复杂环境下稀疏传感器网络的分布式跟踪与融合方法研究
项目介绍
AI项目解读
基本信息
- 批准号:61901151
- 项目类别:青年科学基金项目
- 资助金额:25.0万
- 负责人:
- 依托单位:
- 学科分类:F0104.通信网络
- 结题年份:2022
- 批准年份:2019
- 项目状态:已结题
- 起止时间:2020-01-01 至2022-12-31
- 项目参与者:--
- 关键词:
项目摘要
As a new application of sensor networks, distributed tracking and fusion technique enjoys the capability of decentralization, high scalability and system fault tolerance, which is potential to be implemented into the real tracking system. When the sensor network deploys with sparse topology in the harsh environment, the local tracking robustness and practicability are usually very poor, the fusion accuracy is low and the communication burden between nodes is also very heavy, in order to address those problems, this research is going to: (1) develop an approach to quantificationally model the multi-state based propagation mechanism of target existence in time domain, aimed to robustly maintain the true track when target being heavily occluded. (2) develop an multi-scan automatic target tracking approach based on the integrated track branching, aimed to improve the tracking performance in heavy cluttered environment as well as satisfying the practical requirement on aspects of computation、storage load and thresholding. (3) develop a probabilistic weighting based adaptive double consensus distributed fusion method, aimed to improve the fusion accuracy and alleviate the communication load between neighboring nodes in the environment of sparse topology and clutter presence. (4) utilize the active/passive sonar network real data as well as the acoustic array simulation platform generated data to jointly verify the availability and practices ability of the proposed methods. This project along with its expected achievements will tangibly enrich the research of large scale sensor networks, as we as pave the way for realistic target tracking technology using large scale sparse sensor networks in harsh environment.
分布式跟踪与融合是传感器网络的新型应用领域,具备高可扩展性与容错性、去中心化能力,可满足大规模传感器网络对目标跟踪的实用化需求。针对复杂环境下稀疏传感器网络本节点跟踪鲁棒性与实用性差、节点间融合精度低与通信量高的问题,本课题拟重点研究:(1)基于遮挡目标多状态时域演变过程建模与计算方法,以提升严重遮挡情况下的目标航迹鲁棒维持能力。(2)基于综合航迹拆分的本节点多帧目标自动跟踪技术,以提升高杂波环境下的自动跟踪性能并满足算法在计算量、存储量与调参难度方面的实用化需求。(3)基于概率加权的自适应双一致性分布式融合方法,以提升杂波干扰下的稀疏传感器网络融合精度并减少节点间通信量。(4)利用主被动声呐网实测数据与声阵列仿真数据对所提方法的有效性与实用性进行全面验证。该项目研究及其成果将丰富大规模传感器网络的研究内涵,对稀疏传感器网络在复杂环境下的目标跟踪应用有重要意义。
结项摘要
分布式跟踪与融合技术是大型传感器网络的新型应用领域之一,具备高可扩展性与容错性、去中心化能力,可满足大规模传感器网络对目标跟踪的实用化需求,近年来被广泛应用于广域搜索与监视、环境监控等军民领域。本项目针对复杂环境下稀疏传感器网络分布式跟踪与融合面临的关键科学问题,分别从复杂环境下本节点自动跟踪、稀疏传感器网络分布式融合两个关键环节共开展了五个方面的研究,取得的主要成果有:1)构建了遮挡目标存在多状态时域演变模型,实现了遮挡目标存在状态的精细化表征与精确建模;2)提出了基于目标存在多状态和自适应状态的遮挡目标鲁棒自动跟踪算法,提升了遮挡目标航迹的快速起始与鲁棒维持能力;3)提出了基于航迹假设拆分的本节点多帧自动跟踪算法,实现了密集杂波干扰下的目标航迹稳健维持,提升了虚假航迹的抑制能力;4)提出了基于概率加权多步双一致与最大化一致的分布式融合算法,实现了传感网拓扑稀疏、杂波干扰下的多源信息高效融合,提升了稀疏传感网节点的探测范围与跟踪一致性;5)提出了基于双向解相关的多源乱序信息融合算法,实现了通信时延下的多源信息高效融合,显著提高了融合中心对乱序信息的利用率。. 本项目在目标跟踪与多传感器信息融合领域取得的一系列成果,对大规模稀疏传感器网络在复杂环境下的目标跟踪应用具有推动意义。在项目执行期间,共发表期刊论文6篇,其中发表在Aerospace Science and Technology等高质量SCI期刊4篇,发表在传感技术学报、现代雷达中文核心期刊2篇,申请国家发明专利5项,已授权2项,培养硕士研究生8名,已顺利毕业3名。
项目成果
期刊论文数量(6)
专著数量(0)
科研奖励数量(0)
会议论文数量(0)
专利数量(2)
Multi-Sonar Distributed Fusion for Target Detection and Tracking in Marine Environment.
海洋环境中目标检测与跟踪的多声纳分布式融合
- DOI:10.3390/s22093335
- 发表时间:2022-04-27
- 期刊:Sensors (Basel, Switzerland)
- 影响因子:--
- 作者:
- 通讯作者:
Distributed fusion in harsh environments using multiple bearings-only sensors with out-of-sequence-refined measurements
使用多个仅轴承传感器和无序精细测量在恶劣环境中进行分布式融合
- DOI:10.1016/j.ast.2021.106950
- 发表时间:2021-07-26
- 期刊:AEROSPACE SCIENCE AND TECHNOLOGY
- 影响因子:5.6
- 作者:Shi, Yifang;Farina, Alfonso;Guo, Yunfei
- 通讯作者:Guo, Yunfei
复杂环境下针对遮挡目标的鲁棒自动跟踪方法
- DOI:--
- 发表时间:2022
- 期刊:传感技术学报
- 影响因子:--
- 作者:石义芳;方伟业;周福珍;郭云飞
- 通讯作者:郭云飞
Multisensor distributed out-of-sequence-tracks fusion with track origin uncertainty
具有航迹原点不确定性的多传感器分布式失序航迹融合
- DOI:10.1016/j.ast.2020.106226
- 发表时间:2020-11-01
- 期刊:AEROSPACE SCIENCE AND TECHNOLOGY
- 影响因子:5.6
- 作者:Shi, Yifang;Ullah, Ihsan;Choi, Jee Woong
- 通讯作者:Choi, Jee Woong
Distributed Target Tracking in Challenging Environments Using Multiple Asynchronous Bearing-Only Sensors
使用多个异步仅方位传感器在具有挑战性的环境中进行分布式目标跟踪
- DOI:10.3390/s20092671
- 发表时间:2020-05-01
- 期刊:SENSORS
- 影响因子:3.9
- 作者:Shi, Yifang;Choi, Jee Woong;Khan, Uzair
- 通讯作者:Khan, Uzair
数据更新时间:{{ journalArticles.updateTime }}
{{
item.title }}
{{ item.translation_title }}
- DOI:{{ item.doi || "--"}}
- 发表时间:{{ item.publish_year || "--" }}
- 期刊:{{ item.journal_name }}
- 影响因子:{{ item.factor || "--"}}
- 作者:{{ item.authors }}
- 通讯作者:{{ item.author }}
数据更新时间:{{ journalArticles.updateTime }}
{{ item.title }}
- 作者:{{ item.authors }}
数据更新时间:{{ monograph.updateTime }}
{{ item.title }}
- 作者:{{ item.authors }}
数据更新时间:{{ sciAawards.updateTime }}
{{ item.title }}
- 作者:{{ item.authors }}
数据更新时间:{{ conferencePapers.updateTime }}
{{ item.title }}
- 作者:{{ item.authors }}
数据更新时间:{{ patent.updateTime }}
其他文献
其他文献
{{
item.title }}
{{ item.translation_title }}
- DOI:{{ item.doi || "--" }}
- 发表时间:{{ item.publish_year || "--"}}
- 期刊:{{ item.journal_name }}
- 影响因子:{{ item.factor || "--" }}
- 作者:{{ item.authors }}
- 通讯作者:{{ item.author }}
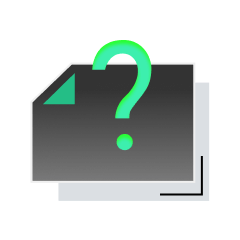
内容获取失败,请点击重试
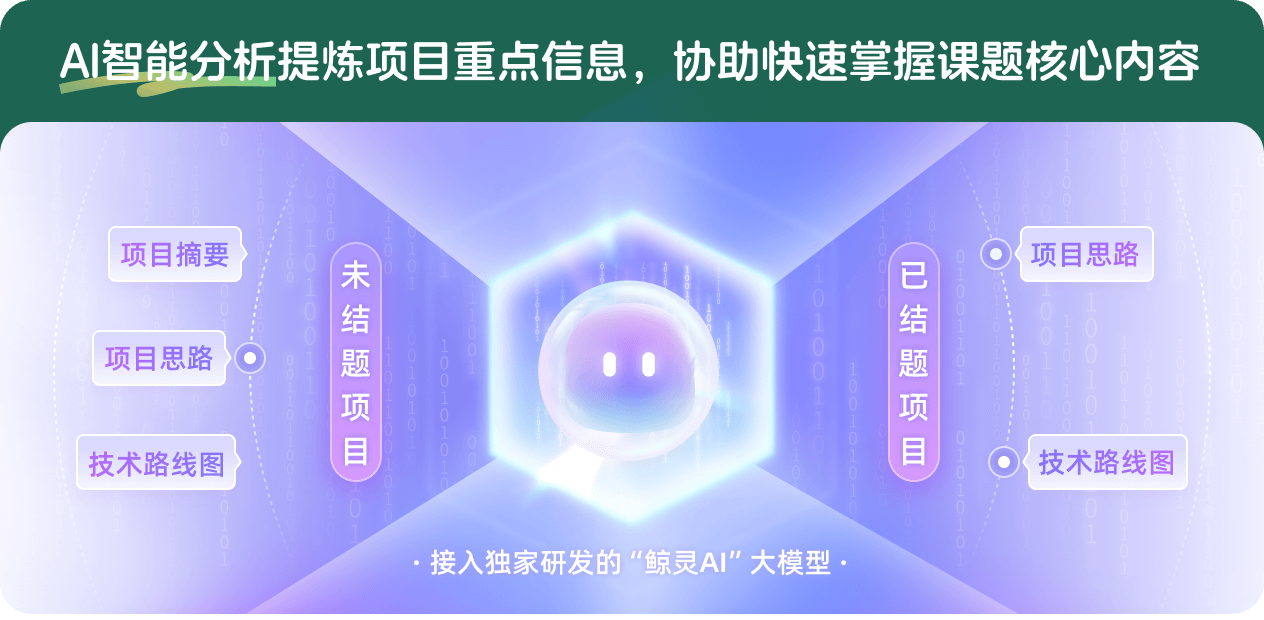
查看分析示例
此项目为已结题,我已根据课题信息分析并撰写以下内容,帮您拓宽课题思路:
AI项目摘要
AI项目思路
AI技术路线图
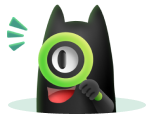
请为本次AI项目解读的内容对您的实用性打分
非常不实用
非常实用
1
2
3
4
5
6
7
8
9
10
您认为此功能如何分析更能满足您的需求,请填写您的反馈:
相似国自然基金
{{ item.name }}
- 批准号:{{ item.ratify_no }}
- 批准年份:{{ item.approval_year }}
- 资助金额:{{ item.support_num }}
- 项目类别:{{ item.project_type }}
相似海外基金
{{
item.name }}
{{ item.translate_name }}
- 批准号:{{ item.ratify_no }}
- 财政年份:{{ item.approval_year }}
- 资助金额:{{ item.support_num }}
- 项目类别:{{ item.project_type }}