面向多特征纵向-生存数据建模及BFH推断研究
项目介绍
AI项目解读
基本信息
- 批准号:81671633
- 项目类别:面上项目
- 资助金额:48.0万
- 负责人:
- 依托单位:
- 学科分类:H1110.区域免疫及黏膜免疫疾病
- 结题年份:2020
- 批准年份:2016
- 项目状态:已结题
- 起止时间:2017-01-01 至2020-12-31
- 项目参与者:Huang Yangxin; 胡复艳; 彭幸春; 张增; 石雨; 魏林晓; 王超; 冯德欣; 陈伟;
- 关键词:
项目摘要
It often happens in longitudinal studies of its application that repeated measurements of markers are observed with various data features of multilevel structure, heterogeneity, non-normality, missingness and mismeasured covariates. Longitudinal data with all the above features combined with time to event survival data modeling and statistical inference analysis, it no doubt is a very difficult challenging project. However, relatively few studies have been conducted on simultaneously accounting for the biases induced by these data features and it is not clear how they may interact and simultaneously influence inferential procedures. Thus, this proposal explores a finite mixture of hierarchical joint models of longitudinal measures with an attempt to alleviate departures from homogeneous characteristics, adjust departures from normality, tailor missing observations and mediate accuracy from measurement error in covariate. The Bayesian-Frequency Hybrid methods are employed to not only estimate all parameters in mixture of hierarchical joint models and its large sample properties, but also evaluate probabilities of class membership. The proposed modeling procedure is applied to analyze real data and simulation studies are conducted to assess the performance of the proposed models and method. In the meantime, associated statistical package will be developed. US NIH AIDS clinical trials and the Framingham data are employed to study the dynamics of HIV infection and immune suppression by the models of this project. Finally we construct an application and promotion practices platform from our results, it provides a methodological reference for disease risk assessment and individual treatment, health big longitudinal - survival data mining, and evaluating the effects of health policy.
同时存在多水平结构、异质性、非正态分布、数据缺失、协变量测量误差等多特征的纵向数据在应用中较为常见。对具有全部上述特征的纵向数据和事件时间生存数据联合建模及统计推断分析,无疑是一个极具挑战性的困难课题。目前很少有研究探讨这些特征同时存在时产生的偏倚及对统计推断的联合影响。为此,本研究拟构建纵向数据包含高水平随机效应、偏斜分布、应变量和/或协变量缺失、多维时协变量测量误差以及生存数据的分层混合联合模型,并采用贝叶斯频率混合(BFH)统计方法对该模型进行统计推断研究,开发相应的统计软件包,并通过美国NIH AIDS临床试验和弗莱明翰研究数据基于所构建的模型对HIV感染动力学以及免疫抑制问题进行实证研究。提供模型的应用与推广实践平台,为疾病风险评估与个体化治疗、大型纵向-生存数据研究的数据分析等提供方法学支持。
结项摘要
复杂特征纵向数据在大规模流行病学纵向调查、多中心临床试验及多学科合作的队列研究中较为常见,事件时间生存数据多与纵向响应过程有关,研究复杂纵向数据和事件时间生存数据联合建模及统计推断分析具有重要的科学意义。.项目建立非线性贝叶斯分位数回归混合效应联合模型研究了具有事件时间的多特征纵向数据,通过贝叶斯统计方法同时估计联合模型中所有参数,纵向响应服从非对称拉普拉斯分布,在当前信息缺失下建立带有偏t分布的误差协变量的线性混合效应模型,对时间事件不可识别的非参数分布建立加速失效时间模型,并对艾滋病临床数据集进行了模拟研究;建立了具有异质性、非正态和缺失的纵向免疫数据的分层混合模型,通过艾滋病临床资料实例,论证了所提出方法的合理性;研究了同时具有异质性、非正态性、LOD或协变量误差特征的复杂纵向数据建模,运用贝叶斯非线性有限混合的建模方法,建立了同时具有偏斜分布的混合效应Tobit联合模型,实证研究中评估了所提出方法的性能;提出了有限混合偏态分布的非线性混合效应模型与二元逻辑模型的联合模型,通过模拟研究评估所提出模型和方法的性能,运用艾滋病临床试验研究的真例证明了该方法的可行性;建立了由个别潜在类指标链接的联合模型,即基于纵向数据建立有限混合的NLME模型和基于时间-事件数据建立比例危险Cox模型,运用艾滋病临床试验数据评估了所提出的联合模型性能;对存在协变量测量误差的偏正态分布纵向数据、响应过程和协变量过程联合建立了NLME模型,采用贝叶斯-频率混合方法进行了研究和分析,在艾滋病临床试验实证研究中,通过病毒动力学建模比较了潜在模型与不同的统计推断方法,仿真研究中评估了所提出模型和方法的性能;纵向轨迹模式的变化率和变化点的时间是量化纵向剖面对事件风险影响的关键指标,为了准确估计该项指标,项目在贝叶斯框架下建立了多元纵向和事件时间数据分段联合模型,并运用实例和模拟研究评估了所建议模型的性能。
项目成果
期刊论文数量(15)
专著数量(0)
科研奖励数量(0)
会议论文数量(0)
专利数量(0)
Simultaneous Bayesian inference on a finite mixture of mixed-effects Tobit joint models for longitudinal data with multiple features
对具有多个特征的纵向数据的混合效应 Tobit 联合模型的有限混合进行同步贝叶斯推理
- DOI:10.4310/sii.2017.v10.n4.a3
- 发表时间:2017
- 期刊:Statistics and Its Interface
- 影响因子:0.8
- 作者:Huang Yangxin;Chen Jiaqing;Yin Ping;Qiu Huahai
- 通讯作者:Qiu Huahai
Bayesian joint analysis of heterogeneous- and skewed-longitudinal data and a binary outcome, with application to AIDS clinical studies
异质和倾斜纵向数据和二元结果的贝叶斯联合分析,及其在艾滋病临床研究中的应用
- DOI:10.1177/0962280217689852
- 发表时间:2018-10
- 期刊:Statistical Methods in Medical Research
- 影响因子:2.3
- 作者:Lu Xiaosun;Huang Yangxin;Chen Jiaqing;Zhou Rong;Yu Shuli;Yin Ping
- 通讯作者:Yin Ping
污染数据情形下线性指数分布参数的经验贝叶斯估计(英文)
- DOI:10.13642/j.cnki.42-1184/o1.2018.04.080
- 发表时间:2018
- 期刊:应用数学
- 影响因子:--
- 作者:陈家清;王玉;刘次华
- 通讯作者:刘次华
Bayesian Change-Point Joint Models for Multivariate Longitudinal and Time-to-Event Data
多元纵向和事件时间数据的贝叶斯变点联合模型
- DOI:10.1080/19466315.2020.1837234
- 发表时间:2020-11-19
- 期刊:STATISTICS IN BIOPHARMACEUTICAL RESEARCH
- 影响因子:1.8
- 作者:Chen, Jiaqing;Huang, Yangxin;Tang, Nian-Sheng
- 通讯作者:Tang, Nian-Sheng
熵损失函数下Burr(α)X分布参数的贝叶斯估计
- DOI:10.13546/j.cnki.tjyjc.2017.20.001
- 发表时间:2017
- 期刊:统计与决策
- 影响因子:--
- 作者:陈家清;王玉;陈志强
- 通讯作者:陈志强
数据更新时间:{{ journalArticles.updateTime }}
{{
item.title }}
{{ item.translation_title }}
- DOI:{{ item.doi || "--"}}
- 发表时间:{{ item.publish_year || "--" }}
- 期刊:{{ item.journal_name }}
- 影响因子:{{ item.factor || "--"}}
- 作者:{{ item.authors }}
- 通讯作者:{{ item.author }}
数据更新时间:{{ journalArticles.updateTime }}
{{ item.title }}
- 作者:{{ item.authors }}
数据更新时间:{{ monograph.updateTime }}
{{ item.title }}
- 作者:{{ item.authors }}
数据更新时间:{{ sciAawards.updateTime }}
{{ item.title }}
- 作者:{{ item.authors }}
数据更新时间:{{ conferencePapers.updateTime }}
{{ item.title }}
- 作者:{{ item.authors }}
数据更新时间:{{ patent.updateTime }}
其他文献
其他文献
{{
item.title }}
{{ item.translation_title }}
- DOI:{{ item.doi || "--" }}
- 发表时间:{{ item.publish_year || "--"}}
- 期刊:{{ item.journal_name }}
- 影响因子:{{ item.factor || "--" }}
- 作者:{{ item.authors }}
- 通讯作者:{{ item.author }}
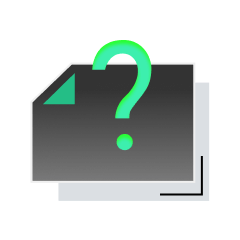
内容获取失败,请点击重试
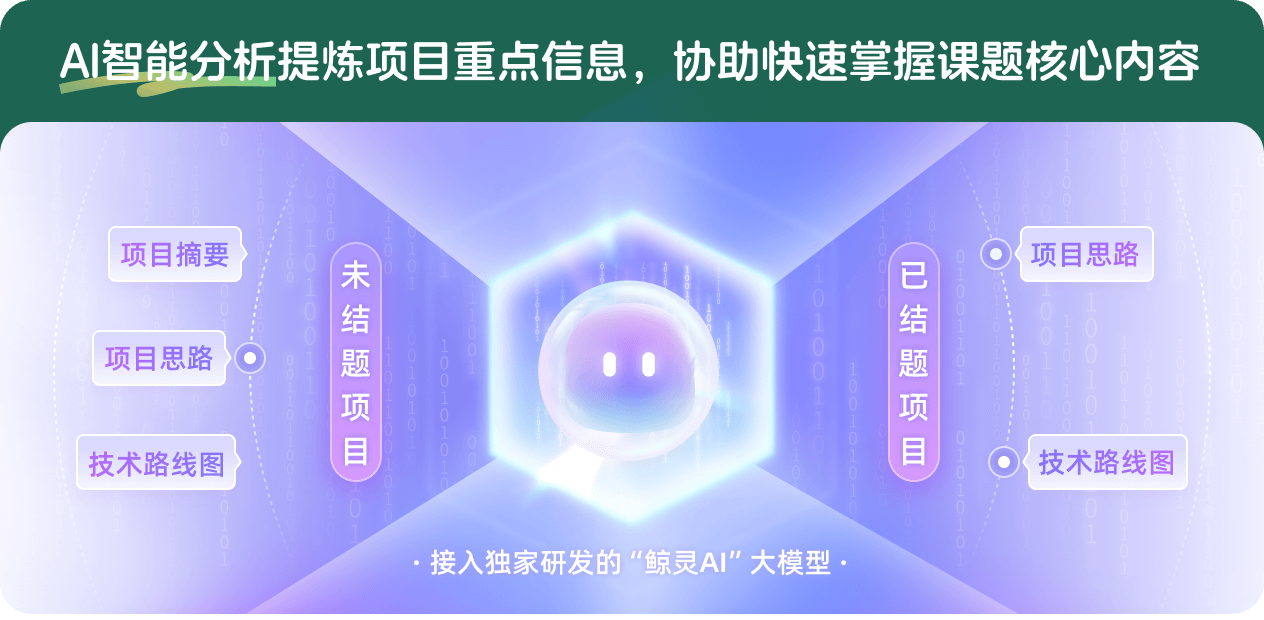
查看分析示例
此项目为已结题,我已根据课题信息分析并撰写以下内容,帮您拓宽课题思路:
AI项目摘要
AI项目思路
AI技术路线图
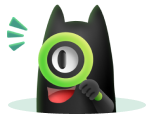
请为本次AI项目解读的内容对您的实用性打分
非常不实用
非常实用
1
2
3
4
5
6
7
8
9
10
您认为此功能如何分析更能满足您的需求,请填写您的反馈:
相似国自然基金
{{ item.name }}
- 批准号:{{ item.ratify_no }}
- 批准年份:{{ item.approval_year }}
- 资助金额:{{ item.support_num }}
- 项目类别:{{ item.project_type }}
相似海外基金
{{
item.name }}
{{ item.translate_name }}
- 批准号:{{ item.ratify_no }}
- 财政年份:{{ item.approval_year }}
- 资助金额:{{ item.support_num }}
- 项目类别:{{ item.project_type }}