基于跨层次生成对抗网络的移动端图像超分辨率研究
项目介绍
AI项目解读
基本信息
- 批准号:61871284
- 项目类别:面上项目
- 资助金额:63.0万
- 负责人:
- 依托单位:
- 学科分类:F0116.图像信息处理
- 结题年份:2022
- 批准年份:2018
- 项目状态:已结题
- 起止时间:2019-01-01 至2022-12-31
- 项目参与者:李源; 王瑞硕; 李炜; 杨帆; 马璁; 陶砺;
- 关键词:
项目摘要
Generative adversarial networks (GANs) are a class of generative models for learning probability distributions of data by formulating a minimax game between a generator and a discriminator. It has been applied in image super-resolution and achieved the progressive results. However, when enlarging the compressed images on mobile terminals, this model cannot fast and stably generate high-resolution textures and details. The reasons include the diversity loss of generated features, the imbalance for optimizing the generator and the discriminator, the inaccurate objective functions and the instability of training performance. To solve these problems, this project focuses on how to design and train GANs for producing the high-quality super-resolution reconstruction. We propose some approaches to improve the generation performance, which include the dense layer connection of cross-level features, the combination with multi-scale shearlet transform, the improved loss functions. These methods are expected to obtain the accurate mapping of feature spaces at different resolution levels, and generate a high-resolution image with natural and reliable textures. In order to explore the ways to enhance the training stability and accelerate the convergence rate, this project adopts the subgradient method and the weight normalization technique to perform the training optimization. Moreover, only the principal weights are normalized according to the spectral properties of a high-dimensional weight space, which are deduced by using random matrix theory. The proposed super-resolution algorithms would be integrated and tested on the terminal devices. This study has important sense to clarify the adversarial mechanism of learning process and reveal the generation rule of image super-resolution.
生成对抗网络是一种通过内部对抗机制进行学习的概率生成模型,在图像超分辨率领域已取得显著进展。但对移动端压缩图像放大时,还不能快速稳定生成高分辨率的纹理细节。这是由于当前研究还未解决该类模型存在的生成特征多样性不足与失真、生成和判别网络优化过程的不同步平衡、目标函数表达不准确以及训练性能不稳定的问题。为解决这些关键问题,本项目研究如何设计与训练对抗生成网络才能实现高质量超分辨率生成,提出对网络的高密集跨特征层次连接,并与多尺度Shearlet变换结合的建模方法,设计合理的目标函数,可望得到不同分辨率特征之间的准确映射关系,生成失真少纹理丰富的高分辨率图像。本项目在训练时使用次梯度优化方法,利用随机矩阵理论分析高维权重空间的谱性质,确定主成分权重并进行归一化处理,探索提高训练稳定性和收敛速度的有效途径。本研究算法在移动端设备进行原型验证,对阐明对抗学习机制,揭示图像超分辨率生成规律有重要意义。
结项摘要
本项目重点针对移动端压缩图像的超分辨率生成任务,深入研究了生成对抗网络的设计、训练、优化与实际部署。为了快速稳定得到高质量的图像超分辨率重建结果,本项目对生成对抗网络的结构设计与建模方法进行研究,提出了基于注意力机制的多尺度网络模型,结合小波变换的特征动态融合与调制模型,以及基于高阶语义关系的模型,这些模型可以学习不同分辨率特征之间的准确映射关系,输出高保真的超分辨率结果。在对训练过程研究时,提出了基于预训练模型的有效目标函数,设计了精确的概率分布距离测度,增强了超分辨率图像的视觉质量,改进了判别器的结构及训练方式,使用一致优化理论同步更新梯度,有效提高了训练的稳定性和收敛速度。优化网络模型时,利用随机矩阵理论分析高维权重的谱性质,确定主成分权重并进行归一化处理,加速收敛并提升了模型的泛化能力。对潜在空间进行正交分解,实现可控的语义解耦,完成图像超分辨率生成系统的移动端快速轻量化部署。本项目阐明了对抗学习机制,突破了图像超分辨率技术在移动端部署的限制,对全面提升生成对抗网络的设计、训练与优化的理论与应用水平具有重要的科学意义。
项目成果
期刊论文数量(8)
专著数量(0)
科研奖励数量(0)
会议论文数量(3)
专利数量(9)
BASNet: A Boundary-Aware Siamese Network for Accurate Remote-Sensing Change Detection
BASNet:用于精确遥感变化检测的边界感知连体网络
- DOI:10.1109/lgrs.2021.3119885
- 发表时间:2022
- 期刊:IEEE Geoscience and Remote Sensing Letters
- 影响因子:4.8
- 作者:Hao Wei;Rui Chen;Chang Yu;Hang Yang;Shipeng An
- 通讯作者:Shipeng An
Point cloud denoising using non-local collaborative projections
使用非局部协作投影进行点云去噪
- DOI:10.1016/j.patcog.2021.108128
- 发表时间:2021-07-03
- 期刊:PATTERN RECOGNITION
- 影响因子:8
- 作者:Zhou, Yiyao;Chen, Rui;Zhou, Guoqing
- 通讯作者:Zhou, Guoqing
基于特征融合的深度学习点云补全算法
- DOI:10.11784/tdxbz202103073
- 发表时间:2022
- 期刊:天津大学学报. 自然科学与工程技术版
- 影响因子:--
- 作者:赵毅强;周意遥;胡彬;艾西丁;陈瑞
- 通讯作者:陈瑞
Learning Dynamic Generative Attention for Single Image Super-Resolution
学习单图像超分辨率的动态生成注意力
- DOI:10.1109/tcsvt.2022.3192099
- 发表时间:2022-12-01
- 期刊:IEEE TRANSACTIONS ON CIRCUITS AND SYSTEMS FOR VIDEO TECHNOLOGY
- 影响因子:8.4
- 作者:Chen, Rui;Zhang, Yan
- 通讯作者:Zhang, Yan
融合参考先验与生成先验的老照片修复
- DOI:10.11834/jig.210562
- 发表时间:2022
- 期刊:中国图象图形学报
- 影响因子:--
- 作者:刘继鑫;陈瑞;安仕鹏
- 通讯作者:安仕鹏
数据更新时间:{{ journalArticles.updateTime }}
{{
item.title }}
{{ item.translation_title }}
- DOI:{{ item.doi || "--"}}
- 发表时间:{{ item.publish_year || "--" }}
- 期刊:{{ item.journal_name }}
- 影响因子:{{ item.factor || "--"}}
- 作者:{{ item.authors }}
- 通讯作者:{{ item.author }}
数据更新时间:{{ journalArticles.updateTime }}
{{ item.title }}
- 作者:{{ item.authors }}
数据更新时间:{{ monograph.updateTime }}
{{ item.title }}
- 作者:{{ item.authors }}
数据更新时间:{{ sciAawards.updateTime }}
{{ item.title }}
- 作者:{{ item.authors }}
数据更新时间:{{ conferencePapers.updateTime }}
{{ item.title }}
- 作者:{{ item.authors }}
数据更新时间:{{ patent.updateTime }}
其他文献
地方政府债务与房价的作用机制分析
- DOI:10.16797/j.cnki.11-5224/c.20180113.001
- 发表时间:2018
- 期刊:北京交通大学学报(社会科学版)
- 影响因子:--
- 作者:陈瑞;葛成唯;傅强;黄映红
- 通讯作者:黄映红
肠道益生菌制剂干预胰岛素抵抗效应的Meta分析
- DOI:10.13193/j.issn.1673-7717.2019.07.008
- 发表时间:2019
- 期刊:中华中医药学刊
- 影响因子:--
- 作者:宋爱群;陈瑞;王文炎;梁凤霞
- 通讯作者:梁凤霞
针灸防治糖尿病前期的临床研究思路探讨
- DOI:--
- 发表时间:2018
- 期刊:中华中医药杂志
- 影响因子:--
- 作者:王文炎;梁凤霞;宋爱群;陈瑞;吴松;李佳;王华
- 通讯作者:王华
浅谈对“心主神明”的认识
- DOI:10.16367/j.issn.1003-5028.2020.08.0292
- 发表时间:2020
- 期刊:河南中医
- 影响因子:--
- 作者:王文炎;陈瑞;梁凤霞
- 通讯作者:梁凤霞
基于多肽的纳米药物递送系统的研究
- DOI:--
- 发表时间:2018
- 期刊:化学进展
- 影响因子:--
- 作者:徐柳;钱晨;朱辰奇;陈志鹏;陈瑞
- 通讯作者:陈瑞
其他文献
{{
item.title }}
{{ item.translation_title }}
- DOI:{{ item.doi || "--" }}
- 发表时间:{{ item.publish_year || "--"}}
- 期刊:{{ item.journal_name }}
- 影响因子:{{ item.factor || "--" }}
- 作者:{{ item.authors }}
- 通讯作者:{{ item.author }}
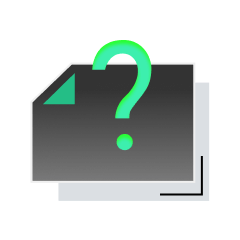
内容获取失败,请点击重试
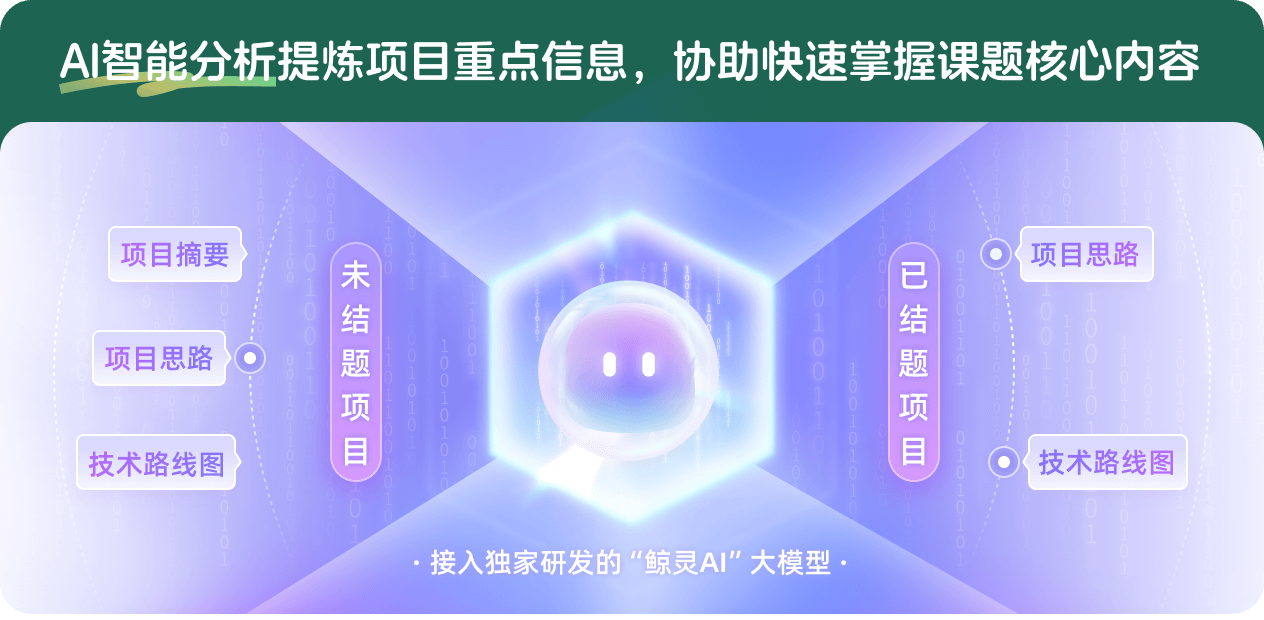
查看分析示例
此项目为已结题,我已根据课题信息分析并撰写以下内容,帮您拓宽课题思路:
AI项目摘要
AI项目思路
AI技术路线图
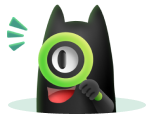
请为本次AI项目解读的内容对您的实用性打分
非常不实用
非常实用
1
2
3
4
5
6
7
8
9
10
您认为此功能如何分析更能满足您的需求,请填写您的反馈:
陈瑞的其他基金
基于几何空间嵌入约束的可控图像生成方法研究
- 批准号:62272341
- 批准年份:2022
- 资助金额:54.00 万元
- 项目类别:面上项目
基于几何空间嵌入约束的可控图像生成方法研究
- 批准号:
- 批准年份:2022
- 资助金额:54 万元
- 项目类别:面上项目
相似国自然基金
{{ item.name }}
- 批准号:{{ item.ratify_no }}
- 批准年份:{{ item.approval_year }}
- 资助金额:{{ item.support_num }}
- 项目类别:{{ item.project_type }}
相似海外基金
{{
item.name }}
{{ item.translate_name }}
- 批准号:{{ item.ratify_no }}
- 财政年份:{{ item.approval_year }}
- 资助金额:{{ item.support_num }}
- 项目类别:{{ item.project_type }}