分布式密码系统中的基础协议与公平性研究
项目介绍
AI项目解读
基本信息
- 批准号:60963023
- 项目类别:地区科学基金项目
- 资助金额:22.0万
- 负责人:
- 依托单位:
- 学科分类:F0206.信息安全
- 结题年份:2012
- 批准年份:2009
- 项目状态:已结题
- 起止时间:2010-01-01 至2012-12-31
- 项目参与者:罗文俊; 汪学明; 杨云江; 张弘; 张晓培; 樊玫玫; 田有亮; 李玉凯; 张仁平;
- 关键词:
项目摘要
课题的目标是寻求新的方法和突破口对分布式密码系统的某些关键问题进行研究,具体内容为:(1)、以双线性对为工具及切入点,利用双线性对的相关特性,设计新的知识证明协议、知识承诺方案、可验证秘密共享方案和可公开验证秘密共享方案,以及具有同态性的线性秘密共享方案,同时基于双线对探索一般访问结构的秘密共享方案;(2)、将双线性对引入到安全多方计算协议中,利用所设计的相关基础工具和协议,设计新的安全多方计算协议。(3)、探讨安全多方计算协议的的公平性,寻找新的方法研究某些特殊安全多方计算协议的公平性,为多方公平交换协议提供更一般的理论框架。(3)、将机制设计理论的思想和方法,引入到公平交换协议的公平性研究中,试图从目标收益达到平衡状态的角度来定义一种新的公平交换机制,并以此为基础研究多方公平交换协议。
结项摘要
项目围绕以秘密共享和安全多方计算为核心的分布式密码系统基础协议的一些关键问题展开研究;探索了基础协议的公平性和公平协议设计;以此为基础,研究面向多方的应用密码协议;设计了一个测试仿真基础平台,完成了基础算法实现。具体包括:(1)、以双线性对良好的数学和密码学特性为切入点,通过设计新的知识证明协议和知识承诺方案,构建了相关可验证秘密共享方案、可公开验证秘密共享方案;初步探讨了基于同态密码的知识证明协议,得到了基于同态密码的零知识证明协议;(2)、将双线性对引入到安全多方计算协议,基于双线性对实现了保密加法和保密乘法计算,并进一步设计了基于双线性对的安全多方计算协议;(3)、提出了一种群组通信的通用可组合机制,为分布式密码系统基础协议的通用可组合安全提供了思路和方法;初步从信息熵的角度探讨了门限结构和一般访问结构的秘密共享,并给出了相关形式化描述;(4)、从概率的角度提出了公平的秘密共享方案;探讨了安全多方计算公平性的定义及一些关键问题,并基于Boolean电路研究通用安全多方计算的公平性,设计了某种条件下的通用公平安全多方计算协议;(5)、鉴于传统协议公平性实现的困难,研究了具有理性参与者的秘密共享协议和安全多方计算协议的效用机制,提出了基于博弈平衡机制的公平性概念;探讨了分布式密码系统基础协议的效用机制设计,初步利用基本博弈、重复博弈、贝叶斯博弈和信誉机制等方法,部分解决了秘密共享中的“空威胁”和“搭便车”问题,给出了适用于秘密共享协议和安全多方计算协议的一些公平机制设计,实现了一些理性公平秘密共享协议和理性公平安全多方计算协议;(6)、在多方应用密码协议方面,围绕群组通信的安全需求,集中在多重签名及签章、门限签名及签章、结构化签名、聚集签名、可传递签名、群签名、基于属性密码体制、多方模糊身份密码体制、多方的无证书密码和基于证书密码体制、分布式云存储和多方电子合同协议等方面展开了研究;(7)、设计并实现了一个测试仿真基础平台,完成了Edwards型椭圆曲线密码、双线性对和基于身份密码的一些基础算法;实现了PKI核心组件设计,构建了面向多方的PKI/CA的公钥密码基础平台,并进行了部分协议的仿真实验。. 项目组在分布式密码系统的理论、方法和应用上有了一定的突破和创新,圆满完成了项目计划任务和目标,在科学研究、人才培养和团队建设方面取得了较好的效果。
项目成果
期刊论文数量(24)
专著数量(0)
科研奖励数量(0)
会议论文数量(6)
专利数量(1)
二进制Edwards曲线上的点压缩算法
- DOI:--
- 发表时间:--
- 期刊:贵州大学学报(自然科学版)
- 影响因子:--
- 作者:丁红发;彭长根;杨震;张晓培
- 通讯作者:张晓培
一种改进的存在特权集的门限群签名方案
- DOI:--
- 发表时间:--
- 期刊:计算机工程与科学
- 影响因子:--
- 作者:董玉蓉;汪学明
- 通讯作者:汪学明
公平的电子合同签署协议的博弈分析与改进
- DOI:--
- 发表时间:--
- 期刊:计算机工程与设计
- 影响因子:--
- 作者:翁立晨;汪学明
- 通讯作者:汪学明
秘密共享体制的博弈论分析
- DOI:--
- 发表时间:--
- 期刊:电子学报
- 影响因子:--
- 作者:田有亮;马建峰;彭长根;姬文江
- 通讯作者:姬文江
无随机预言模型下基于模糊身份的签名方案
- DOI:--
- 发表时间:--
- 期刊:计算机工程与应用
- 影响因子:--
- 作者:高建平;彭长根;石伟敏;洪流
- 通讯作者:洪流
数据更新时间:{{ journalArticles.updateTime }}
{{
item.title }}
{{ item.translation_title }}
- DOI:{{ item.doi || "--"}}
- 发表时间:{{ item.publish_year || "--" }}
- 期刊:{{ item.journal_name }}
- 影响因子:{{ item.factor || "--"}}
- 作者:{{ item.authors }}
- 通讯作者:{{ item.author }}
数据更新时间:{{ journalArticles.updateTime }}
{{ item.title }}
- 作者:{{ item.authors }}
数据更新时间:{{ monograph.updateTime }}
{{ item.title }}
- 作者:{{ item.authors }}
数据更新时间:{{ sciAawards.updateTime }}
{{ item.title }}
- 作者:{{ item.authors }}
数据更新时间:{{ conferencePapers.updateTime }}
{{ item.title }}
- 作者:{{ item.authors }}
数据更新时间:{{ patent.updateTime }}
其他文献
隐私保护的信息熵模型及其度量方法
- DOI:10.13328/j.cnki.jos.005096
- 发表时间:2016
- 期刊:软件学报
- 影响因子:--
- 作者:彭长根;丁红发;朱义杰;田有亮;符祖峰
- 通讯作者:符祖峰
基于身份的可验证密钥的公钥内积函数加密算法
- DOI:10.11897/sp.j.1016.2021.00209
- 发表时间:2021
- 期刊:计算机学报
- 影响因子:--
- 作者:邓宇乔;宋歌;杨波;彭长根;唐春明;温雅敏
- 通讯作者:温雅敏
理性公平的秘密共享方案
- DOI:--
- 发表时间:2020
- 期刊:计算机学报
- 影响因子:--
- 作者:刘海;李兴华;田有亮;雒彬;马建峰;彭长根
- 通讯作者:彭长根
基于Edwards曲线的移动RFID安全认证协议
- DOI:--
- 发表时间:2014
- 期刊:通信学报
- 影响因子:--
- 作者:杨玉龙;彭长根;周洲;张晓培
- 通讯作者:张晓培
基于口令的密钥协商协议的改进与设计
- DOI:--
- 发表时间:2014
- 期刊:信息网络安全
- 影响因子:--
- 作者:周洲;彭长根;杨玉龙
- 通讯作者:杨玉龙
其他文献
{{
item.title }}
{{ item.translation_title }}
- DOI:{{ item.doi || "--" }}
- 发表时间:{{ item.publish_year || "--"}}
- 期刊:{{ item.journal_name }}
- 影响因子:{{ item.factor || "--" }}
- 作者:{{ item.authors }}
- 通讯作者:{{ item.author }}
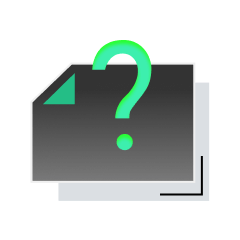
内容获取失败,请点击重试
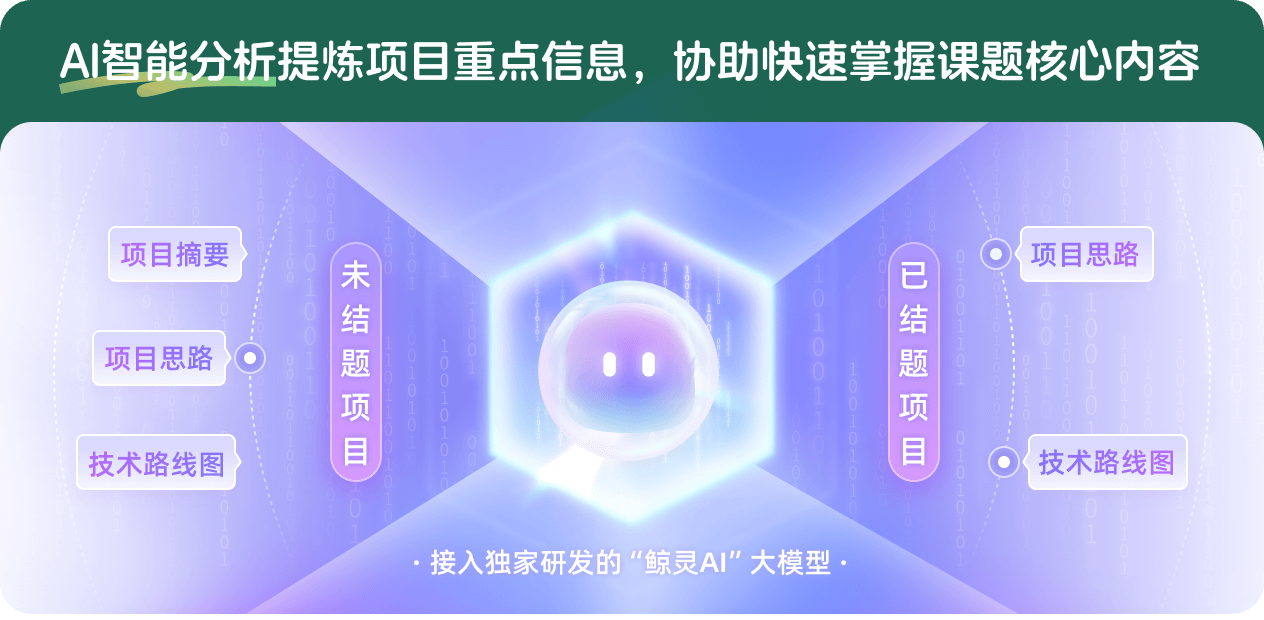
查看分析示例
此项目为已结题,我已根据课题信息分析并撰写以下内容,帮您拓宽课题思路:
AI项目摘要
AI项目思路
AI技术路线图
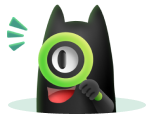
请为本次AI项目解读的内容对您的实用性打分
非常不实用
非常实用
1
2
3
4
5
6
7
8
9
10
您认为此功能如何分析更能满足您的需求,请填写您的反馈:
彭长根的其他基金
访问控制函数加密体制及细粒度隐私计算理论与方法
- 批准号:62272124
- 批准年份:2022
- 资助金额:55 万元
- 项目类别:面上项目
数据共享应用的块数据融合分析理论与安全管控模型研究
- 批准号:U1836205
- 批准年份:2018
- 资助金额:243.0 万元
- 项目类别:联合基金项目
理性隐私计算及隐私风险可控技术研究
- 批准号:61662009
- 批准年份:2016
- 资助金额:43.0 万元
- 项目类别:地区科学基金项目
安全协议的理性模型与理性公平性及其机制设计方法研究
- 批准号:61262073
- 批准年份:2012
- 资助金额:46.0 万元
- 项目类别:地区科学基金项目
相似国自然基金
{{ item.name }}
- 批准号:{{ item.ratify_no }}
- 批准年份:{{ item.approval_year }}
- 资助金额:{{ item.support_num }}
- 项目类别:{{ item.project_type }}
相似海外基金
{{
item.name }}
{{ item.translate_name }}
- 批准号:{{ item.ratify_no }}
- 财政年份:{{ item.approval_year }}
- 资助金额:{{ item.support_num }}
- 项目类别:{{ item.project_type }}