从水鸟的栖息地利用探讨人工栖息地对种群维持的作用
项目介绍
AI项目解读
基本信息
- 批准号:32170518
- 项目类别:面上项目
- 资助金额:60万
- 负责人:
- 依托单位:
- 学科分类:
- 结题年份:
- 批准年份:2021
- 项目状态:未结题
- 起止时间:2021至
- 项目参与者:马志军;
- 关键词:
项目摘要
结项摘要
项目成果
期刊论文数量(0)
专著数量(0)
科研奖励数量(0)
会议论文数量(0)
专利数量(0)
数据更新时间:{{ journalArticles.updateTime }}
{{
item.title }}
{{ item.translation_title }}
- DOI:{{ item.doi || "--"}}
- 发表时间:{{ item.publish_year || "--" }}
- 期刊:{{ item.journal_name }}
- 影响因子:{{ item.factor || "--"}}
- 作者:{{ item.authors }}
- 通讯作者:{{ item.author }}
数据更新时间:{{ journalArticles.updateTime }}
{{ item.title }}
- 作者:{{ item.authors }}
数据更新时间:{{ monograph.updateTime }}
{{ item.title }}
- 作者:{{ item.authors }}
数据更新时间:{{ sciAawards.updateTime }}
{{ item.title }}
- 作者:{{ item.authors }}
数据更新时间:{{ conferencePapers.updateTime }}
{{ item.title }}
- 作者:{{ item.authors }}
数据更新时间:{{ patent.updateTime }}
其他文献
铁电隧道结的研究进展
- DOI:--
- 发表时间:--
- 期刊:材料导报
- 影响因子:--
- 作者:潘瑞琨;章天金;杨凤霞;马志军;何苗
- 通讯作者:何苗
Pt/MgO/Pb0.2Zr0.8TiO3/SrRuO3复合铁电隧道结的阻变特性研究
- DOI:--
- 发表时间:2019
- 期刊:湖北大学学报(自然科学版)
- 影响因子:--
- 作者:李立谦;王一豪;章天金;马志军
- 通讯作者:马志军
Fluoride ion adsorption from wastewater using Magnesium(II), aluminum(III) and titanium(IV) modified natural zeolite: kinetics, thermodynamics, and mechanistic aspects of mechanistic adsorption
使用镁 (II)、铝 (III) 和钛 (IV) 改性天然沸石吸附废水中的氟离子:机械吸附的动力学、热力学和机理方面
- DOI:--
- 发表时间:--
- 期刊:Journal of Water Reuse and Desalination
- 影响因子:2.3
- 作者:马志军;张琪
- 通讯作者:张琪
基于毛细不稳定性的聚焦通道微液滴操控研究
- DOI:--
- 发表时间:2015
- 期刊:轻工机械
- 影响因子:--
- 作者:刘丹丹;徐玉梅;杨健;马志军;丁显廷
- 通讯作者:丁显廷
鄱阳湖四种水鸟的栖息地利用与水深和食物的关系
- DOI:--
- 发表时间:2014
- 期刊:动物学杂志
- 影响因子:--
- 作者:陈家宽;吴建东;刘观华;马志军
- 通讯作者:马志军
其他文献
{{
item.title }}
{{ item.translation_title }}
- DOI:{{ item.doi || "--" }}
- 发表时间:{{ item.publish_year || "--"}}
- 期刊:{{ item.journal_name }}
- 影响因子:{{ item.factor || "--" }}
- 作者:{{ item.authors }}
- 通讯作者:{{ item.author }}
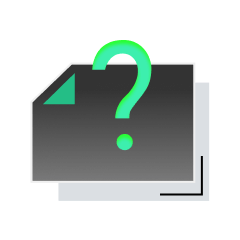
内容获取失败,请点击重试
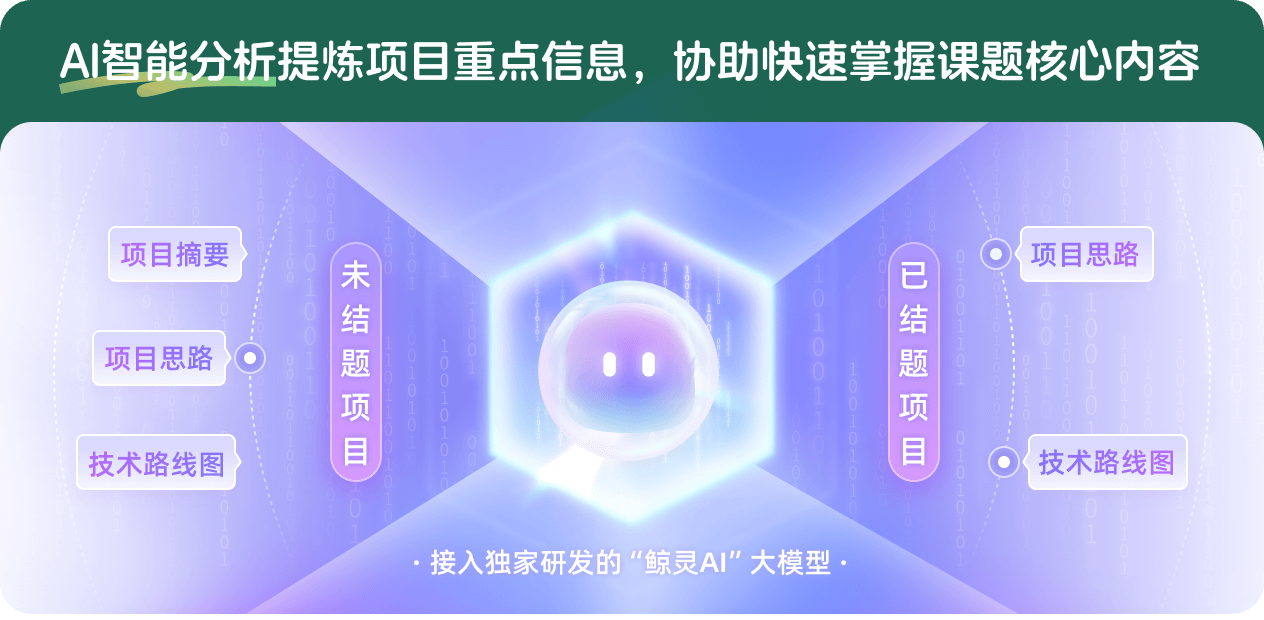
查看分析示例
此项目为未结题,我已根据课题信息分析并撰写以下内容,帮您拓宽课题思路:
AI项目摘要
AI项目思路
AI技术路线图
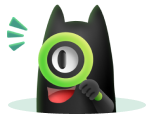
请为本次AI项目解读的内容对您的实用性打分
非常不实用
非常实用
1
2
3
4
5
6
7
8
9
10
您认为此功能如何分析更能满足您的需求,请填写您的反馈:
马志军的其他基金
人鸟冲突的发生原因、影响后果及减缓对策
- 批准号:32370544
- 批准年份:2023
- 资助金额:50 万元
- 项目类别:面上项目
候鸟迁徙活动的季节差异和年际差异:以中杓鹬为例
- 批准号:31772467
- 批准年份:2017
- 资助金额:62.0 万元
- 项目类别:面上项目
迁徙停歇地的食物资源变化对鸻鹬类食物利用、能量积累及活动性的影响
- 批准号:31572280
- 批准年份:2015
- 资助金额:68.0 万元
- 项目类别:面上项目
外来植物互花米草入侵盐沼湿地对繁殖鸟类的影响及其机理
- 批准号:31272334
- 批准年份:2012
- 资助金额:90.0 万元
- 项目类别:面上项目
鸻鹬类的迁徙停歇生态学研究:食物来源、组成及能量积累
- 批准号:31071939
- 批准年份:2010
- 资助金额:34.0 万元
- 项目类别:面上项目
鸻鹬类在迁徙停歇地的雄性早现及其生态影响
- 批准号:30670269
- 批准年份:2006
- 资助金额:29.0 万元
- 项目类别:面上项目
黑腹滨鹬对中途停歇地的利用模式及迁徙对策
- 批准号:30400057
- 批准年份:2004
- 资助金额:19.0 万元
- 项目类别:青年科学基金项目
相似国自然基金
{{ item.name }}
- 批准号:{{ item.ratify_no }}
- 批准年份:{{ item.approval_year }}
- 资助金额:{{ item.support_num }}
- 项目类别:{{ item.project_type }}
相似海外基金
{{
item.name }}
{{ item.translate_name }}
- 批准号:{{ item.ratify_no }}
- 财政年份:{{ item.approval_year }}
- 资助金额:{{ item.support_num }}
- 项目类别:{{ item.project_type }}