基于深度内容基元学习的遥感影像表征与重建方法研究
项目介绍
AI项目解读
基本信息
- 批准号:61801260
- 项目类别:青年科学基金项目
- 资助金额:26.0万
- 负责人:
- 依托单位:
- 学科分类:F0113.信息获取与处理
- 结题年份:2021
- 批准年份:2018
- 项目状态:已结题
- 起止时间:2019-01-01 至2021-12-31
- 项目参与者:韩超诣; 王隽; 黄丹蓝; 林柏洪; 张子卓; 陈昭;
- 关键词:
项目摘要
With the steady improvement of temporal and spatial resolution, the remote-sensing image data size is increasing explosively. This results in the bottleneck between the big data of the remote sensing images and the limited transmission capacity. Intelligent processing of massive remote sensing images in orbit is one of the important ways to break the bottleneck of transmission bandwidth. The source of intelligent processing is the representation of the remote sensing images. The traditional representation methods are restricted by Shannon information theory and is inefficient. In this project, from the essential characteristics of human visual perception, we can mine the content pattern of the remote sensing images, and then study the efficient representation and reconstruction key technologies based on the content pattern and visual attention model. In fact, we use the intelligent computing to reduce the pressure of the transmit bandwidth. The specific research contents include: (1) remote sensing image content dictionary optimization and learning method based on sparse cognition; (2) remote sensing image deep representation model based on content dictionary; (3) Content-of-interest remote sensing reconstruction model based on attentive generative adversarial network. The above technologies will be tested on the real optical, SAR remote sensing images and so on. This project will provide new theory and technical support for the intelligent processing in orbit.
随着遥感影像时空分辨率的稳步提升,其数据规模呈几何级数增长,遥感影像大数据与有限传输能力之间的矛盾日益突出。海量遥感影像星上智能化处理是突破传输带宽瓶颈的重要途径之一,其分析与处理的源头是信息表征。传统方法受香农信息论的制约,表征效率低下。本项目面向高分辨率遥感影像数据,从人类视觉感知的本质特征入手,挖掘遥感影像的内容模式,研究基于内容基元与视觉注意的高效表征与重建关键技术,以智能计算换取带宽,从根本上降低遥感大数据对传输带宽需求的压力。具体研究内容包括:(1)基于稀疏认知的遥感影像内容字典学习与优化方法;(2)基于内容字典的遥感影像深度表征模型;(3)基于视觉注意生成对抗网络的围绕感兴趣内容的遥感影像重建。将上述技术在光学遥感影像、SAR遥感影像等实测数据上进行验证,为遥感大数据星上智能化处理提供新的理论依据与技术支持。
结项摘要
该项目从人类视觉感知的本质特征入手,挖掘遥感影像的内容模式,研究基于内容基元与视觉注意机制的高效表征与重建关键技术,在内容字典构建、时空结构表征以及生成式重建方面取得了一定的理论和技术突破,从根本上降低遥感大数据对传输带宽需求的压力,达到了预期的研究目标。取得的主要创新成果有:.(1)针对特定变换基下图像的结构化特性难以描述的问题,提出基于稀疏认知的内容字典学习与优化方法。具体地,将非参数贝叶斯方法引入字典学习,通过稀疏表示训练优化,发掘图像样本的子空间聚类特性,同时自适应地得到与之相匹配的多个结构化内容字典,可大幅提升表征效率。.(2)针对海量遥感数据难以及时下传的难题,提出基于深度内容字典的时空结构模型。通过综合遥感数据初始素描模型、语义分割模型、动态关系生成模型,构建遥感数据新的处理单元:轮廓、语义、关系,使得在低码率下优先保存重要的语义信息,成倍降低多媒体数据量。.(3)针对传统率-失真优化理论在低码率下质量严重退化的难题,提出基于时空素描图的自适应生成式重建方法。基于大规模数据样本构建先验知识库,并结合接收到的时空素描图,建立基于视觉注意机制与生成对抗网络的目标生成模型和图像生成模型,可确保重建遥感数据的语义保真度以及感知质量。.已发表SCI论文10篇,EI论文12篇;申请及授权发明专利16项、软件著作权7项;培养博士研究生4人,硕士研究生2人;入选2019-2021年度中国科协青年人才托举工程;参与举办2020年ICC*CC国际会议;获国际会议最佳学术论文奖2次;参与获得2021年全国发明展览会金奖(2/3)、2019年中国通信学会科学技术发明一等奖(7/15)、2020年上海市技术发明一等奖(11/15)、2019年教育部科技进步一等奖(19/19)等奖项。
项目成果
期刊论文数量(12)
专著数量(0)
科研奖励数量(7)
会议论文数量(12)
专利数量(16)
Dense Convolutional Networks for Semantic Segmentation
用于语义分割的密集卷积网络
- DOI:10.1109/access.2019.2908685
- 发表时间:2019-01-01
- 期刊:IEEE ACCESS
- 影响因子:3.9
- 作者:Han, Chaoyi;Duan, Yiping;Lu, Jianhua
- 通讯作者:Lu, Jianhua
脑启发视频用户体验 评测关键技术
- DOI:--
- 发表时间:2021
- 期刊:中兴通讯技术
- 影响因子:--
- 作者:陶晓明 TAO Xiaoming;杜冰 DU Bing;段一平 DUAN Yiping
- 通讯作者:段一平 DUAN Yiping
Collaborative image compression and classification with multi-task learning for visual internet of things
视觉物联网多任务学习的协作图像压缩和分类
- DOI:10.1016/j.cja.2021.10.003
- 发表时间:2021-10
- 期刊:Chinese Journal of Aeronautics
- 影响因子:5.7
- 作者:Du Bing;Duan Yiping;Zhang Hang;Tao Xiaoming;Wu Yue;Ru CongChong
- 通讯作者:Ru CongChong
Toward Variable-Rate Generative Compression by Reducing the Channel Redundancy
通过减少通道冗余实现可变速率生成压缩
- DOI:10.1109/tcsvt.2020.2965055
- 发表时间:2020-01
- 期刊:IEEE Transactions on on Circuits and Systems for Video Technology
- 影响因子:--
- 作者:Han Chaoyi;Duan Yiping;Tao Xiaoming;Xu Mai;Lu Jianhua
- 通讯作者:Lu Jianhua
Semantic Perceptual Image Compression with a Laplacian Pyramid of Convolutional Networks
使用卷积网络拉普拉斯金字塔进行语义感知图像压缩
- DOI:10.1109/icip.2019.8803784
- 发表时间:2019-09
- 期刊:IEEE Transactions on Image Processing
- 影响因子:10.6
- 作者:Wang Juan;Duan Yiping;Tao Xiaoming;Xu Mai;Lu Jianhua
- 通讯作者:Lu Jianhua
数据更新时间:{{ journalArticles.updateTime }}
{{
item.title }}
{{ item.translation_title }}
- DOI:{{ item.doi || "--"}}
- 发表时间:{{ item.publish_year || "--" }}
- 期刊:{{ item.journal_name }}
- 影响因子:{{ item.factor || "--"}}
- 作者:{{ item.authors }}
- 通讯作者:{{ item.author }}
数据更新时间:{{ journalArticles.updateTime }}
{{ item.title }}
- 作者:{{ item.authors }}
数据更新时间:{{ monograph.updateTime }}
{{ item.title }}
- 作者:{{ item.authors }}
数据更新时间:{{ sciAawards.updateTime }}
{{ item.title }}
- 作者:{{ item.authors }}
数据更新时间:{{ conferencePapers.updateTime }}
{{ item.title }}
- 作者:{{ item.authors }}
数据更新时间:{{ patent.updateTime }}
其他文献
其他文献
{{
item.title }}
{{ item.translation_title }}
- DOI:{{ item.doi || "--" }}
- 发表时间:{{ item.publish_year || "--"}}
- 期刊:{{ item.journal_name }}
- 影响因子:{{ item.factor || "--" }}
- 作者:{{ item.authors }}
- 通讯作者:{{ item.author }}
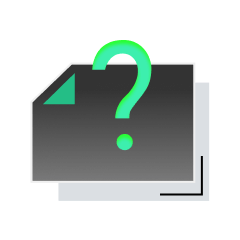
内容获取失败,请点击重试
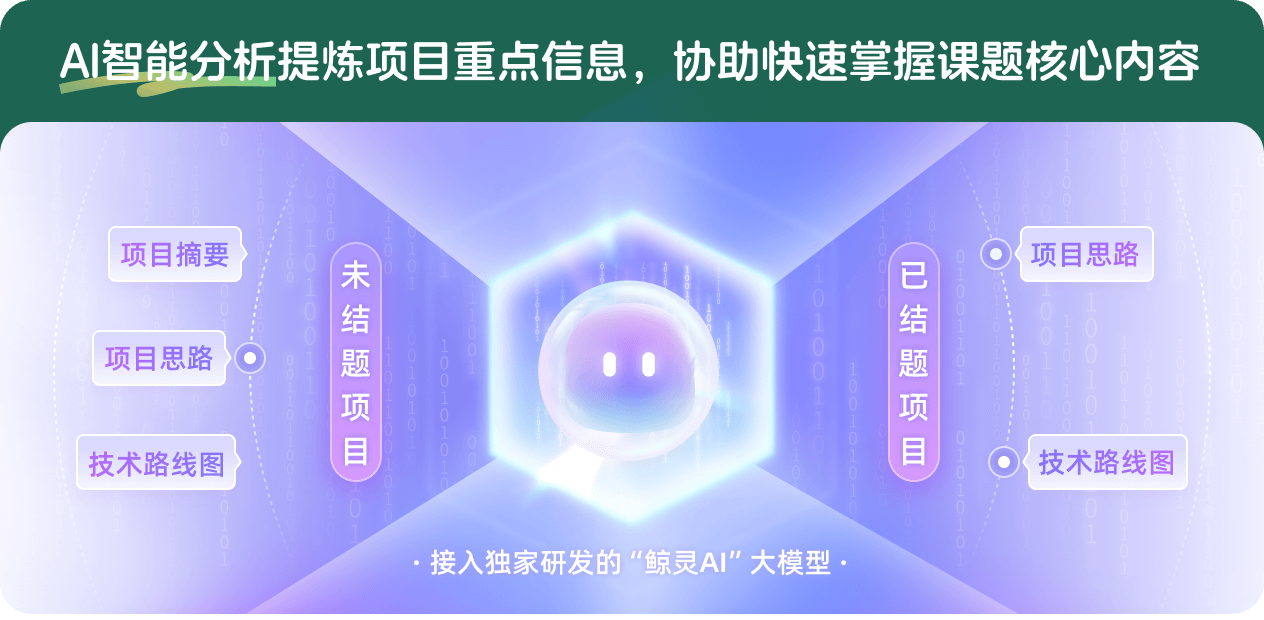
查看分析示例
此项目为已结题,我已根据课题信息分析并撰写以下内容,帮您拓宽课题思路:
AI项目摘要
AI项目思路
AI技术路线图
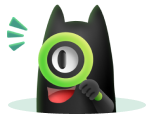
请为本次AI项目解读的内容对您的实用性打分
非常不实用
非常实用
1
2
3
4
5
6
7
8
9
10
您认为此功能如何分析更能满足您的需求,请填写您的反馈:
段一平的其他基金
脑启发视频通信
- 批准号:62171257
- 批准年份:2021
- 资助金额:63 万元
- 项目类别:面上项目
相似国自然基金
{{ item.name }}
- 批准号:{{ item.ratify_no }}
- 批准年份:{{ item.approval_year }}
- 资助金额:{{ item.support_num }}
- 项目类别:{{ item.project_type }}
相似海外基金
{{
item.name }}
{{ item.translate_name }}
- 批准号:{{ item.ratify_no }}
- 财政年份:{{ item.approval_year }}
- 资助金额:{{ item.support_num }}
- 项目类别:{{ item.project_type }}