OPA1缺失介导的线粒体自噬水平降低促发慢性心力衰竭及机制
项目介绍
AI项目解读
基本信息
- 批准号:81700342
- 项目类别:青年科学基金项目
- 资助金额:20.0万
- 负责人:
- 依托单位:
- 学科分类:H0209.心力衰竭
- 结题年份:2020
- 批准年份:2017
- 项目状态:已结题
- 起止时间:2018-01-01 至2020-12-31
- 项目参与者:张星; 杨璐; 廉坤; 王臻; 杨红燕; 侯作旭; 徐杰; 李国华;
- 关键词:
项目摘要
Mortality and morbidity in patients with chronic heart failure (HF) are increasing each year and survival estimates only 50% at 5 years after the diagnosis of HF. So, HF is one of the major causes of human death and disability. Mitochondrial defects reduce the amount of cardiomyocytes energy and relate to many cardiac disorders, which exist in the beginning of hypertrophy and HF. Alteration of mitochondrial function is considered an origin of HF, as well as a target. However, what causes mitochondrial dysfunction in the development of HF has not been fully understood. OPA1 is a dynamic-related protein and mediates the mitochondrial fusion, maintains mitochondrial cristae morphology and prevents mitochondria-related apoptosis. In our latest preliminary study, mice with transverse aortic constriction-induced HF showed decreased mitophagy and OPA1 protein level. On the other hand, deficiency of OPA1 in cardiac-specific OPA1 knockout mice caused mitochondrial dysfunction and HF, meanwhile with lower level of mitophagy. These data suggest that mitophagy possibly plays a role in the development of HF and OPA1 may regulate mitophagy. However, the mechanisms remain largely unknown. Based on these data, we hypothesized that downregulation of mitophagy induced by OPA1 deficiency in cardiomyocytes contributes to development of HF. The present study was designed to clarify this hypothesis and investigate the underlying mechanisms. Proving this hypothesis might gain further insight into the pathogenesis of HF and provide theoretical basis and novel possible targets for preventing mitochondrial dysfunction in HF.
心力衰竭是严重威胁人类健康和寿命的重大慢病,近年国人心衰患病率持续上升,亟需阐明其分子病因学机制以探寻新的防控措施。线粒体自噬是线粒体质控途径的关键环节,然而其在心衰中的变化、作用及上游机制尚未阐明。OPA1是核编码的线粒体蛋白,可介导线粒体融合、防止线粒体途径引发的凋亡等。申请者新近预实验发现,压力负荷性心衰小鼠心肌线粒体自噬水平降低、OPA1蛋白下调,更重要的是,心肌特异性OPA1敲除小鼠线粒体结构功能异常、线粒体自噬水平降低并出现心力衰竭。提示,线粒体自噬水平与心衰进程存在密切联系;OPA1参与线粒体自噬的调控,但其与心衰的关系及机制尚不清楚。本课题拟在前期发现基础上,阐明OPA1下调介导的线粒体自噬水平降低可促发慢性心衰、并进一步研究其机制,期望为深入认识心衰发生的线粒体机理提供实验依据,并为防控心衰提供分子靶点。
结项摘要
心力衰竭(心衰)是冠心病、高血压、糖尿病心肌病等多种心血管疾病的终末阶段,线粒体缺陷可致代谢异常、心肌能量匮乏以及氧化应激增强等,参与心脏的多种病理过程,因此,改善线粒体功能、提高心肌能量供应成为早期防控心血管疾病的可能途径之一。在本项目资助下,我们在国际上首次发现:①压力负荷性心衰小鼠心肌线粒体自噬水平下降,OPA1表达降低;心肌特异性OPA1缺失可诱发小鼠线粒体结构和功能障碍,线粒体自噬水平降低及心力衰竭。OPA1敲除小鼠心肌组织mTOR及下游S6、ULK1磷酸化水平均升高,雷帕霉素腹腔注射可阻断TOR及其下游S6、ULK1磷酸化水平,同时改善OPA1缺失导致的线粒体自噬水平降低、线粒体功能、心肌肥厚和心脏功能下降,OPA1可与ULK1和FUNDC1形成复合体调控线粒体自噬过程,提示其改变是线粒体自噬影响心衰发生发展的关键机制。② 胎球蛋白B(FetB)上调导致胰岛素受体β酪氨酸磷酸化水平降低及其下游信号激活减少,致胰岛素诱导的代谢效应受损和糖尿病缺血心肌损伤加重。③ 长期热水浴可改善慢性应激大鼠心肌自噬紊乱、促进线粒体结构恢复、减轻慢性应激所致的心肌损伤。这些发现不仅对认识心衰发生发展的线粒体相关机理有理论意义,而且对探索新的防治措施有现实意义。
项目成果
期刊论文数量(5)
专著数量(1)
科研奖励数量(4)
会议论文数量(0)
专利数量(1)
热水浴对慢性应激心肌损伤的防护作用
- DOI:--
- 发表时间:2019
- 期刊:心脏杂志
- 影响因子:--
- 作者:田沛;徐莉;王化宁;王鹏莉;杨红燕;胡文东;邢文娟;高峰
- 通讯作者:高峰
内质网-线粒体交互在心血管疾病中的作用研究进展
- DOI:--
- 发表时间:2020
- 期刊:心脏杂志
- 影响因子:--
- 作者:张滋彬;贾敏;王敏;张鹏飞;张海锋;高峰;邢文娟
- 通讯作者:邢文娟
Tribbles Homolog 3-Mediated Vascular Insulin Resistance Contributes to Hypoxic Pulmonary Hypertension in Intermittent Hypoxia Rat Model.
Tribbles 同源物 3 介导的血管胰岛素抵抗导致间歇性缺氧大鼠模型中的缺氧性肺动脉高压
- DOI:10.3389/fphys.2020.542146
- 发表时间:2020
- 期刊:Frontiers in physiology
- 影响因子:4
- 作者:Fan F;He J;Su H;Zhang H;Wang H;Dong Q;Zeng M;Xing W;Sun X
- 通讯作者:Sun X
Tetrahydroxystilbene Glycoside Improves Microvascular Endothelial Dysfunction and Ameliorates Obesity-Associated Hypertension in Obese ZDF Rats Via Inhibition of Endothelial Autophagy
四羟基二苯乙烯苷通过抑制内皮自噬改善肥胖 ZDF 大鼠的微血管内皮功能障碍并改善肥胖相关高血压
- DOI:10.1159/000480410
- 发表时间:2017-01-01
- 期刊:CELLULAR PHYSIOLOGY AND BIOCHEMISTRY
- 影响因子:--
- 作者:Dong, Qianqian;Xing, Wenjuan;Zhang, Haifeng
- 通讯作者:Zhang, Haifeng
数据更新时间:{{ journalArticles.updateTime }}
{{
item.title }}
{{ item.translation_title }}
- DOI:{{ item.doi || "--"}}
- 发表时间:{{ item.publish_year || "--" }}
- 期刊:{{ item.journal_name }}
- 影响因子:{{ item.factor || "--"}}
- 作者:{{ item.authors }}
- 通讯作者:{{ item.author }}
数据更新时间:{{ journalArticles.updateTime }}
{{ item.title }}
- 作者:{{ item.authors }}
数据更新时间:{{ monograph.updateTime }}
{{ item.title }}
- 作者:{{ item.authors }}
数据更新时间:{{ sciAawards.updateTime }}
{{ item.title }}
- 作者:{{ item.authors }}
数据更新时间:{{ conferencePapers.updateTime }}
{{ item.title }}
- 作者:{{ item.authors }}
数据更新时间:{{ patent.updateTime }}
其他文献
长期胰岛素治疗通过促进BNP表达延缓缺血性心力衰竭的发展及其机制
- DOI:--
- 发表时间:2013
- 期刊:中国病理生理杂志
- 影响因子:--
- 作者:于立明;闫文俊;邢文娟;乔红玉;张薇;朱妙章;高峰;于军;张海锋
- 通讯作者:张海锋
胰岛素与心血管健康——纪念胰岛素发现100周年
- DOI:10.1360/ssv-2021-0351
- 发表时间:2021
- 期刊:中国科学. 生命科学
- 影响因子:--
- 作者:邢文娟;张星;李嘉;董玲;张海锋;高峰
- 通讯作者:高峰
航天脑科学研究进展
- DOI:10.13376/j.cbls/2022082
- 发表时间:2022
- 期刊:生命科学
- 影响因子:--
- 作者:范媛媛;厉建伟;邢文娟;凌树宽;李英贤
- 通讯作者:李英贤
脂肪因子CTRP9对低氧性肺动脉高压大鼠肺血管的舒张作用及其机制。
- DOI:--
- 发表时间:2016
- 期刊:心脏杂志
- 影响因子:--
- 作者:杨瑞;张存娟;邢文娟;梁向艳;苏慧;张海锋;孙 新
- 通讯作者:孙 新
脂联素改善间歇性低氧性肺动脉高压大鼠离体肺动脉舒张功能及其机制
- DOI:--
- 发表时间:2016
- 期刊:心脏杂志
- 影响因子:--
- 作者:张存娟;杨瑞;邢文娟;梁向艳;苏慧;张海锋;孙 新
- 通讯作者:孙 新
其他文献
{{
item.title }}
{{ item.translation_title }}
- DOI:{{ item.doi || "--" }}
- 发表时间:{{ item.publish_year || "--"}}
- 期刊:{{ item.journal_name }}
- 影响因子:{{ item.factor || "--" }}
- 作者:{{ item.authors }}
- 通讯作者:{{ item.author }}
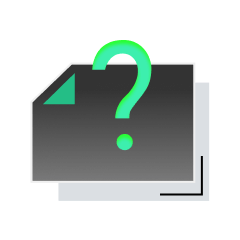
内容获取失败,请点击重试
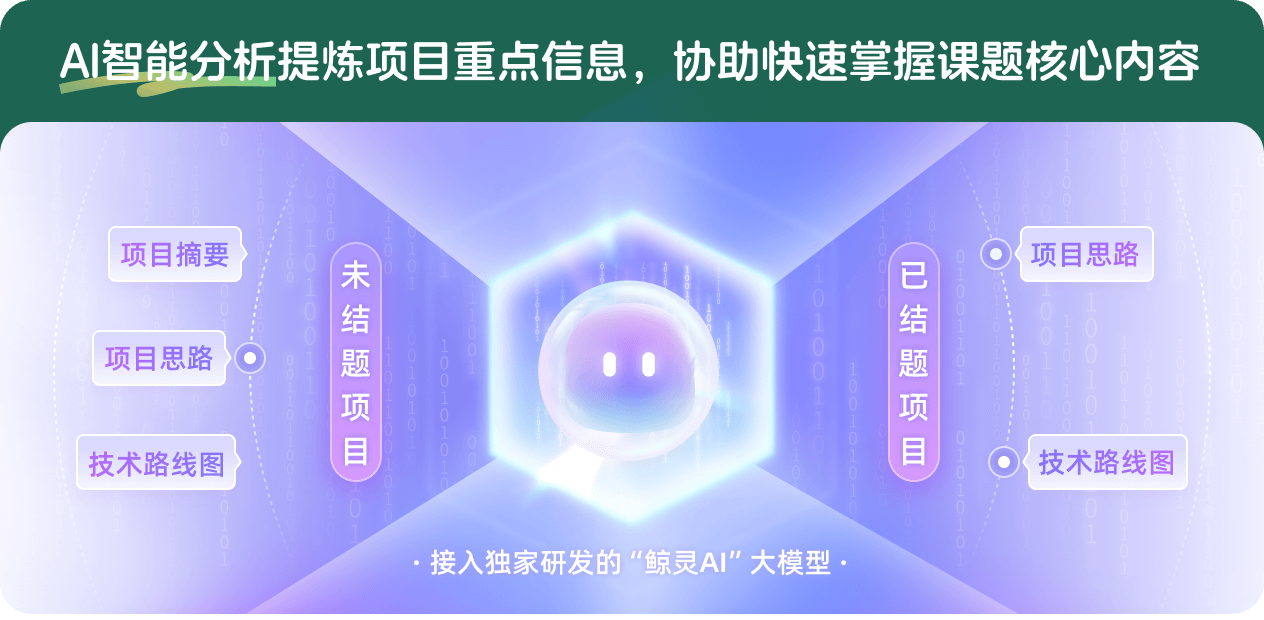
查看分析示例
此项目为已结题,我已根据课题信息分析并撰写以下内容,帮您拓宽课题思路:
AI项目摘要
AI项目思路
AI技术路线图
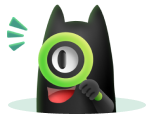
请为本次AI项目解读的内容对您的实用性打分
非常不实用
非常实用
1
2
3
4
5
6
7
8
9
10
您认为此功能如何分析更能满足您的需求,请填写您的反馈:
邢文娟的其他基金
HADHA乳酸化修饰调控代谢稳态在模拟失重心脏储备功能失调中的作用及机制
- 批准号:32371239
- 批准年份:2023
- 资助金额:50 万元
- 项目类别:面上项目
胰岛素受体核转位异常促糖尿病心肌缺血易损性增加及机制
- 批准号:
- 批准年份:2020
- 资助金额:58 万元
- 项目类别:面上项目
相似国自然基金
{{ item.name }}
- 批准号:{{ item.ratify_no }}
- 批准年份:{{ item.approval_year }}
- 资助金额:{{ item.support_num }}
- 项目类别:{{ item.project_type }}
相似海外基金
{{
item.name }}
{{ item.translate_name }}
- 批准号:{{ item.ratify_no }}
- 财政年份:{{ item.approval_year }}
- 资助金额:{{ item.support_num }}
- 项目类别:{{ item.project_type }}