面向缺失值和非均衡类别面板数据的财务危机预测多层次集成模型研究
项目介绍
AI项目解读
基本信息
- 批准号:71801187
- 项目类别:青年科学基金项目
- 资助金额:19.0万
- 负责人:
- 依托单位:
- 学科分类:G0104.预测与评价
- 结题年份:2021
- 批准年份:2018
- 项目状态:已结题
- 起止时间:2019-01-01 至2021-12-31
- 项目参与者:陈远高; 姜辉; 揭婉晨; 张捷; 虞佳; 朱磊;
- 关键词:
项目摘要
Financial distress prediction is an important content in the field of financial management and risk management. Because of the limitation of the study in terms of the type of the selected financial indictor sample data which leads to that models can’t solve practical problems well, and the demand of the constructed integration model and the improved classifier, the panel data with missing values and imbalanced classes is regard as the study subject, and every layer in the process of prediction is researched, including the data pretreatment, the construction of integration models, the improvement of base classifiers and so on, in order to construct the multi-layer integration model from a new perspective. The main contents include: to process samples with the panel data of missing values, the integration model is constructed by combining different methods which is used to process different types of missing values; To process samples with the panel data of imbalanced classes, the sliding time window and the weight sampling are used to improve Learn++ incremental learning model; Fuzzy C-Means (FCM) algorithm is improved by different kernel functions to offset its deficiencies. Then, the integration model is constructed by these improved algorithms to enhance the prediction accuracy; Based on above research, the multi-layer integration model is built which synthesizes the whole process of prediction. This new model can process the sample data of financial indicators with the panel data of missing values and imbalanced classes, is applied to solve problems in fact, and enhances the prediction accuracy of financial distress.
财务危机预测是财务管理和风险管理领域的重要研究内容。针对财务指标样本数据选取类型的研究不足导致模型无法良好的解决实际问题的现象,同时满足集成模型建模和分类器改进的需要,以缺失值和非均衡类别面板数据为研究视角,对预测过程的数据预处理、集成模型构建、基分类器改进等每个层次进行深入研究,构建一种全新视角下的多层次集成模型。主要内容包括:构造一种由不同类型缺失值的解决方法组建的集成模型,处理带有缺失值面板数据的样本;采用滑动时间窗口和权重采样法改进Learn++增量学习模型,处理具有非均衡类别面板数据的样本;采用多种核函数分别改进模糊C均值聚类算法用于弥补其不足之处,并构建一种由这些改进算法组建的集成模型,提高基分类器的预测准确率;在此基础之上,综合整个预测过程构建一种多层次集成模型,使其能够同时处理缺失值和非均衡类别面板数据的财务样本,使模型的应用范围更符合实际情况,提高财务危机预测的准确率。
结项摘要
财务危机的发生会给企业人员和利益相关者带来严重的经济损失。良好的财务危机预警模型能够有效监控企业运营情况,避免企业倒闭或被重组的悲剧发生。本项目以缺失值和非均衡类别面板数据为研究视角,对预测过程的数据预处理、集成模型构建、基分类器改进等每个层次进行深入研究,构建一种全新视角下的多层次集成模型。研究过程中总结出了很多重要的结果。本研究提出了一种基于改进Learn++和模糊c均值(FCM)的动态不平衡商业信用评价集成模型。为了处理动态的不平衡财务数据,通过使用滑动时间窗(STW)和权重采样(WS)对Learn++进行了改进,并称为Learn++.STW-WS。STW可以将具有相同概念的数据划分为相同的数据集,以解决动态数据中存在的概念漂移问题;WS可以重新分配不同类别样本的权重,解决不平衡的问题。为了满足Learn++.STW-WS对基分类器预测精度上的要求,通过多核(MK)改进FCM,并将其命名为MK-FCM。利用均值法将多个核函数集成构造MK,采用MK改进FCM中点间距离的计算方法。在此基础之上构造一种具有优化算法的集成模型。该集成模型主要结合了SMOTE和MK-FCM,并采用粒子群优化算法进行优化。针对构造MK-FCM时不同核函数权重寻找困难的不足之处,采用粒子群优化算法对MK-FCM的权重参数进行优化。但就粒子群优化自身的不足之处,又采用动态调节功能改进了粒子群优化算法。采用集成模型的思想处理缺失值和非均衡类别的财务数据,提出了一种集成了多采样、多核模糊自组织映射和局部准确度的分类不平衡信用风险模型。此外,本项目还研究了财务绩效对我国这样的发展中国家企业绿色行为的影响,并进行了实证分析。该项目从新的视角研究了企业财务危机,构造的模型也都经过实证验证,确实能够帮助企业提高预测的准确率。
项目成果
期刊论文数量(7)
专著数量(0)
科研奖励数量(0)
会议论文数量(0)
专利数量(0)
Dynamic imbalanced business credit evaluation based on Learn ++ with sliding time window and weight sampling and FCM with multiple kernels
基于滑动时间窗Learn、权重采样和多核FCM的动态不平衡企业信用评价
- DOI:--
- 发表时间:2020
- 期刊:Information Sciences
- 影响因子:8.1
- 作者:Lu Wang;Chong Wu
- 通讯作者:Chong Wu
A hybrid model with novel feature selection method and enhanced voting method for credit scoring
一种具有新颖特征选择方法和增强投票方法的信用评分混合模型
- DOI:10.3233/jifs-211828
- 发表时间:2021-08
- 期刊:Journal of Intelligent & Fuzzy Systems
- 影响因子:2
- 作者:Yao Jianrong;Wang Zhongyi;Wang Lu;Zhang Zhebin;Jiang Hui;Yan Surong
- 通讯作者:Yan Surong
Investigating the Complex Relationship between Financial Performance and Company’s Green Behavior: A Comparative Analysis
调查财务绩效与公司绿色行为之间的复杂关系:比较分析
- DOI:10.1155/2021/9979835
- 发表时间:2021-06
- 期刊:Discrete Dynamics in Nature and Society
- 影响因子:1.4
- 作者:Zhang Wenyao;Xu Ruzhi;Wang Lu
- 通讯作者:Wang Lu
Imbalanced credit risk evaluation based on multiple sampling, multiple kernel fuzzy self-organizing map and local accuracy ensemble
基于多重采样、多核模糊自组织图和局部精度集成的不平衡信用风险评估
- DOI:10.1016/j.asoc.2020.106262
- 发表时间:2020-06
- 期刊:Applied Soft Computing Journal
- 影响因子:--
- 作者:Lu Wang;Yuangao Chen;Hui Jiang;Jianrong Yao
- 通讯作者:Jianrong Yao
Imbalanced credit risk prediction based on SMOTE and multi-kernel FCM improved by particle swarm optimization
粒子群优化改进的基于SMOTE和多核FCM的不平衡信用风险预测
- DOI:10.1016/j.asoc.2021.108153
- 发表时间:2022-01-01
- 期刊:APPLIED SOFT COMPUTING
- 影响因子:8.7
- 作者:Wang, Lu
- 通讯作者:Wang, Lu
数据更新时间:{{ journalArticles.updateTime }}
{{
item.title }}
{{ item.translation_title }}
- DOI:{{ item.doi || "--"}}
- 发表时间:{{ item.publish_year || "--" }}
- 期刊:{{ item.journal_name }}
- 影响因子:{{ item.factor || "--"}}
- 作者:{{ item.authors }}
- 通讯作者:{{ item.author }}
数据更新时间:{{ journalArticles.updateTime }}
{{ item.title }}
- 作者:{{ item.authors }}
数据更新时间:{{ monograph.updateTime }}
{{ item.title }}
- 作者:{{ item.authors }}
数据更新时间:{{ sciAawards.updateTime }}
{{ item.title }}
- 作者:{{ item.authors }}
数据更新时间:{{ conferencePapers.updateTime }}
{{ item.title }}
- 作者:{{ item.authors }}
数据更新时间:{{ patent.updateTime }}
其他文献
基于网络药理学分析金英黄归汤治疗乳腺炎的作用机制
- DOI:10.13822/j.cnki.hxsj.2021008045
- 发表时间:2021
- 期刊:化学试剂
- 影响因子:--
- 作者:彭俊超;杨会;何绪华;蔡亚玲;廖加美;龙利;王鲁
- 通讯作者:王鲁
CD14、MD-2在LPS致乳腺上皮细胞炎症中的作用
- DOI:10.3969/j.issn.1000-484x.2020.06.001
- 发表时间:2020
- 期刊:中国免疫学杂志
- 影响因子:--
- 作者:王万林;高月;谢雪艳;李天珍;易琼;王鲁
- 通讯作者:王鲁
基于改进遗传算法的社区挖掘研究
- DOI:10.19678/j.issn.1000-3428.0049128
- 发表时间:2019
- 期刊:计算机工程
- 影响因子:--
- 作者:郭旭超;王鲁;郝霞;孙晓勇;孙博
- 通讯作者:孙博
单酰基甘油酯酶对MHCC97H肝癌细胞体内增殖的影响
- DOI:10.3760/cma.j.issn.1007-3418.2019.07.008
- 发表时间:2019
- 期刊:中华肝脏病杂志
- 影响因子:--
- 作者:朱卫平;贺西淦;赵一鸣;潘奇;张宁;周嘉敏;王龙蓉;王苗;刘泽阳;朱洪旭;王鲁
- 通讯作者:王鲁
解淀粉芽孢杆菌HM618对盐胁迫下小麦幼苗生长及生理特性的影响
- DOI:--
- 发表时间:2020
- 期刊:天津农业科学
- 影响因子:--
- 作者:王鲁;魏宏达;方可;徐秋曼
- 通讯作者:徐秋曼
其他文献
{{
item.title }}
{{ item.translation_title }}
- DOI:{{ item.doi || "--" }}
- 发表时间:{{ item.publish_year || "--"}}
- 期刊:{{ item.journal_name }}
- 影响因子:{{ item.factor || "--" }}
- 作者:{{ item.authors }}
- 通讯作者:{{ item.author }}
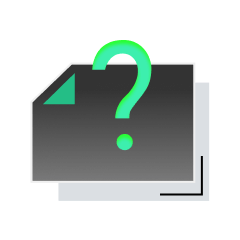
内容获取失败,请点击重试
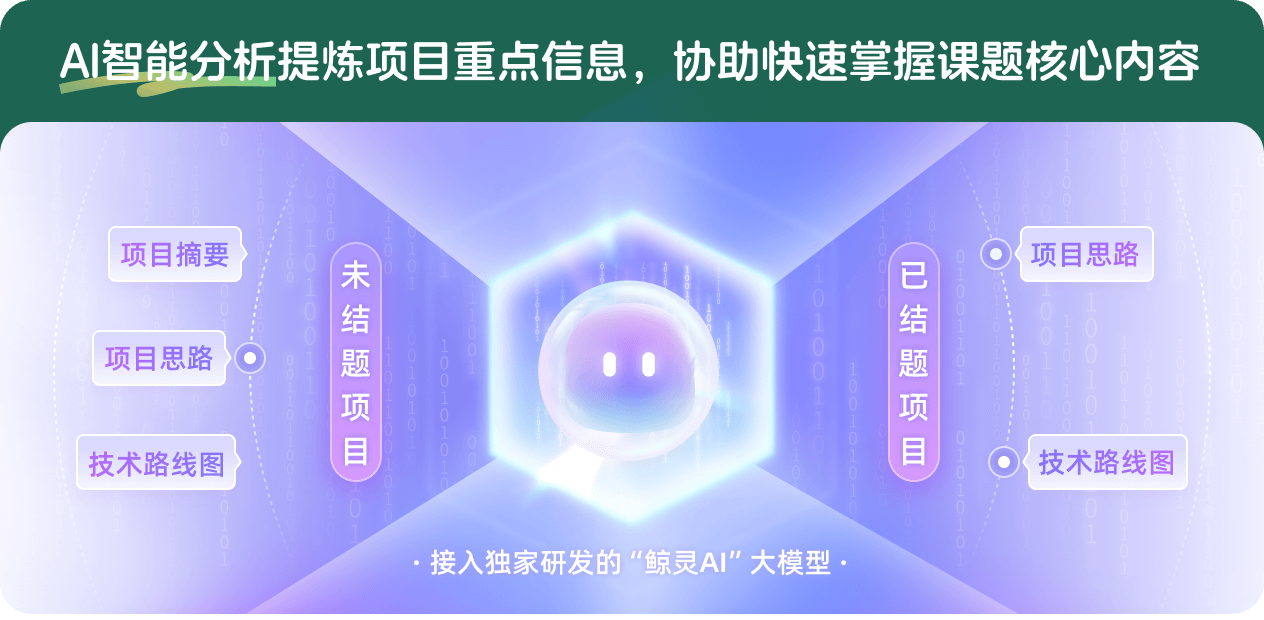
查看分析示例
此项目为已结题,我已根据课题信息分析并撰写以下内容,帮您拓宽课题思路:
AI项目摘要
AI项目思路
AI技术路线图
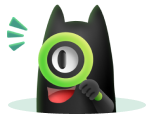
请为本次AI项目解读的内容对您的实用性打分
非常不实用
非常实用
1
2
3
4
5
6
7
8
9
10
您认为此功能如何分析更能满足您的需求,请填写您的反馈:
相似国自然基金
{{ item.name }}
- 批准号:{{ item.ratify_no }}
- 批准年份:{{ item.approval_year }}
- 资助金额:{{ item.support_num }}
- 项目类别:{{ item.project_type }}
相似海外基金
{{
item.name }}
{{ item.translate_name }}
- 批准号:{{ item.ratify_no }}
- 财政年份:{{ item.approval_year }}
- 资助金额:{{ item.support_num }}
- 项目类别:{{ item.project_type }}