高效鲁棒的行人重识别研究
项目介绍
AI项目解读
基本信息
- 批准号:61872329
- 项目类别:面上项目
- 资助金额:64.0万
- 负责人:
- 依托单位:
- 学科分类:F0210.计算机图像视频处理与多媒体技术
- 结题年份:2022
- 批准年份:2018
- 项目状态:已结题
- 起止时间:2019-01-01 至2022-12-31
- 项目参与者:李亚; 郭聪; 杨继伟; 何岸峰; 徐开胜; 曾令华; 王章成; 万超群; 崔燕平;
- 关键词:
项目摘要
With the development of video capturing and data storage techniques, video surveillance plays an increasingly important role in social public security. Person re-identification, one of the key techniques in video surveillance, has received more and more attentions. Various methods have been proposed and they mainly focus on extracting effective features for person re-identification via deep-learning-based models. Although they have made some progress, the identification accuracy and speed still cannot meet the practical requirements. In order to build a person re-identification system which can be applied in real-world video surveillance, on one aspect we propose to learn robust features and to design effective model training strategy, in order to boost the accuracy of person re-identification. On the other hand, in order to apply the pedestrian re-identification system to the ever-changing real environment, we propose to use the domain adaption method to enhance the generalization ability of the person re-identification model, so that the trained model can adapt to different monitoring environments. At the same time, we design an efficient end-to-end deep learning network low-bit quantization method, which significantly reduces the storage overhead and computational burden of pedestrian re-identification system, making the system can be effectively applied to video surveillance. The aim of this project is to make a breakthrough in the theory, methods, and techniques of person re-identification, and we believe it will advance the development of both theoretical research and techniques in the area of person re-identification.
随着视频采集及数据存储技术的发展,视频监控在社会公共安全中的作用越来越重要。行人重识别作为视频监控中的关键技术受到越来越多的关注。目前的研究主要集中在用深度学习模型提取有效的行人重识别特征。虽然取得了一定的研究进展,然而在准确度和速度上仍然达不到实用的要求。为了实现面向现实应用的行人重识别系统,本课题一方面学习鲁棒的特征并设计高效的模型训练方法,从而提升行人重识别的准确度。另一方面,为了能将行人重识别系统应用到千变万化的实际环境中,本课题提出使用域自适应方法提升模型的泛化性能,使得训练的模型能够适应不同的监控环境。同时,本课题设计了高效的端到端深度网络低比特量化方法,大幅降低行人重识别系统的存储开销和计算量,使得该系统可以高效地应用到实际视频监控中。本课题预期在行人重识别的理论、方法和技术上有较大突破,推动行人重识别的理论基础和技术研究。
结项摘要
为了实现面向现实应用的行人重识别系统,项目组主要从鲁棒特征学习、域泛化学习和模型加速三个方面展开研究,提升行人重识别系统的识别准确性、域泛化性和模型识别效率。项目组经过四年研究,在这三个方面取得了一系列创新研究成果。在特征学习方面,项目组提出了从多粒度区域提取多粒度行人重识别特征的方法和基于注意机制的行人重识别局部特征学习方法,提高了行人重识别特征的区分性,从而行人重识别的准确率得到提升。在域泛化学习方面,项目组提出了基于因果推断的域和类别解耦的泛化行人重识别和基于元卷积网络的单域泛化方法,使得在单个或多个域上训练的行人重识别模型可以很好地适应未知域,提升了行人重识别模型在不同环境下的适应性。在模型加速方面,项目组提出了可微分端到端优化的深度网络量化和基于继承与探索机制的深度模型蒸馏方法,大幅减少了深度模型的参数量和计算量,提升了行人重识别模型的效率。项目执行期间项目组发表学术论文27篇,其中IEEE和ACM汇刊6篇,CCF A类会议19篇;申请发明专利2项。该项目培养博士毕业生7人,硕士毕业生3人。项目组圆满完成了项目计划书制定的研究计划,达到了预期的研究目标。
项目成果
期刊论文数量(6)
专著数量(0)
科研奖励数量(0)
会议论文数量(21)
专利数量(2)
Learning multi-granularity features from multi-granularity regions for person re-identification
从多粒度区域学习多粒度特征以进行人员重新识别
- DOI:10.1016/j.neucom.2020.12.016
- 发表时间:2021
- 期刊:Neurocomputing
- 影响因子:6
- 作者:Kaiwen Yang;Jiwei Yang;Xinmei Tian
- 通讯作者:Xinmei Tian
Domain-Class Correlation Decomposition for Generalizable Person Re-Identification
用于泛化行人重识别的领域类相关分解
- DOI:10.1109/tmm.2022.3160057
- 发表时间:--
- 期刊:IEEE Transactions on Multimedia
- 影响因子:7.3
- 作者:Kaiwen Yang;Xinmei Tian
- 通讯作者:Xinmei Tian
CRAR: Accelerating Stereo Matching with Cascaded Residual Regression and Adaptive Refinement
CRAR:通过级联残差回归和自适应细化加速立体匹配
- DOI:10.1145/3488719
- 发表时间:2022
- 期刊:ACM Trans. Multim. Comput. Commun. Appl.
- 影响因子:--
- 作者:Linghua Zeng;Xinmei Tian
- 通讯作者:Xinmei Tian
Principal Component Adversarial Example
主成分对抗示例
- DOI:10.1109/tip.2020.2975918
- 发表时间:2020
- 期刊:IEEE Transactions on Image Processing (TIP), SCI-IF 10.856
- 影响因子:--
- 作者:Yonggang Zhang;Xinmei Tian;Ya Li;Xinchao Wang;Dacheng Tao
- 通讯作者:Dacheng Tao
Concentrated Local Part Discovery With Fine-Grained Part Representation for Person Re-Identification
通过细粒度部件表示进行集中局部部件发现,以实现人员重新识别
- DOI:10.1109/tmm.2019.2946486
- 发表时间:2020
- 期刊:IEEE Transactions on Multimedia
- 影响因子:7.3
- 作者:Chaoqun Wan;Yue Wu;Xinmei Tian;Jianqiang Huang;Xian-Sheng Hua
- 通讯作者:Xian-Sheng Hua
数据更新时间:{{ journalArticles.updateTime }}
{{
item.title }}
{{ item.translation_title }}
- DOI:{{ item.doi || "--"}}
- 发表时间:{{ item.publish_year || "--" }}
- 期刊:{{ item.journal_name }}
- 影响因子:{{ item.factor || "--"}}
- 作者:{{ item.authors }}
- 通讯作者:{{ item.author }}
数据更新时间:{{ journalArticles.updateTime }}
{{ item.title }}
- 作者:{{ item.authors }}
数据更新时间:{{ monograph.updateTime }}
{{ item.title }}
- 作者:{{ item.authors }}
数据更新时间:{{ sciAawards.updateTime }}
{{ item.title }}
- 作者:{{ item.authors }}
数据更新时间:{{ conferencePapers.updateTime }}
{{ item.title }}
- 作者:{{ item.authors }}
数据更新时间:{{ patent.updateTime }}
其他文献
其他文献
{{
item.title }}
{{ item.translation_title }}
- DOI:{{ item.doi || "--" }}
- 发表时间:{{ item.publish_year || "--"}}
- 期刊:{{ item.journal_name }}
- 影响因子:{{ item.factor || "--" }}
- 作者:{{ item.authors }}
- 通讯作者:{{ item.author }}
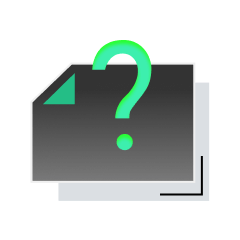
内容获取失败,请点击重试
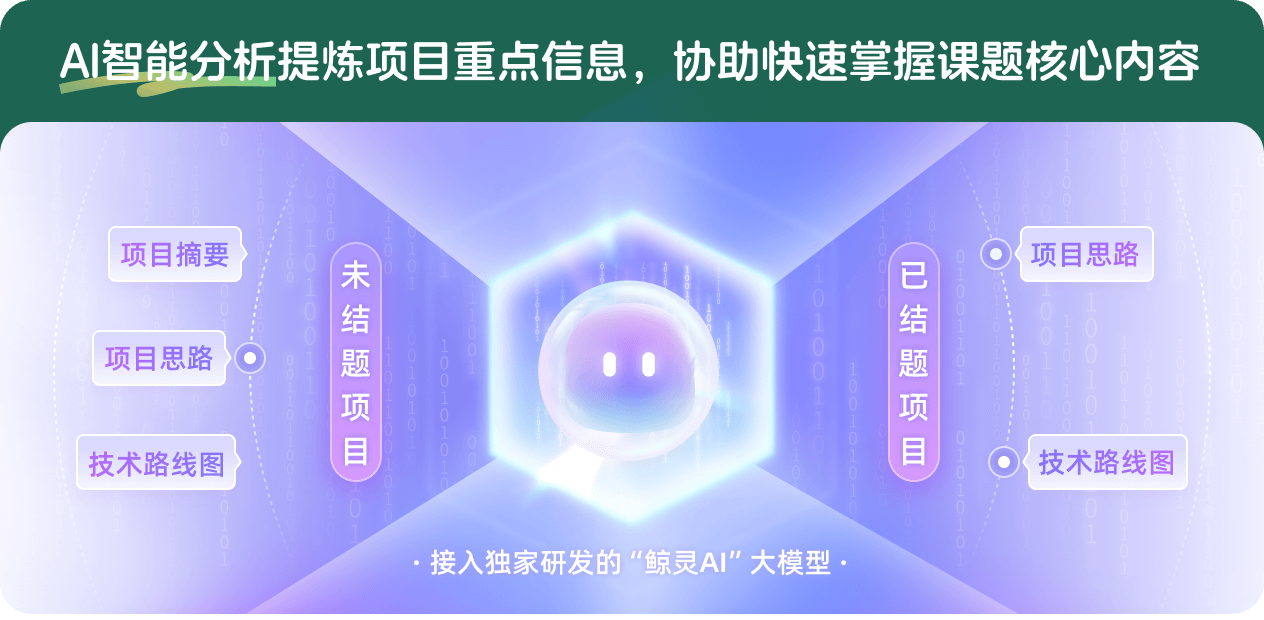
查看分析示例
此项目为已结题,我已根据课题信息分析并撰写以下内容,帮您拓宽课题思路:
AI项目摘要
AI项目思路
AI技术路线图
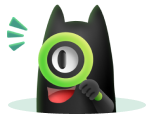
请为本次AI项目解读的内容对您的实用性打分
非常不实用
非常实用
1
2
3
4
5
6
7
8
9
10
您认为此功能如何分析更能满足您的需求,请填写您的反馈:
田新梅的其他基金
海量数据小训练样本集环境下的高效图像理解
- 批准号:61572451
- 批准年份:2015
- 资助金额:66.0 万元
- 项目类别:面上项目
遥感图象解译竞赛设计与实施
- 批准号:61350002
- 批准年份:2013
- 资助金额:30.0 万元
- 项目类别:专项基金项目
基于网络知识和人工知识的图像语义建模方法研究
- 批准号:61201413
- 批准年份:2012
- 资助金额:27.0 万元
- 项目类别:青年科学基金项目
相似国自然基金
{{ item.name }}
- 批准号:{{ item.ratify_no }}
- 批准年份:{{ item.approval_year }}
- 资助金额:{{ item.support_num }}
- 项目类别:{{ item.project_type }}
相似海外基金
{{
item.name }}
{{ item.translate_name }}
- 批准号:{{ item.ratify_no }}
- 财政年份:{{ item.approval_year }}
- 资助金额:{{ item.support_num }}
- 项目类别:{{ item.project_type }}