载脂蛋白B100和载脂蛋白E在奶牛脂肪肝发生中的作用机制
项目介绍
AI项目解读
基本信息
- 批准号:30640049
- 项目类别:专项基金项目
- 资助金额:10.0万
- 负责人:
- 依托单位:
- 学科分类:C1801.基础兽医学
- 结题年份:2007
- 批准年份:2006
- 项目状态:已结题
- 起止时间:2007-01-01 至2007-12-31
- 项目参与者:李小兵; 孙玉成; 牛淑玲; 张才; 王雪莹; 宋文华; 姜玉富;
- 关键词:
项目摘要
以肝脏脂肪蓄积和脂肪变性为病理特征的奶牛脂肪肝是围产期奶牛重要的群发性常见多发病,且多发于高产奶牛,严重影响了奶牛的生产性能和经济效益。故一直被各国列为最重要的奶牛疾病而加以研究。本项目以奶牛脂肪肝发生的关键因素-肝脂转运的主要工具VLDL的组装和分泌为重点,从神经内分泌因子对VLDL组装和分泌的调控作用和VLDL主要结构蛋白ApoB100和ApoE基因表达二个层面,应用分子生物学和细胞生物学技术
结项摘要
项目成果
期刊论文数量(6)
专著数量(0)
科研奖励数量(0)
会议论文数量(2)
专利数量(0)
干乳期能量摄入水平对围产期奶牛
- DOI:--
- 发表时间:--
- 期刊:中国兽医学报,2006(3):320~322
- 影响因子:--
- 作者:孙玉成;王雪莹;李红梅;王 哲
- 通讯作者:王 哲
胰岛素、胰高血糖素对体外培养脂
- DOI:--
- 发表时间:--
- 期刊:中国兽医学报,2007(6):862~864
- 影响因子:--
- 作者:张永宏;孙玉成;李小兵;王 哲
- 通讯作者:王 哲
不同能量摄入水平对围产期奶牛肝
- DOI:--
- 发表时间:--
- 期刊:畜牧兽医学报,2006(4):342~347
- 影响因子:--
- 作者:孙玉成;王雪莹;李红梅;王 哲
- 通讯作者:王 哲
竞争RT-PCR方法检测奶牛肝载脂蛋
- DOI:--
- 发表时间:--
- 期刊:中国兽医杂志,2006(12):5~7
- 影响因子:--
- 作者:王雪莹;孙玉成;陈仕均;王 哲
- 通讯作者:王 哲
神经内分泌因子对体外培养新生犊
- DOI:--
- 发表时间:--
- 期刊:中国畜牧兽医,2007(8):45~48
- 影响因子:--
- 作者:张永宏;孙玉成;刘国文;李小兵
- 通讯作者:李小兵
数据更新时间:{{ journalArticles.updateTime }}
{{
item.title }}
{{ item.translation_title }}
- DOI:{{ item.doi || "--"}}
- 发表时间:{{ item.publish_year || "--" }}
- 期刊:{{ item.journal_name }}
- 影响因子:{{ item.factor || "--"}}
- 作者:{{ item.authors }}
- 通讯作者:{{ item.author }}
数据更新时间:{{ journalArticles.updateTime }}
{{ item.title }}
- 作者:{{ item.authors }}
数据更新时间:{{ monograph.updateTime }}
{{ item.title }}
- 作者:{{ item.authors }}
数据更新时间:{{ sciAawards.updateTime }}
{{ item.title }}
- 作者:{{ item.authors }}
数据更新时间:{{ conferencePapers.updateTime }}
{{ item.title }}
- 作者:{{ item.authors }}
数据更新时间:{{ patent.updateTime }}
其他文献
热喷涂Ni基金属陶瓷层的重熔处理研究进展与展望
- DOI:10.16577/j.cnki.42-1215/tb.2018.05.024
- 发表时间:2018
- 期刊:材料保护
- 影响因子:--
- 作者:李亚龙;董天顺;郑晓东;李小兵;周秀锴;李国禄
- 通讯作者:李国禄
“7•26”暴雨调查对黄土高原水土保持工作的启示
- DOI:10.14123/j.cnki.swcc.2021.0058
- 发表时间:2021
- 期刊:中国水土保持
- 影响因子:--
- 作者:党维勤;党恬敏;张泉;李小兵;田金梅
- 通讯作者:田金梅
ZnS纳米粒子的水热法和溶剂热法制备
- DOI:--
- 发表时间:--
- 期刊:压电与声光
- 影响因子:--
- 作者:李小兵;田 莳;孙玉静;何 亮;涂 湛
- 通讯作者:涂 湛
基于Zigbee的无线传感器网络教学平台设计
- DOI:--
- 发表时间:2014
- 期刊:实验室科学
- 影响因子:--
- 作者:丁明理;李小兵;李松泽
- 通讯作者:李松泽
长江上游干流及赤水河蛇遗传多样性与种群历史分析
- DOI:--
- 发表时间:2016
- 期刊:动物学杂志
- 影响因子:--
- 作者:李小兵;唐琼英;俞丹;刘焕章
- 通讯作者:刘焕章
其他文献
{{
item.title }}
{{ item.translation_title }}
- DOI:{{ item.doi || "--" }}
- 发表时间:{{ item.publish_year || "--"}}
- 期刊:{{ item.journal_name }}
- 影响因子:{{ item.factor || "--" }}
- 作者:{{ item.authors }}
- 通讯作者:{{ item.author }}
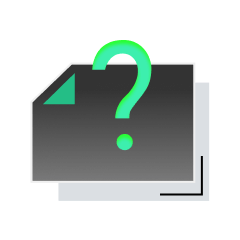
内容获取失败,请点击重试
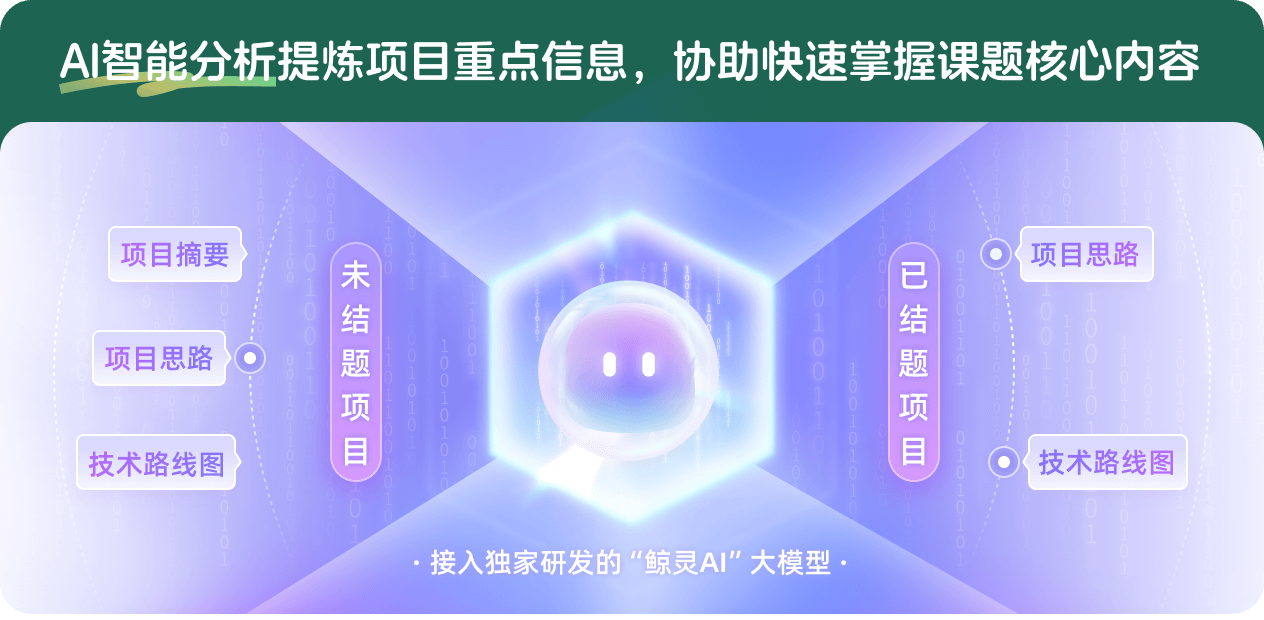
查看分析示例
此项目为已结题,我已根据课题信息分析并撰写以下内容,帮您拓宽课题思路:
AI项目摘要
AI项目思路
AI技术路线图
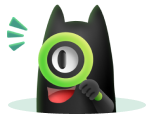
请为本次AI项目解读的内容对您的实用性打分
非常不实用
非常实用
1
2
3
4
5
6
7
8
9
10
您认为此功能如何分析更能满足您的需求,请填写您的反馈:
李小兵的其他基金
围产期奶牛高NEFA血症对脂肪组织脂代谢和分泌机能的影响
- 批准号:31572581
- 批准年份:2015
- 资助金额:64.0 万元
- 项目类别:面上项目
奶牛酮病肝脂代谢紊乱AMPK信号传导机制
- 批准号:31272621
- 批准年份:2012
- 资助金额:81.0 万元
- 项目类别:面上项目
PTEN和SREBP-1c基因调控对奶牛肝细胞脂肪沉积的影响
- 批准号:30972224
- 批准年份:2009
- 资助金额:31.0 万元
- 项目类别:面上项目
相似国自然基金
{{ item.name }}
- 批准号:{{ item.ratify_no }}
- 批准年份:{{ item.approval_year }}
- 资助金额:{{ item.support_num }}
- 项目类别:{{ item.project_type }}
相似海外基金
{{
item.name }}
{{ item.translate_name }}
- 批准号:{{ item.ratify_no }}
- 财政年份:{{ item.approval_year }}
- 资助金额:{{ item.support_num }}
- 项目类别:{{ item.project_type }}