面向异构计算系统的非对称容错架构
项目介绍
AI项目解读
基本信息
- 批准号:61702328
- 项目类别:青年科学基金项目
- 资助金额:29.0万
- 负责人:
- 依托单位:
- 学科分类:F0204.计算机系统结构与硬件技术
- 结题年份:2020
- 批准年份:2017
- 项目状态:已结题
- 起止时间:2018-01-01 至2020-12-31
- 项目参与者:李超; 王振宁; 王鸿伟; 赵文益; 赵涵;
- 关键词:
项目摘要
We are in the heterogeneous computing era when computing systems harness computational horsepower from not only general purpose CPUs but also other processors such as graphics processing unit (GPU) and hardware accelerators. Performance, power-efficiency, and reliability are three most critical aspects of these heterogeneous processors, and there is a tradeoff amongst them. Accelerators are heavily optimized for performance and power-efficiency, rather than reliability. However, given the CMOS reliability problems that the industry is facing in deep nanometer technology scaling, it is paramount to ensure overall reliability while introducing accelerators into computing systems without increasing additional complexity...The PI will focus on how to architect future computing system for reliability when integrating accelerators into the computing fabric of a system in a scalable and cost-efficient manner. At present, the industry is adopting a “whac-a-mole” design paradigm to achieve accelerator reliability. Each accelerator (e.g., GPU) is designed uniquely to cater for its reliability. The PI believes that this is not a scalable paradigm because it requires a significant amount of engineering effort, and it leads to accelerator-specific reliability optimizations that can hinder the ability to integrate accelerators into different computing fabrics readily...The PI proposes a novel design paradigm called “asymmetric resilience,” whose objective is to ease designers away from developing accelerator-specific reliability optimizations and instead focus on the generic design principles and mechanisms needed to enable a more flexible “plug-n-play” style of architecture to achieve reliability at scale in accelerator-rich heterogeneous systems. A key tenet of asymmetric resilience is to ensure robustness and integrity of the whole system in the event of individual accelerator failures...In asymmetric resiliency, the reliability of an accelerator is centered around the core CPU subsystem. Accelerators adhere to a set of failure semantics that is governed by a hardened, fault-tolerant CPU and a corresponding tightly coupled runtime. In the event of an accelerator failure caused by transient events such as cosmic arrays or Ldi/dt noise, failure semantics and the accelerators resiliency domain (strong or weak domain) dictate how the system reacts. Either the accelerator is revived, or its fault is fully contained. Since accelerators are inherently energy efficient, it costs less to revive the accelerator and replay the previously erroneous computation. Alternatively, since accelerators will be abundant, asymmetric resilience opens up new avenues for reliability optimization, such as commissioning new accelerators and decommissioning fault prone accelerators on-the-fly based on runtime characteristics and power and performance trade-offs.
在如今异构计算时代,传统的CPU和大量加速器一起为主流应用提供性能保障。性能、能效和可靠性是处理器的三大重要指标,而且三者相互制约。加速器的主要优势在于性能和能效,而非可靠性。鉴于芯片制程的可靠性问题日益凸显,我们亟需解决加速器的可靠性问题。此课题重点研究设计可扩展和有效的可靠异构架构。传统思路是单独地优化加速器可靠性。然而加速器的种类各不相同,对其进行单独优化将导致极大的工程量,使得系统无法快速地集成这些加速器。因此这种传统的优化方案是不可扩展的。申请人提出了一种新颖的可靠性设计方案,即非对称容错架构。该设计方案的目标在于找到一种“即插即用”的以加速器为主的系统架构,无须单独地优化其可靠性,而是在系统的层面上解决此问题。非对称容错架构以CPU为核心进行可靠性优化,当加速器发生错误时保障整个系统仍然能够可靠地运转。申请书中论证了该架构的可行性,并详细地描述了其设计思想和其中的关键科学问题。
结项摘要
现在的计算平台已经进入异构时代,包含了传统的CPU架构和大量的专用加速器架构。然而,随着芯片工艺制程的进一步缩小,晶体管接近原子大小,容易受包括高能粒子、供电电压扰动等外部因素影响而产生瞬态错误,给异构计算系统带来了可靠性难题,而芯片架构的可靠性在如自动驾驶和航空航天等安全优先场景下尤为重要。由于专用加速器架构和CPU架构存在着很大的不同,且专用架构的种类繁多,沿用传统CPU架构的可靠性优化容易造成很大的性能开销。通过三年的研究,本项目建立了一套面向高可靠异构计算平台的非对称容错系统架构。具体而言,本项目的研究成果仅需要专用加速器架构具备检错功能,而运行在CPU上的运行时系统对专用加速器上所检测到的错误进行恢复,其中用到的关键技术包括内存隔离、任务级幂等特性、任务依赖关系动态追踪等。通过这一系列关键技术,本项目的研究成果在保证可靠性优化方案的普适性,即不同的加速器架构可以采用这种类似的方法的同时,也最大程度地简化了加速器的容错要求和减轻了系统的性能开销。最终,我们可以小于1%的性能代价,保证整体异构系统的可靠性。在非对称容错系统架构基础上,我们还研究了目前最为广泛的人工智能专用架构的异常检测问题、基于新型3D堆叠工艺的处理器架构容错问题以及协处理器的低功耗优化技术。基于上述研究,本项目设计了面向异构计算平台的低开销高可靠系统架构。
项目成果
期刊论文数量(3)
专著数量(0)
科研奖励数量(0)
会议论文数量(7)
专利数量(2)
Architectural Implications of Graph Neural Networks
图神经网络的架构含义
- DOI:10.1109/lca.2020.2988991
- 发表时间:2020-01
- 期刊:IEEE Computer Architecture Letters
- 影响因子:2.3
- 作者:Zhang Zhihui;Leng Jingwen;Ma Lingxiao;Miao Youshan;Li Chao;Guo Minyi
- 通讯作者:Guo Minyi
Predictive Guardbanding: Program-driven Timing Margin Reduction for GPUs
预测性保护带:程序驱动的 GPU 时序裕度减少
- DOI:10.1109/tcad.2020.2992684
- 发表时间:2020
- 期刊:IEEE Transactions on Computer-Aided Design of Integrated Circuits and Systems
- 影响因子:2.9
- 作者:Jingwen Leng;Alper Buyuktosunoglu;Ramon Bertran;Pradip Bose;Yazhou Zu;Vijay Janapa Reddi
- 通讯作者:Vijay Janapa Reddi
Voltage-Stacked Power Delivery Systems: Reliability, Efficiency, and Power Management
电压堆叠供电系统:可靠性、效率和电源管理
- DOI:10.1109/tcad.2020.2969607
- 发表时间:2020-12
- 期刊:IEEE Transactions on Computer-Aided Design of Integrated Circuits and Systems
- 影响因子:2.9
- 作者:A. Zou;J. Leng;X. He;Y. Zu;C. D. Gill;V. J. Reddi;X. Zhang
- 通讯作者:X. Zhang
数据更新时间:{{ journalArticles.updateTime }}
{{
item.title }}
{{ item.translation_title }}
- DOI:{{ item.doi || "--"}}
- 发表时间:{{ item.publish_year || "--" }}
- 期刊:{{ item.journal_name }}
- 影响因子:{{ item.factor || "--"}}
- 作者:{{ item.authors }}
- 通讯作者:{{ item.author }}
数据更新时间:{{ journalArticles.updateTime }}
{{ item.title }}
- 作者:{{ item.authors }}
数据更新时间:{{ monograph.updateTime }}
{{ item.title }}
- 作者:{{ item.authors }}
数据更新时间:{{ sciAawards.updateTime }}
{{ item.title }}
- 作者:{{ item.authors }}
数据更新时间:{{ conferencePapers.updateTime }}
{{ item.title }}
- 作者:{{ item.authors }}
数据更新时间:{{ patent.updateTime }}
其他文献
其他文献
{{
item.title }}
{{ item.translation_title }}
- DOI:{{ item.doi || "--" }}
- 发表时间:{{ item.publish_year || "--"}}
- 期刊:{{ item.journal_name }}
- 影响因子:{{ item.factor || "--" }}
- 作者:{{ item.authors }}
- 通讯作者:{{ item.author }}
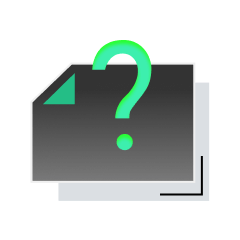
内容获取失败,请点击重试
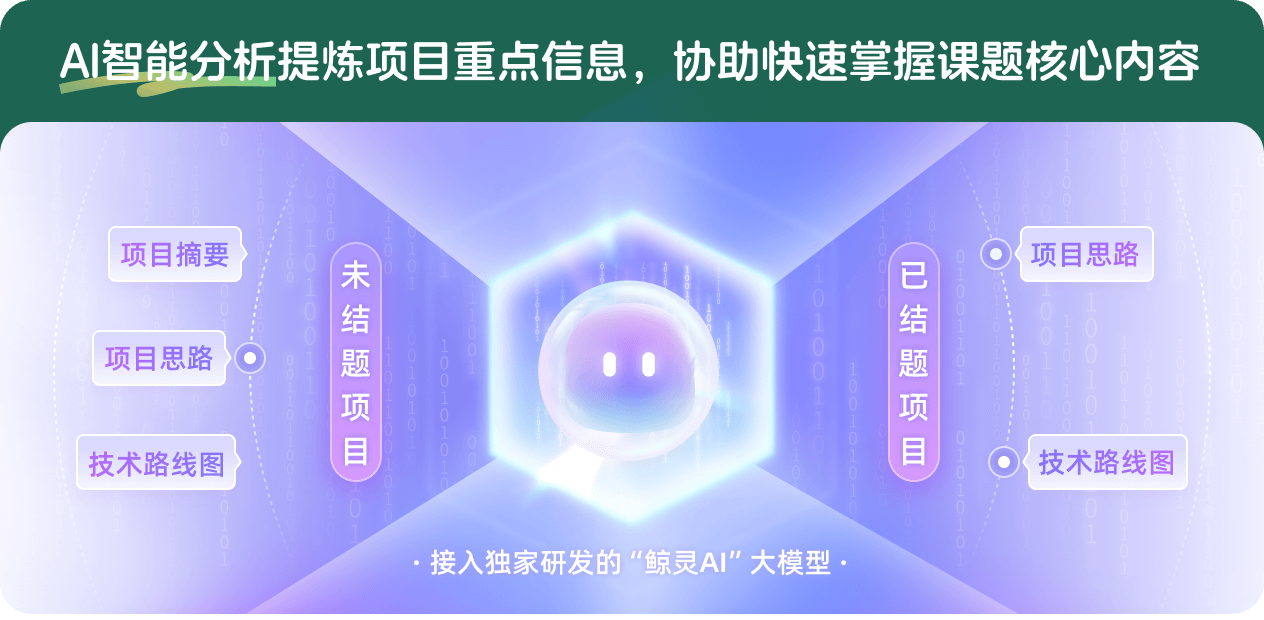
查看分析示例
此项目为已结题,我已根据课题信息分析并撰写以下内容,帮您拓宽课题思路:
AI项目摘要
AI项目思路
AI技术路线图
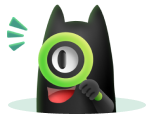
请为本次AI项目解读的内容对您的实用性打分
非常不实用
非常实用
1
2
3
4
5
6
7
8
9
10
您认为此功能如何分析更能满足您的需求,请填写您的反馈:
冷静文的其他基金
基于微架构模块的可组合式人工智能处理器设计和优化研究
- 批准号:62072297
- 批准年份:2020
- 资助金额:56 万元
- 项目类别:面上项目
相似国自然基金
{{ item.name }}
- 批准号:{{ item.ratify_no }}
- 批准年份:{{ item.approval_year }}
- 资助金额:{{ item.support_num }}
- 项目类别:{{ item.project_type }}
相似海外基金
{{
item.name }}
{{ item.translate_name }}
- 批准号:{{ item.ratify_no }}
- 财政年份:{{ item.approval_year }}
- 资助金额:{{ item.support_num }}
- 项目类别:{{ item.project_type }}