m-项逼近的超级贪婪算法的性能分析
项目介绍
AI项目解读
基本信息
- 批准号:11701411
- 项目类别:青年科学基金项目
- 资助金额:18.0万
- 负责人:
- 依托单位:
- 学科分类:A0205.调和分析与逼近论
- 结题年份:2020
- 批准年份:2017
- 项目状态:已结题
- 起止时间:2018-01-01 至2020-12-31
- 项目参与者:陈宗青; 杨丽萍; 陈利满; 李健华; 张世杰;
- 关键词:
项目摘要
Greedy algorithm is one of the important tools of function approximation. We will make some investigations of the efficiency of some kinds of super greedy algorithms for m-term approximations. The focus of performance analysis is the approximation error and convergence. .At first, we will analyze the performance of greedy algorithms from the perspective of error. We establish the Lebesgue-type inequalities for super greedy algorithms with respect to dictionaries of Banach spaces to describe the approximation properties of these algorithms. Then, we analyze the convergence of the super greedy algorithm for compressed sensing. Besides, under the framework of infinite dimensional compressed sensing proposed by Ben Adcock and Anders Hansen, we study the recovery of asymptotic sparse signals for the weighted lp minmiziation and multilevel sampling. Our expected results will provide new direction for the future study of greedy approximation and will also be helpful for the developments of compressed sensing.
贪婪算法是函数逼近的重要工具之一,我们将研究m-项逼近的超级贪婪算法的性能。性能分析的侧重点是逼近误差和收敛性。我们首先从误差角度分析超级贪婪算法的性能。建立借助于给定Banach空间的字典的超级贪婪算法的Lebesgue型不等式,以深入地刻画它们的逼近性质。然后,研究用于压缩感知中的超级贪婪算法的收敛性。另外,我们在Ben Adcock和Anders Hansen建立的无限维压缩感知的框架下,考虑用带权lp极小化模型,给出在已知部分信号支撑信息的情况下的多层采样及重建算法的性能分析。我们的预期结果将为贪婪逼近的研究提供新的增长点,同时也对压缩感知的发展起到推进作用。
结项摘要
贪婪算法是函数逼近的重要工具之一, 其高效性和简洁性使得其被广泛的应用于压缩感知,凸优化,机器学习,信号处理等领域,成为工程领域被应用的最多的算法之一。在该项目期间,我们深入挖掘贪婪算法的理论基础,首先我们建立了在Hilbert空间借助于满足RIP字典的超级正交贪婪算法的Lebesgue型不等式,并在此基础上给出了A1类的收敛阶,且得到有噪声条件下,研究了超级贪婪算法恢复稀疏信号时的收敛性,该研究显示超级算法在一定程度上可以提高贪婪算法的效率。另外,2019年度我们给出了近似弱切比雪夫贪婪算法在一些特殊Banach空间的Lebesgue型不等式;特别的,我们对于Hilbert空间的情况给出了AWOMP算法的Lebesgue型不等式。最后在2020年度我们研究了从含噪声的群稀疏信号中如何恢复信号的问题,得到了在GRIP条件下GOMP算法的信号恢复性能的估计。我们的研究针对的是现实的情况中可能会遇到的各种问题,考虑了各种不理想的情况,如含噪声,近似逼近等,从而丰富了贪婪逼近的理论基础,扩大了其应用范围。
项目成果
期刊论文数量(1)
专著数量(0)
科研奖励数量(0)
会议论文数量(0)
专利数量(0)
Efficiency of orthogonal super greedy algorithm under the restricted isometry property
受限等距性质下正交超贪心算法的效率
- DOI:10.1186/s13660-019-2075-x
- 发表时间:2019
- 期刊:Journal of Inequalities and Applications
- 影响因子:1.6
- 作者:魏秀杰;叶培新
- 通讯作者:叶培新
数据更新时间:{{ journalArticles.updateTime }}
{{
item.title }}
{{ item.translation_title }}
- DOI:{{ item.doi || "--"}}
- 发表时间:{{ item.publish_year || "--" }}
- 期刊:{{ item.journal_name }}
- 影响因子:{{ item.factor || "--"}}
- 作者:{{ item.authors }}
- 通讯作者:{{ item.author }}
数据更新时间:{{ journalArticles.updateTime }}
{{ item.title }}
- 作者:{{ item.authors }}
数据更新时间:{{ monograph.updateTime }}
{{ item.title }}
- 作者:{{ item.authors }}
数据更新时间:{{ sciAawards.updateTime }}
{{ item.title }}
- 作者:{{ item.authors }}
数据更新时间:{{ conferencePapers.updateTime }}
{{ item.title }}
- 作者:{{ item.authors }}
数据更新时间:{{ patent.updateTime }}
其他文献
其他文献
{{
item.title }}
{{ item.translation_title }}
- DOI:{{ item.doi || "--" }}
- 发表时间:{{ item.publish_year || "--"}}
- 期刊:{{ item.journal_name }}
- 影响因子:{{ item.factor || "--" }}
- 作者:{{ item.authors }}
- 通讯作者:{{ item.author }}
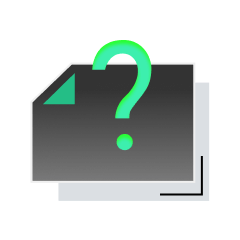
内容获取失败,请点击重试
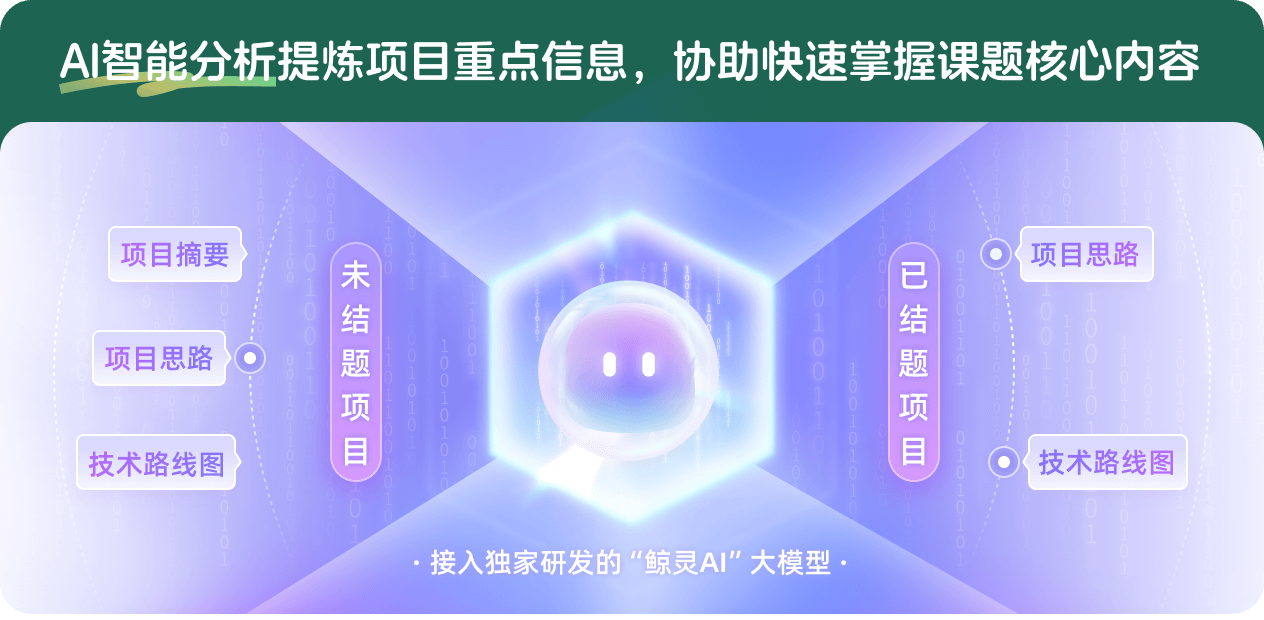
查看分析示例
此项目为已结题,我已根据课题信息分析并撰写以下内容,帮您拓宽课题思路:
AI项目摘要
AI项目思路
AI技术路线图
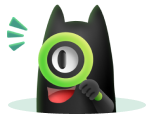
请为本次AI项目解读的内容对您的实用性打分
非常不实用
非常实用
1
2
3
4
5
6
7
8
9
10
您认为此功能如何分析更能满足您的需求,请填写您的反馈:
相似国自然基金
{{ item.name }}
- 批准号:{{ item.ratify_no }}
- 批准年份:{{ item.approval_year }}
- 资助金额:{{ item.support_num }}
- 项目类别:{{ item.project_type }}
相似海外基金
{{
item.name }}
{{ item.translate_name }}
- 批准号:{{ item.ratify_no }}
- 财政年份:{{ item.approval_year }}
- 资助金额:{{ item.support_num }}
- 项目类别:{{ item.project_type }}