解脂耶氏酵母还原性丁二酸合成途径的构建及代谢调控研究
项目介绍
AI项目解读
基本信息
- 批准号:
- 项目类别:青年科学基金项目
- 资助金额:30万
- 负责人:
- 依托单位:
- 学科分类:
- 结题年份:
- 批准年份:2022
- 项目状态:未结题
- 起止时间:2022至
- 项目参与者:崔志勇;
- 关键词:
项目摘要
结项摘要
项目成果
期刊论文列表
专著列表
科研奖励列表
会议论文列表
专利列表
数据更新时间:{{ journalArticles.updateTime }}
{{
item.title }}
{{ item.translation_title }}
- DOI:{{ item.doi || "--"}}
- 发表时间:{{ item.publish_year || "--" }}
- 期刊:{{ item.journal_name }}
- 影响因子:{{ item.factor || "--"}}
- 作者:{{ item.authors }}
- 通讯作者:{{ item.author }}
数据更新时间:{{ journalArticles.updateTime }}
{{ item.title }}
- 作者:{{ item.authors }}
数据更新时间:{{ monograph.updateTime }}
{{ item.title }}
- 作者:{{ item.authors }}
数据更新时间:{{ sciAawards.updateTime }}
{{ item.title }}
- 作者:{{ item.authors }}
数据更新时间:{{ conferencePapers.updateTime }}
{{ item.title }}
- 作者:{{ item.authors }}
数据更新时间:{{ patent.updateTime }}
其他文献
1992—2009年格拉丹东冰川及冰前湖面积变化的遥感研究
- DOI:--
- 发表时间:2014
- 期刊:中南大学学报(自然科学版)
- 影响因子:--
- 作者:周文明;李志伟;李佳;崔志勇;汪长城
- 通讯作者:汪长城
一种风力机虚拟惯量控制 与传统发电机调速控制的协调方法
- DOI:--
- 发表时间:2015
- 期刊:电网技术
- 影响因子:--
- 作者:王刚;侍乔明;崔志勇;付立军;吴优;徐力
- 通讯作者:徐力
1970-2000年青藏高原内流区冰川储量变化的初探
- DOI:--
- 发表时间:2014
- 期刊:地球物理学报
- 影响因子:--
- 作者:崔志勇;李志伟;李佳;汪长城;周文明
- 通讯作者:周文明
不同交通量下沥青面层整体动稳定度控制标准确定方法探讨
- DOI:--
- 发表时间:2016
- 期刊:公路交通科技
- 影响因子:--
- 作者:杨涛;李植淮;崔志勇;关宏信;单楠
- 通讯作者:单楠
车联网环境下的隐私安全度量方法
- DOI:10.11908/j.issn.0253-374x.22388
- 发表时间:2022
- 期刊:同济大学学报. 自然科学版
- 影响因子:--
- 作者:徐小雅;于海洋;崔志勇;王颖会;王朋成
- 通讯作者:王朋成
其他文献
{{
item.title }}
{{ item.translation_title }}
- DOI:{{ item.doi || "--" }}
- 发表时间:{{ item.publish_year || "--"}}
- 期刊:{{ item.journal_name }}
- 影响因子:{{ item.factor || "--" }}
- 作者:{{ item.authors }}
- 通讯作者:{{ item.author }}
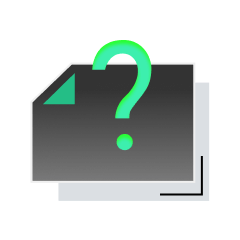
内容获取失败,请点击重试
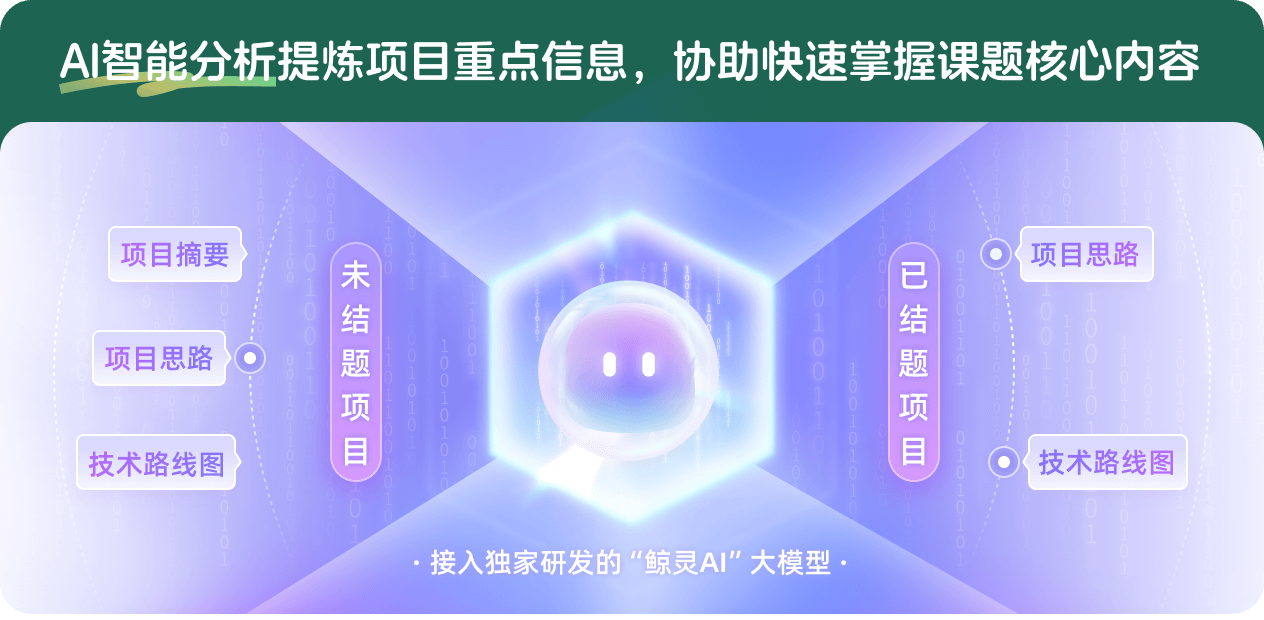
查看分析示例
此项目为未结题,我已根据课题信息分析并撰写以下内容,帮您拓宽课题思路:
AI项目摘要
AI项目思路
AI技术路线图
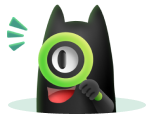
请为本次AI项目解读的内容对您的实用性打分
非常不实用
非常实用
1
2
3
4
5
6
7
8
9
10
您认为此功能如何分析更能满足您的需求,请填写您的反馈:
相似国自然基金
{{ item.name }}
- 批准号:{{ item.ratify_no }}
- 批准年份:{{ item.approval_year }}
- 资助金额:{{ item.support_num }}
- 项目类别:{{ item.project_type }}
相似海外基金
{{
item.name }}
{{ item.translate_name }}
- 批准号:{{ item.ratify_no }}
- 财政年份:{{ item.approval_year }}
- 资助金额:{{ item.support_num }}
- 项目类别:{{ item.project_type }}