基于空间相关特性的大幅宽SAR图像舰船检测方法研究
项目介绍
AI项目解读
基本信息
- 批准号:61701157
- 项目类别:青年科学基金项目
- 资助金额:25.0万
- 负责人:
- 依托单位:
- 学科分类:F0113.信息获取与处理
- 结题年份:2020
- 批准年份:2017
- 项目状态:已结题
- 起止时间:2018-01-01 至2020-12-31
- 项目参与者:贾璐; 杨昭; 汪骏; 石聪聪; 胡志勇; 张皖南;
- 关键词:
项目摘要
Ship detection has been a hot topic in the fields of synthetic aperture radar (SAR) image application. Due to the limited observation time of the SAR satellite, on the one hand, we expect that SAR imaging has a wide swath to improve the observation time efficiency; on the other hand, we expect that SAR imaging has a high resolution to improve the detection accuracy. However, the resolution and the observation swath are always the main contradiction of SAR imaging, which restricts its effective application. To solve this problem, this project uses wide swath SAR image as the data source, and aims to realize high-precision ship detection. The spatial correlation characteristics of ship targets in SAR images is analyzed in-depth. A new feature named “spatial correlation constraint relationship” is constructed, and it is integrated into the whole process of ship detection. Based on the newly constructed feature, a spatial correlation tree model is constructed to fuse the context information of the extracted feature points, multi-layer and co-ordinate target representation is realized. A ship detection framework based on the spatial correlation tree model matching is built, model learning and its corresponding classification methods are optimized to realize high-precision ship detection. This research is expected to promote the application level of wide swath SAR image in the field of ship detection, which has important theoretical and practical value.
舰船检测一直是合成孔径雷达(SAR)图像应用领域的研究热点。由于SAR卫星过顶观测时间有限,一方面期望SAR成像具有较大的观测带宽,提升舰船检测的时效性;另一方面期望SAR成像具有较高的分辨率,提高舰船检测的准确性。然而分辨率与观测带宽始终是困扰SAR成像的主要矛盾,制约着其有效应用。针对这一问题,本项目以大幅宽SAR图像为数据源,以高精度舰船检测为研究目标,深入分析SAR图像舰船目标的空间相关特性,构建空间相关约束关系这一新特征,并将该特征融合到舰船检测的全过程;基于目标特征点之间的空间相关约束关系,构建一种空间相关树模型,深度融合特征点之间的上下文信息,实现目标特征的多层级有序表达;搭建基于空间相关树模型匹配的舰船检测框架,优化相应的模型学习与分类方法,实现舰船目标的高精度检测。本项目的开展有望提升大幅宽SAR图像舰船检测的水平,具有重要的理论意义和应用价值。
结项摘要
本项目针对SAR图像舰船检测中存在的准确性与时效性之间的矛盾,以中等分辨率大幅宽SAR图像为数据源,在深入分析舰船目标特性的基础上,建立了目标像素点之间、目标特征点之间的空间相关约束关系这一新特征,并将该特征融合到舰船检测的全过程,实现了大幅宽SAR图像舰船目标的高精度检测。.在本项目支持下,取得了如下几方面的重要成果:.(1) 构建了复杂环境下的大幅宽星载SAR图像舰船目标样本库,样本库数量为8400,构建了SAR图像目标分类样本库,包含舰船、飞机、坦克、岛屿及油罐5类目标,样本数量为7500。.(2) 构建了目标像素点之间、目标特征点之间的空间相关约束关系模型,并将该模型应用到舰船目标CFAR初筛与目标鉴别中, 搭建了基于空间相关特性的大幅宽SAR图像舰船检测与实验验证方法体系,并针对复杂环境海域开展了实验验证。.(3) 研制了一套基于空间相关特性的大幅宽SAR图像舰船检测系统,并针对我国上海港、青岛港等附近复杂环境海域开展了大量的舰船检测实验,设计了相应的检测性能评估参数,对舰船检测性能进行评估。.(4) 发表了高水平论文与申报发明专利:在IEEE TGRS、IEEE TAES、IEEE TITS、IEEE Access、IEEE JOE、IJRS、遥感学报、雷达学报、IGARSS 2018等国内外知名期刊及会议上发表或录用论文20余篇。其中:SCI论文11篇,JCR 1区论文8篇。申报国家发明专利6项、软件著作权3项。.(5) 培养优秀人才并完善创新团队:在项目支持下,联合培养了SAR图像解译方向的博士生2名、硕士生6名,完善了“高分系列卫星协同目标探测与应用”创新人才团队。.(6) 积极推动成果转化:与自然资源部第一海洋研究所、中科院电子所、中电38所等开展项目合作,将本项目研制过程中提出的目标检测识别新理论、新模型进行成果转化,积极推动我国SAR目标检测识别等领域的产业化发展。.(7) 积极参加国际学术交流,参与国内外国际会议与研讨会交流3次。.(8) 开展成果展示,通过遥感学报、雷达学报等微信公众号及网站开展学术成果展示与推广。. 本项目研究提升了复杂环境下SAR舰船目标的检测性能,提高了目标检测率、同时降低了虚警率。研究成果具有较高的理论研究意义与良好的军事与民事应用价值,可有力推动我国SAR卫星在海洋目标检测方面的应用。
项目成果
期刊论文数量(15)
专著数量(0)
科研奖励数量(6)
会议论文数量(2)
专利数量(7)
A Refined Bilateral Filtering Algorithm Based on Adaptively-Trimmed-Statistics for Speckle Reduction in SAR Imagery
基于自适应修整统计的改进SAR图像散斑抑制双边滤波算法
- DOI:10.1109/access.2019.2931572
- 发表时间:2019
- 期刊:IEEE Access
- 影响因子:3.9
- 作者:Ai Jiaqiu;Liu Ruiming;Tang Bo;Jia Lu;Zhao Jinling;Zhou Fang
- 通讯作者:Zhou Fang
Adaptively truncated clutter-statistics-based variability index constant false-alarm-rate detector in SAR imagery
SAR 图像中基于自适应截断杂波统计的变异性指数常数虚警率检测器
- DOI:10.1117/1.jrs.14.026509
- 发表时间:2020
- 期刊:Journal of Applied Remote Sensing
- 影响因子:1.7
- 作者:Yang Hang;Ai Jiaqiu;Shi Weidong;Zhou Fang;Zhao Jinling;Niu Zhao
- 通讯作者:Niu Zhao
An adaptive-trimming-depth based CFAR detector of heterogeneous environment in SAR imagery
基于自适应修整深度的SAR图像异构环境CFAR检测器
- DOI:10.1080/2150704x.2020.1763501
- 发表时间:2020
- 期刊:Remote Sensing Letters
- 影响因子:2.3
- 作者:Ai Jiaqiu;Cao Zhenxiang;Xing Mengdao
- 通讯作者:Xing Mengdao
Surface Defect Classification for Hot-Rolled Steel Strips by Selectively Dominant Local Binary Patterns
通过选择性主导局部二元模式对热轧钢带进行表面缺陷分类
- DOI:10.1109/access.2019.2898215
- 发表时间:2019
- 期刊:IEEE Access
- 影响因子:3.9
- 作者:Luo Qiwu;Fang Xiaoxin;Sun Yichuang;Li Li;Ai Jiaqiu;Yang Chunhua;Simpson Oluyomi
- 通讯作者:Simpson Oluyomi
Truncated-statistics-based bilateral filter for speckle reduction in synthetic aperture radar imagery
用于合成孔径雷达图像中散斑减少的基于截断统计的双边滤波器
- DOI:10.1117/1.jrs.13.026505
- 发表时间:2019
- 期刊:Journal of Applied Remote Sensing
- 影响因子:1.7
- 作者:Ai Jiaqiu;Yang Hang;Yang Xuezhi;Liu Ruiming;Luo Qiwu;Zhang Xiaohui
- 通讯作者:Zhang Xiaohui
数据更新时间:{{ journalArticles.updateTime }}
{{
item.title }}
{{ item.translation_title }}
- DOI:{{ item.doi || "--"}}
- 发表时间:{{ item.publish_year || "--" }}
- 期刊:{{ item.journal_name }}
- 影响因子:{{ item.factor || "--"}}
- 作者:{{ item.authors }}
- 通讯作者:{{ item.author }}
数据更新时间:{{ journalArticles.updateTime }}
{{ item.title }}
- 作者:{{ item.authors }}
数据更新时间:{{ monograph.updateTime }}
{{ item.title }}
- 作者:{{ item.authors }}
数据更新时间:{{ sciAawards.updateTime }}
{{ item.title }}
- 作者:{{ item.authors }}
数据更新时间:{{ conferencePapers.updateTime }}
{{ item.title }}
- 作者:{{ item.authors }}
数据更新时间:{{ patent.updateTime }}
其他文献
A New CFAR Ship Detection Algorithm Based on 2-D Joint Log-Normal Distribution in SAR Images
一种基于SAR图像二维联合对数正态分布的恒虚警船舶检测新算法
- DOI:--
- 发表时间:2010-10-01
- 期刊:
- 影响因子:--
- 作者:艾加秋;齐向阳;禹卫东
- 通讯作者:禹卫东
安徽省安庆市沿江沼泽湿地恢复空间分析
- DOI:10.13961/j.cnki.stbctb.2017.06.030
- 发表时间:2017
- 期刊:水土保持通报
- 影响因子:--
- 作者:董张玉;杨学志;王宗明;周芳;艾加秋;贾璐
- 通讯作者:贾璐
其他文献
{{
item.title }}
{{ item.translation_title }}
- DOI:{{ item.doi || "--" }}
- 发表时间:{{ item.publish_year || "--"}}
- 期刊:{{ item.journal_name }}
- 影响因子:{{ item.factor || "--" }}
- 作者:{{ item.authors }}
- 通讯作者:{{ item.author }}
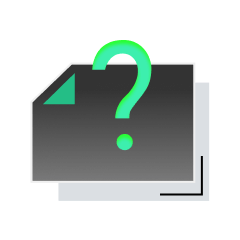
内容获取失败,请点击重试
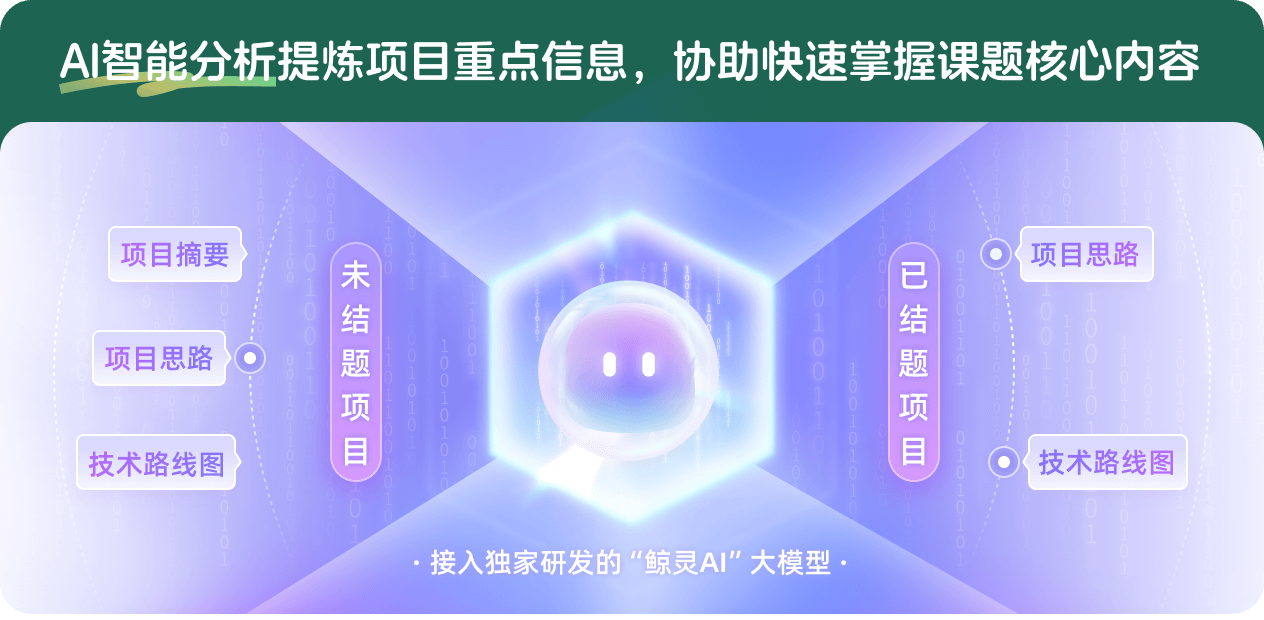
查看分析示例
此项目为已结题,我已根据课题信息分析并撰写以下内容,帮您拓宽课题思路:
AI项目摘要
AI项目思路
AI技术路线图
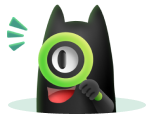
请为本次AI项目解读的内容对您的实用性打分
非常不实用
非常实用
1
2
3
4
5
6
7
8
9
10
您认为此功能如何分析更能满足您的需求,请填写您的反馈:
相似国自然基金
{{ item.name }}
- 批准号:{{ item.ratify_no }}
- 批准年份:{{ item.approval_year }}
- 资助金额:{{ item.support_num }}
- 项目类别:{{ item.project_type }}
相似海外基金
{{
item.name }}
{{ item.translate_name }}
- 批准号:{{ item.ratify_no }}
- 财政年份:{{ item.approval_year }}
- 资助金额:{{ item.support_num }}
- 项目类别:{{ item.project_type }}