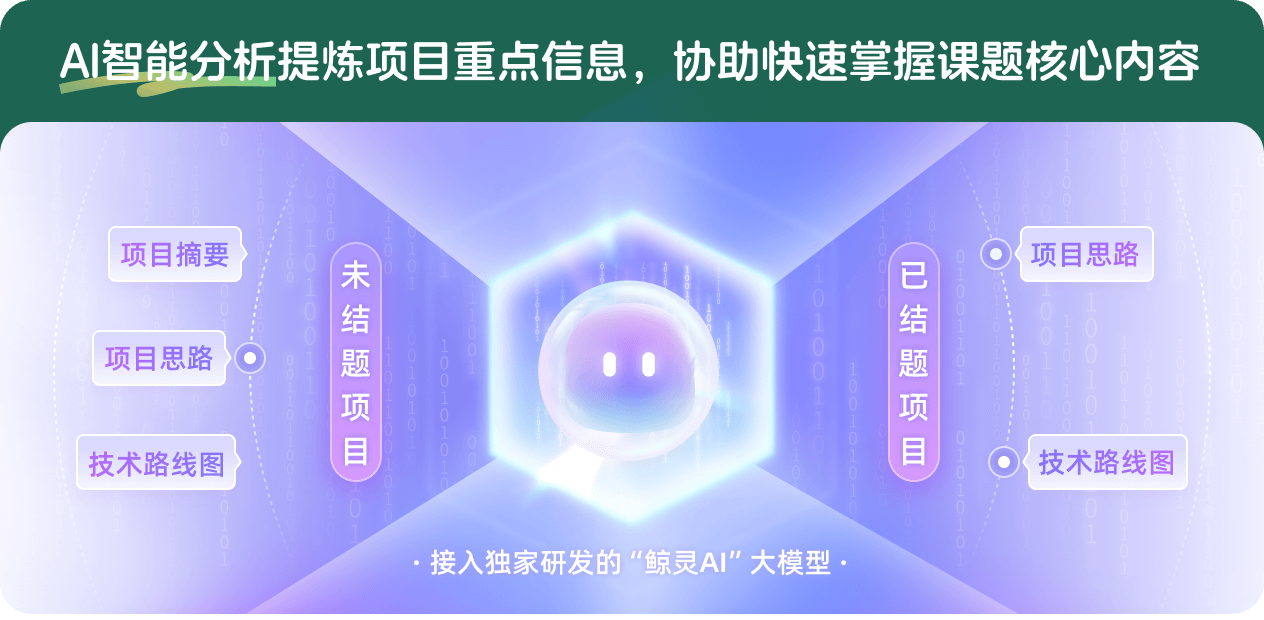
查看分析示例
此项目为已结题,我已根据课题信息分析并撰写以下内容,帮您拓宽课题思路:
AI项目摘要
AI项目思路
AI技术路线图
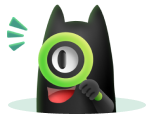
请为本次AI项目解读的内容对您的实用性打分
非常不实用
非常实用
1
2
3
4
5
6
7
8
9
10
您认为此功能如何分析更能满足您的需求,请填写您的反馈:
池荣虎的其他基金
基于数据驱动迭代学习的橡胶混炼过程二维动态预测与控制
- 批准号:
- 批准年份:2022
- 资助金额:54 万元
- 项目类别:面上项目
基于子空间特征相似性的数据驱动主动学习控制
- 批准号:61873139
- 批准年份:2018
- 资助金额:66.0 万元
- 项目类别:面上项目
基于离线/在线数据的学习控制方法及其性能评价
- 批准号:60974040
- 批准年份:2009
- 资助金额:34.0 万元
- 项目类别:面上项目
相似国自然基金
{{ item.name }}
- 批准号:{{ item.ratify_no }}
- 批准年份:{{ item.approval_year }}
- 资助金额:{{ item.support_num }}
- 项目类别:{{ item.project_type }}
相似海外基金
{{
item.name }}
{{ item.translate_name }}
- 批准号:{{ item.ratify_no }}
- 财政年份:{{ item.approval_year }}
- 资助金额:{{ item.support_num }}
- 项目类别:{{ item.project_type }}